Though RDD is getting replaced with DataFrame/DataSet-based APIs, there are still a lot of APIs that have not been migrated yet. In this recipe, we will look at how the concept of lineage works in RDD.
Externally, RDD is a distributed, immutable collection of objects. Internally, it consists of the following five parts:
- Set of partitions (
rdd.getPartitions
) - List of dependencies on parent RDDs (
rdd.dependencies
) - Function to compute a partition, given its parents
- Partitioner, which is optional (
rdd.partitioner
) - Preferred location of each partition, which is optional (
rdd.preferredLocations
)
The first three are needed for an RDD to be recomputed in case data is lost. When combined, it is called lineage. The last two parts are optimizations.
A set of partitions is how data is divided into nodes. In the case of HDFS, it means InputSplits
, which are mostly the same as the block (except when a record crosses block boundaries; in that case, it will be slightly bigger than a block).
Let's revisit our word count example to understand these five parts. This is how an RDD graph looks for wordCount at the dataset level view:
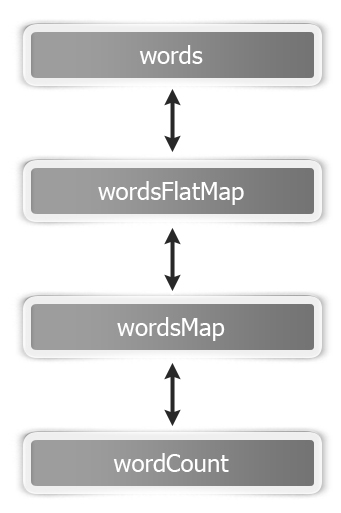
Basically, this is how the flow goes:
- Load the
words
folder as an RDD:
scala> val words = sc.textFile("hdfs://localhost:9000/user/hduser/words")
The following are the five parts of the words
RDD:
Part | Description |
Partitions | One partition per HDFS inputsplit/block ( |
Dependencies | None |
Compute function | To read the block |
Preferred location | The HDFS block's location |
Partitioner | None |
- Tokenize the words of the
words
RDD with each word on a separate line:
scala> val wordsFlatMap = words.flatMap(_.split("W+"))
The following are the five parts of the wordsFlatMap
RDD:
Part | Description |
Partitions | Same as the parent RDD, that is, |
Dependencies | Same as the parent RDD, that is, |
Compute function | To compute the parent and split each element, which flattens the results |
Preferred location | Ask parent RDD |
Partitioner | None |
- Transform each word in the
wordsFlatMap
RDD into the (word,1) tuple:
scala> val wordsMap = wordsFlatMap.map( w => (w,1))
The following are the five parts of the wordsMap
RDD:
Part | Description |
Partitions | Same as the parent RDD, that is, wordsFlatMap (org.apache.spark.rdd.HadoopPartition) |
Dependencies | Same as the parent RDD, that is, wordsFlatMap (org.apache.spark.OneToOneDependency) |
Compute function | To compute the parent and map it to PairRDD |
Preferred Location | Ask parent RDD |
Partitioner | None |
- Reduce all the values of a given key and sum them up:
scala> val wordCount = wordsMap.reduceByKey(_+_)
The following are the five parts of the wordCount
RDD:
Part | Description |
Partitions | One per reduce task ( |
Dependencies | Shuffle dependency on each parent ( |
Compute function | To perform additions on shuffled data |
Preferred location | None |
Partitioner | HashPartitioner ( |
This is how an RDD graph of wordcount
looks at the partition level view:
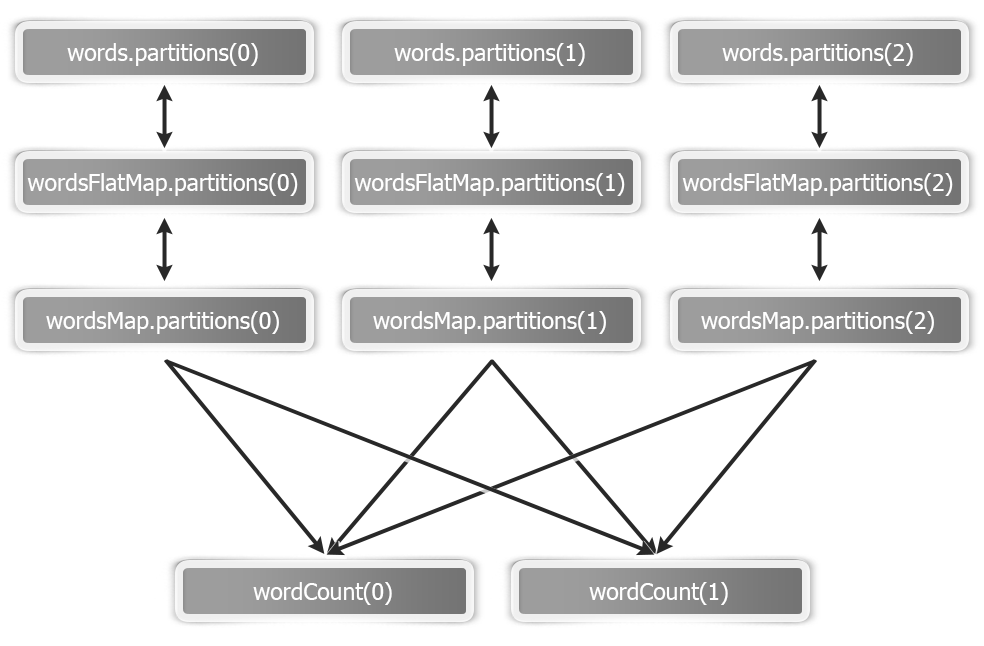