Each word can be represented by an index in a vocabulary:
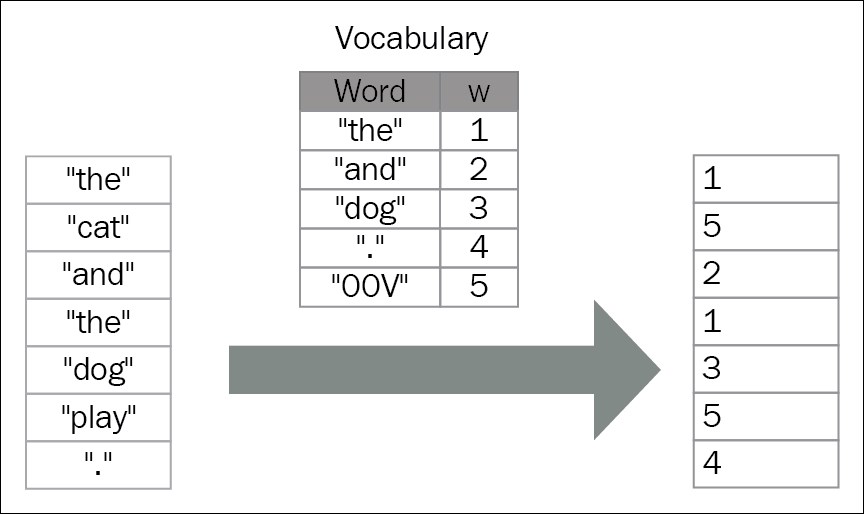
Encoding words is the process of representing each word as a vector. The simplest method of encoding words is called one-hot or 1-of-K vector representation. In this method, each word is represented as an vector with all 0s and one 1 at the index of that word in the sorted vocabulary. In this notation, |V| is the size of the vocabulary. Word vectors in this type of encoding for vocabulary {King, Queen, Man, Woman, Child} appear as in the following example of encoding for the word Queen:
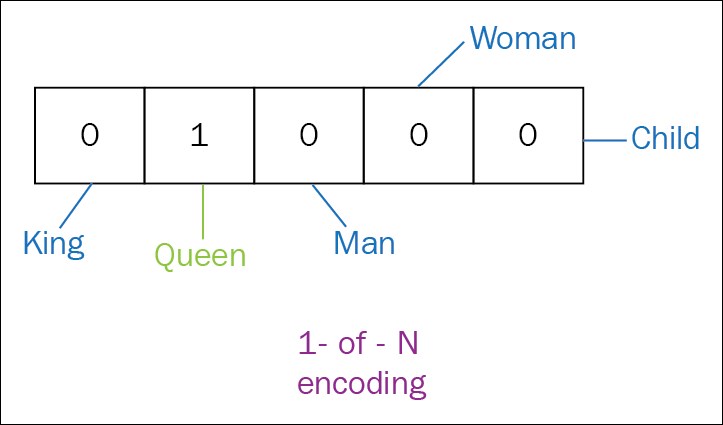
In the one-hot vector representation method, every word is equidistant from the other. However, it fails to preserve any relationship between them and leads to data sparsity. Using word embedding does overcome some of these drawbacks.
Word embedding is an approach to distributional semantics that represents words as vectors of real numbers. Such representation has useful clustering properties, since it groups together words that...