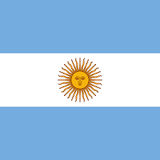
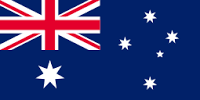
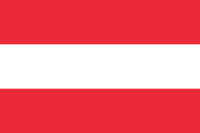
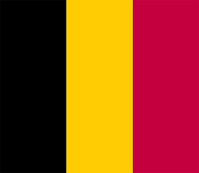
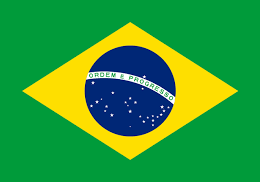
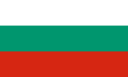
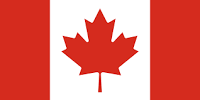
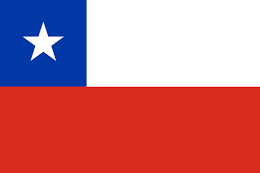
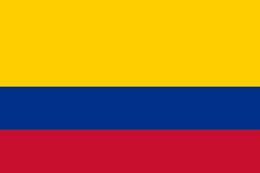
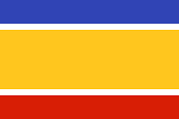
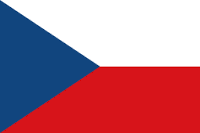
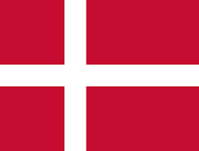
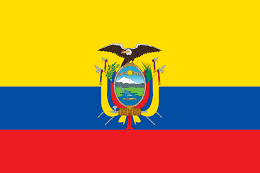
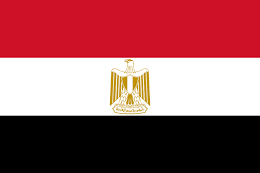
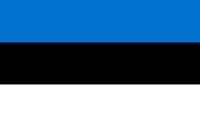
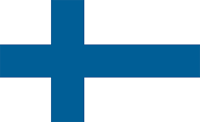
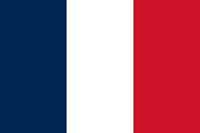
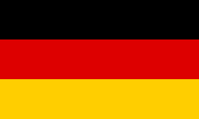
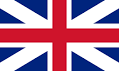
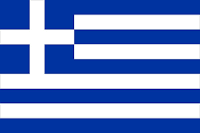
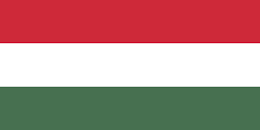
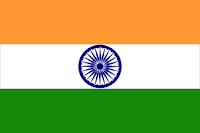
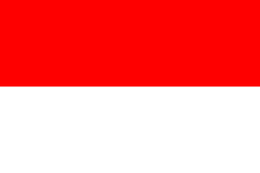
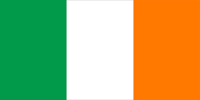
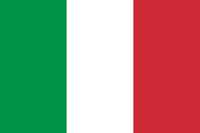
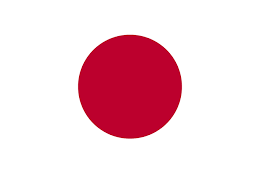
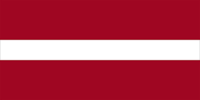
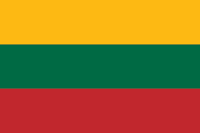
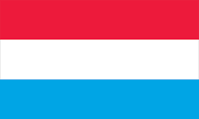
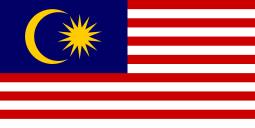
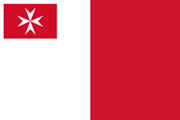
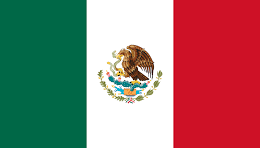
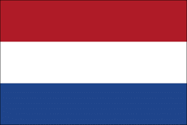
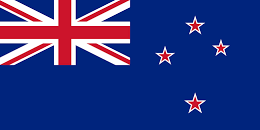
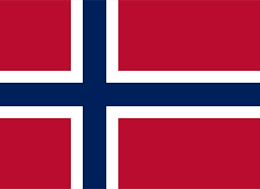
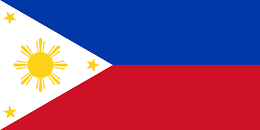
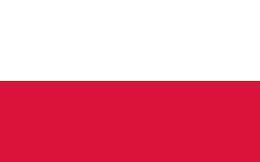
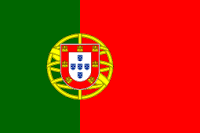
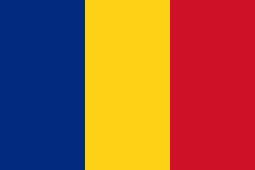
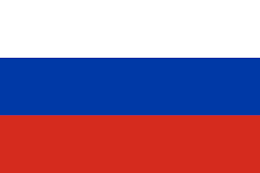

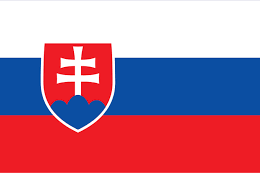
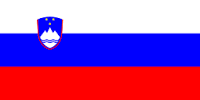
