A major approach to solve games has been the Q-learning approach. In order to fully understand the approach, a basic example will illustrate a simplistic case where the number of states of the environment is limited to 6, state 0 is the entrance, state 5 is the exit. At each stage, some actions make it possible to jump to another state, as described in the following figure:
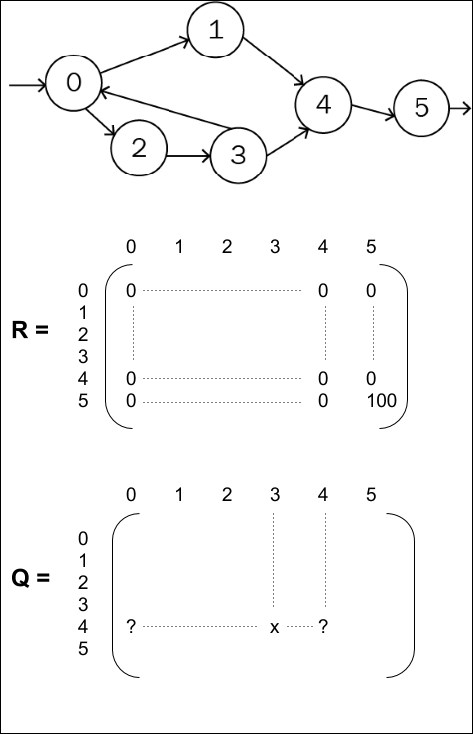
The reward is, let's say, 100, when the agent leaves state 4 to state 5. There isn't any other reward for other states since the goal of the game in this example is to find the exit. The reward is time-delayed and the agent has to scroll through multiple states from state 0 to state 4 to find the exit.
In this case, Q-learning consists of learning a matrix Q, representing the value of a state-action pair:
Each row in the Q-matrix corresponds to a state the agent would be in
Each column the target state from that state
the value representing how much choosing that action in that state will move us close to the exit...