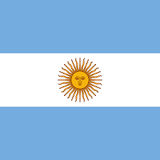
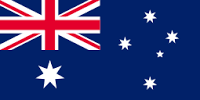
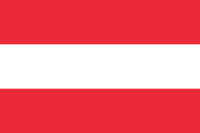
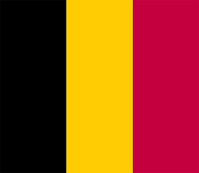
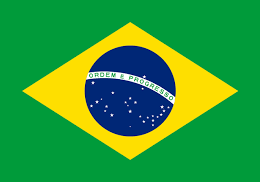
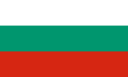
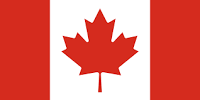
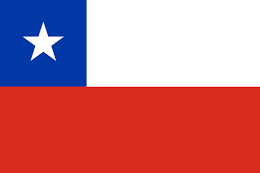
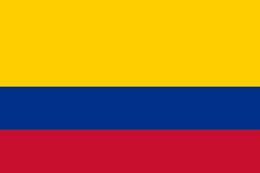
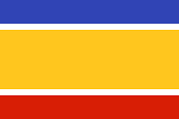
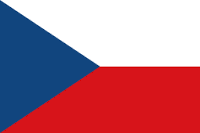
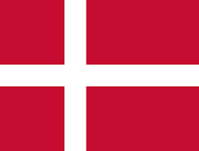
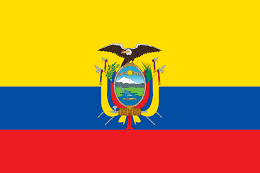
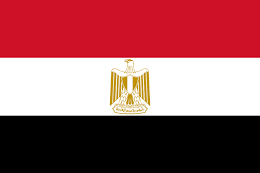
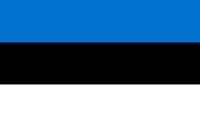
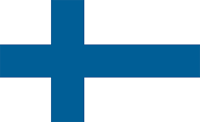
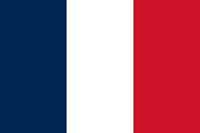
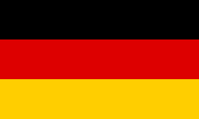
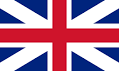
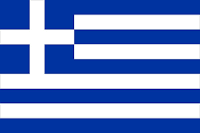
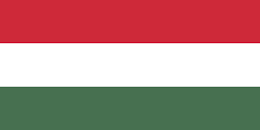
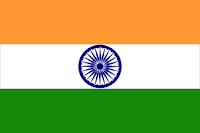
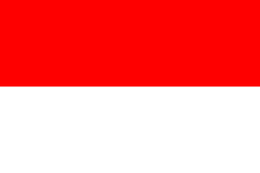
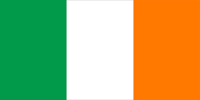
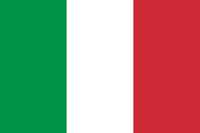
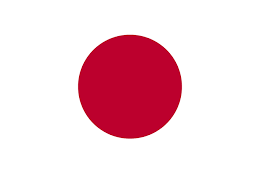
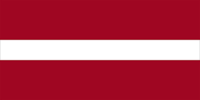
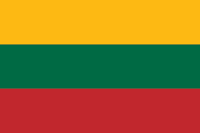
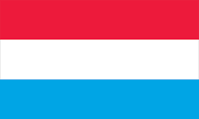
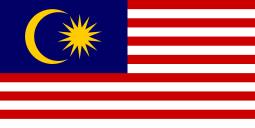
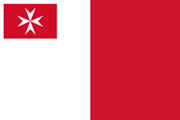
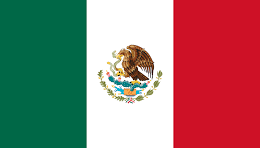
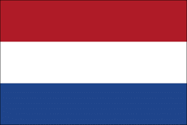
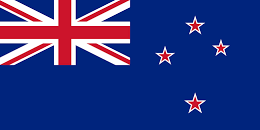
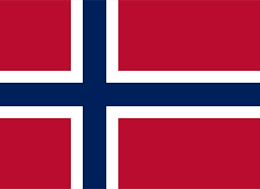
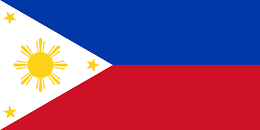
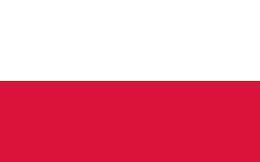
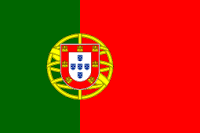
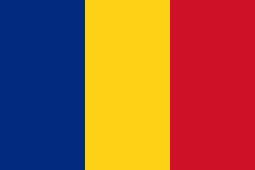
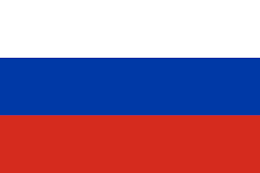

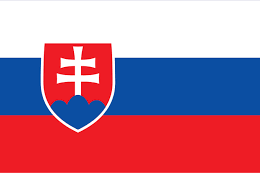
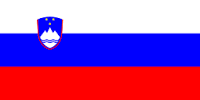
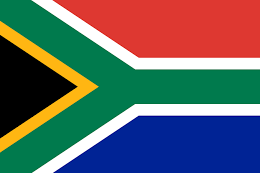
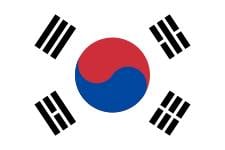
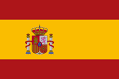
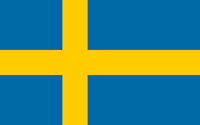
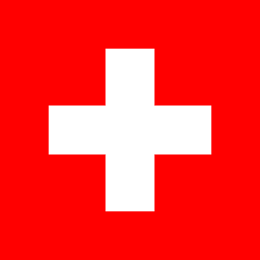
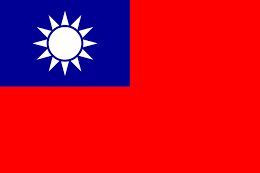
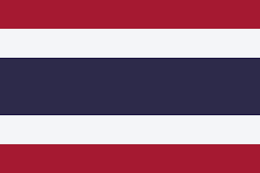
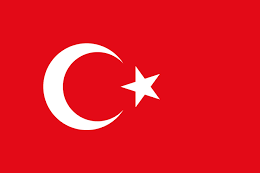
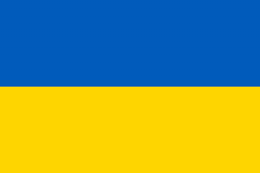
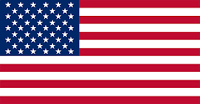
The following is Wikipedia's definition of recommender systems:
"Recommender systems are a subclass of information filtering system that seeks to predict the rating or preference that user would give to an item."
