The story of how the ELK Stack becomes Elasticsearch, Logstash, and Kibana, is a pretty long story (https://www.elastic.co/about/history-of-elasticsearch). At Elastic{ON} 2015 in San Francisco, Elasticsearch Inc. was renamed Elastic and announced the next evolution of Elastic Stack. Elasticsearch will still play an important role, no matter what happens.
Elasticsearch architectural overview
Elastic Stack architecture
Elastic Stack is an end-to-end software stack for search and analysis solutions. It is designed to help users get data from any type of source in any format to allow for searching, analyzing, and visualizing data in real time. The full stack consists of the following:
- Beats master: A lightweight data conveyor that can send data directly to Elasticsearch or via Logstash
- APM server master: Used for measuring and monitoring the performance of applications
- Elasticsearch master: A highly scalable full-text search and analytics engine
- Elasticsearch Hadoop master: A two-way fast data mover between Apache Hadoop and Elasticsearch
- Kibana master: A primer on data exploration, visualization, and dashboarding
- Logstash master: A data-collection engine with real-time pipelining capabilities
Each individual product has its own purpose and features, as shown in the following diagram:

Elasticsearch architecture
Elasticsearch is a real-time distributed search and analytics engine with high availability. It is used for full-text search, structured search, analytics, or all three in combination. It is built on top of the Apache Lucene library. It is a schema-free, document-oriented data store. However, unless you fully understand your use case, the general recommendation is not to use it as the primary data store. One of the advantages is that the RESTful API uses JSON over HTTP, which allows you to integrate, manage, and query index data in a variety of ways.
An Elasticsearch cluster is a group of one or more Elasticsearch nodes that are connected together. Let's first outline how it is laid out, as shown in the following diagram:
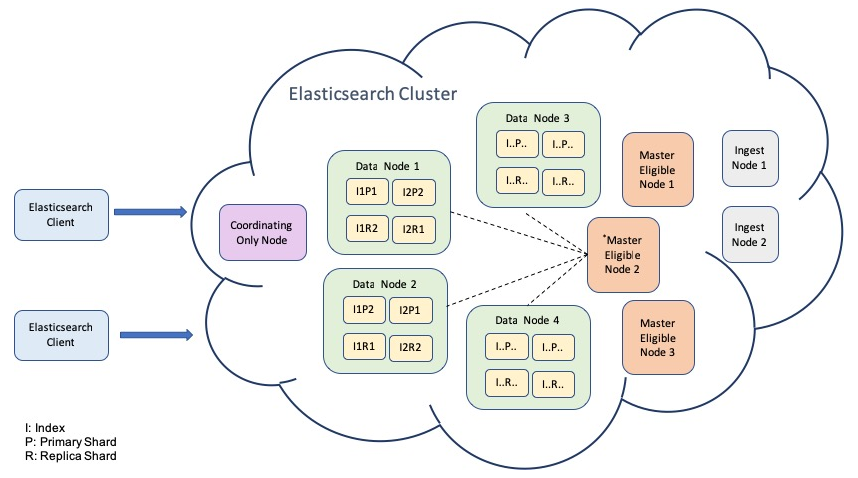
Although each node has its own purpose and responsibility, each node can forward client requests (coordination) to the appropriate nodes. The following are the nodes used in an Elasticsearch cluster:
- Master-eligible node: The master node's tasks are primarily used for lightweight cluster-wide operations, including creating or deleting an index, tracking the cluster nodes, and determining the location of the allocated shards. By default, the master-eligible role is enabled. A master-eligible node can be elected to become the master node (the node with the asterisk) by the master-election process. You can disable this type of role for a node by setting node.master to false in the elasticsearch.yml file.
- Data node: A data node contains data that contains indexed documents. It handles related operations such as CRUD, search, and aggregation. By default, the data node role is enabled, and you can disable such a role for a node by setting the node.data to false in the elasticsearch.yml file.
- Ingest node: Using an ingest nodes is a way to process a document in pipeline mode before indexing the document. By default, the ingest node role is enabled—you can disable such a role for a node by setting node.ingest to false in the elasticsearch.yml file.
- Coordinating-only node: If all three roles (master eligible, data, and ingest) are disabled, the node will only act as a coordination node that performs routing requests, handling the search reduction phase, and distributing works via bulk indexing.
