Deep Q-network
While the number of possible actions is usually limited (number of keyboard keys or movements), the number of possible states can be dramatically huge, the search space can be enormous, for example, in the case of a robot equipped with cameras in a real-world environment or a realistic video game. It becomes natural to use a computer vision neural net, such as the ones we used for classification in Chapter 7, Classifying Images with Residual Networks, to represent the value of an action given an input image (the state), instead of a matrix:
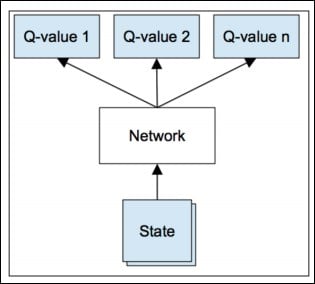
The Q-network is called a state-action value network and predicts action values given a state. To train the Q-network, one natural way of doing it is to have it fit the Bellman equation via gradient descent:
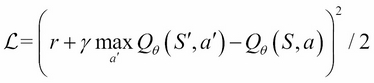
Note that, is evaluated and fixed, while the descent is computed for the derivatives in,
and that the value of each state can be estimated as the maximum of all state-action values.
After initializing the Q-network with random weights...