Recommender systems have gained immense popularity in recent years. Amazon uses them to recommend books, Netflix for movies, and Google News to recommend news stories. As the proof is in the pudding, here are some examples of the impact recommendations can have (source: Celma, Lamere, 2008):
As we saw in the previous chapters, features and feature selection play a major role in the efficacy of machine learning algorithms. Recommender engine algorithms discover these features, called latent features, automatically. In short, there are latent features responsible for a user to like one movie and...
Collaborative filtering is the most commonly used technique for recommender systems. It has an interesting property—it learns the features on its own. So, in the case of movie ratings, we do not need to provide actual human feedback on whether the movie is romantic or action.
As we saw, in the preceding section, movies have some latent features, such as genre, in the same way, users have some latent features, such as age, gender, and more. Collaborative filtering does not need them; it figures out latent features on its own.
We are going to use an algorithm called alternating least squares (ALS) in this example. This algorithm explains the association between a movie and a user based on a small number of latent features. It uses three training parameters: rank
, number of iterations
, and lambda
(explained later in the chapter). The best way to figure out the optimum values of these three parameters is to try different values and see which value...
Sometimes, the feedback available is not in the form of ratings but in the form of audio tracks played, movies watched, and so on. This data, at first glance, may not look as good as explicit ratings by users, but this is much more exhaustive.
We are going to use the million song data from http://www.kaggle.com/c/msdchallenge/data. You need to download three files:
kaggle_visible_evaluation_triplets
kaggle_users.txt
kaggle_songs.txt
We still need to do some more preprocessing. ALS in MLlib takes both user and product IDs as integers. The Kaggle_songs.txt
file has song IDs and a sequence number next to it. The Kaggle_users.txt
file does not have a sequence number. Our goal is to replace the userid
and songid
in the triplets
data with the corresponding integer sequence numbers. To do this, follow these steps:
$ spark-shell
import org.apache.spark...