When you launch an instance of Elasticsearch, you actually launch the Elasticsearch node. In our installation, we are running a single node of Elasticsearch, so we have a cluster with one node. Let's retrieve the information for all nodes from our installed server using the Elasticsearch cluster nodes info API, as shown in the following screenshot:
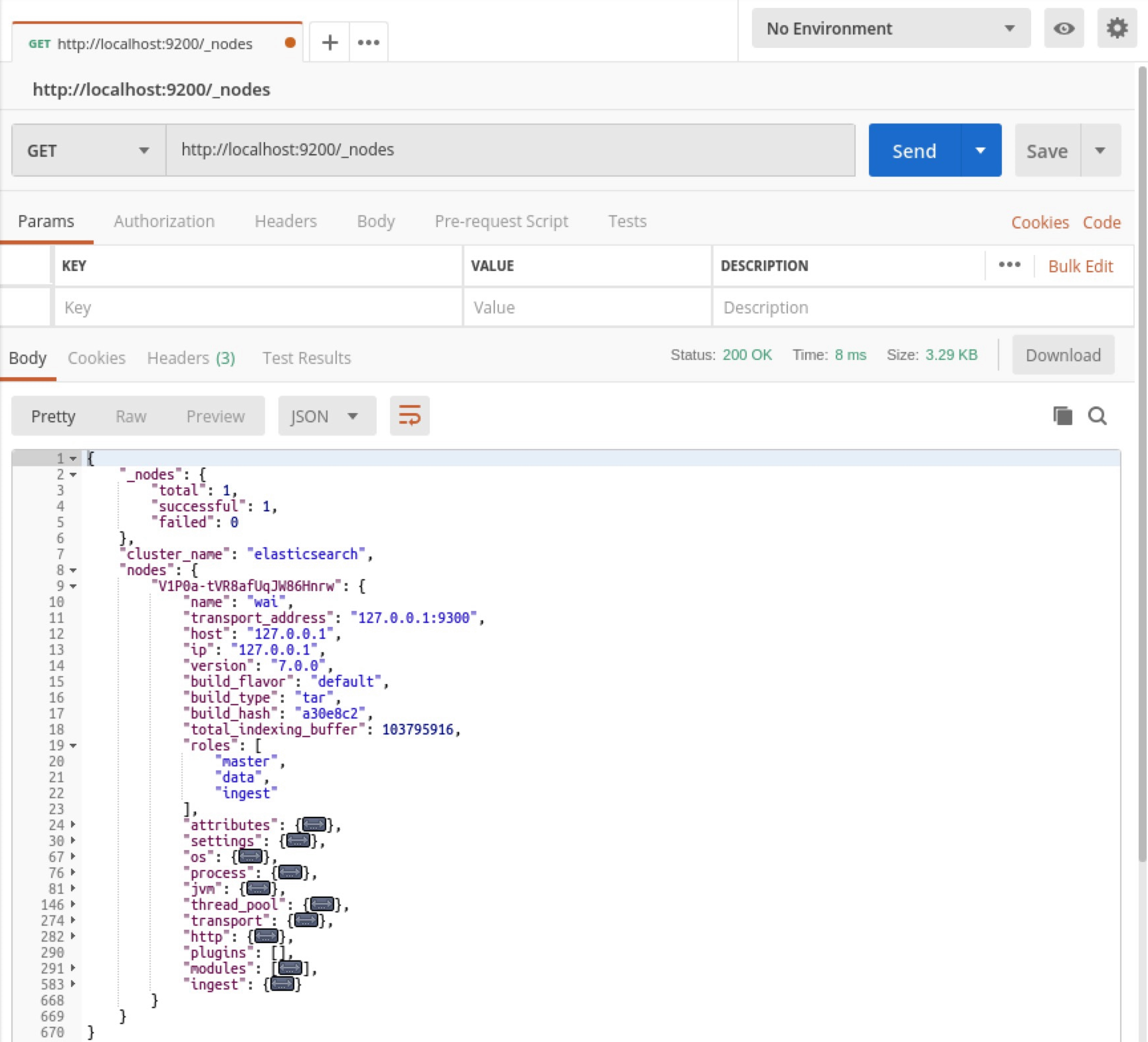
The cluster name is elasticsearch. The total number of nodes is 1. The node ID is V1P0a-tVR8afUqJW86Hnrw. The node name is wai. The wai node has three roles, which are master, data, and ingest. The Elasticsearch version running on the node is 7.0.0.
Between the Elasticsearch index and the Lucene index
The data in Elasticsearch is organized into indices. Each index is a logical namespace for organizing data. The document is a basic unit of data in Elasticsearch. An inverted index is created by tokenizing the terms in the document, creating a sorted list of all unique terms, and associating the document list with the location where the terms can be found. An index consists of one or more shards. A shard is a Lucene index that uses a data structure (inverted index) to store data. Each shard can have zero or more replicas. Elasticsearch ensures that the primary and the replica of the same shard will not collocate in the same node, as shown in the following screenshot, where Data Node 1 contains primary shard 1 of Index 1 (I1P1), primary shard 2 of Index 2 (I2P2), replica shard 2 of Index 1 (I1R2), and replica shard 1 of Index 2 (I2R1).
A Lucene index consists of one or more immutable index segments, and a segment is a functional inverted index. Segments are immutable, allowing Lucene to incrementally add new documents to the index without rebuilding efforts. To maintain the manageability of the number of segments, Elasticsearch merges the small segments together into one larger segment, commits the new merge segment to disk and eliminates the old smaller segments at the appropriate time. For each search request, all Lucene segments of a given shard of an Elasticsearch index will be searched. Let's examine the query process in a cluster, as shown in the following diagram:
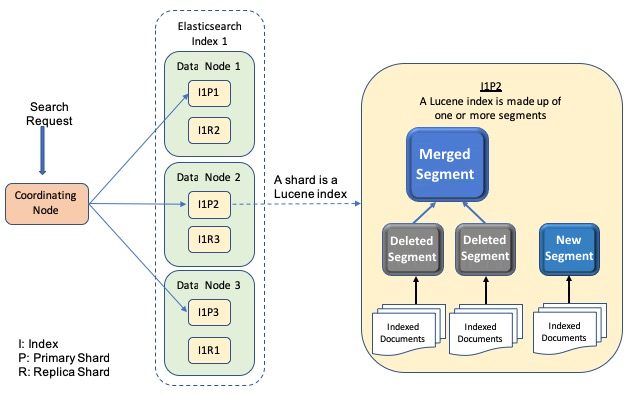
In the next section, let's drilled down to the key concepts.