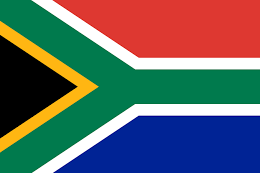
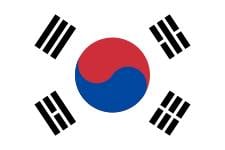
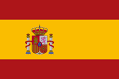
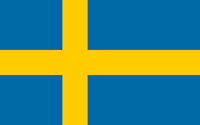
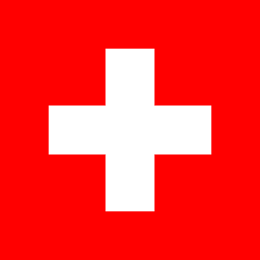
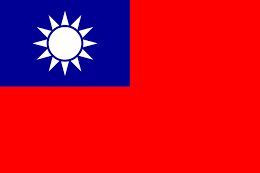
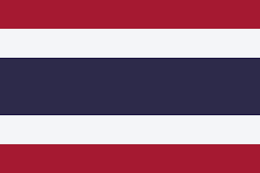
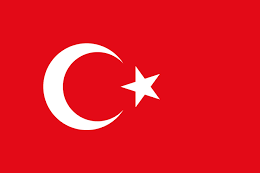
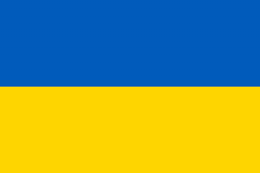
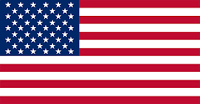
The following is Wikipedia's definition of supervised learning:
"Supervised learning is the machine learning task of inferring a function from labeled training data."
There are two types of supervised learning algorithms:
We are going to cover regression in this chapter and classification in the next.
We will use the recently sold house data of the City of Saratoga, CA, as an example to illustrate the steps of supervised learning in the case of regression:
Linear regression is the approach to model the value of a response or outcome variable y, based on one or more predictor variables or features, represented by x.
Let's use some housing data to predict the price of a house based on its size. The following are the sizes and prices of houses in the City of Saratoga, CA, in early 2014:
House size (sq. ft.) | Price |
---|---|
2100 | $ 1,620,000 |
2300 | $ 1,690,000 |
2046 | $ 1,400,000 |
4314 | $ 2,000,000 |
1244 | $ 1,060,000 |
4608 | $ 3,830,000 |
2173 | $ 1,230,000 |
2750 | $ 2,400,000 |
4010 | $ 3,380,000 |
1959 | $ 1,480,000 |
Here's a graphical representation of the same:
$ spark-shell
scala> import org.apache.spark.ml.linalg.Vectors
scala> import org.apache.spark.ml.regression.LinearRegression
scala> val points = spark.createDataFrame(Seq(
(1620000,Vectors.dense(2100)),
(1690000,Vectors.dense(2300)),
(1400000,Vectors.dense(2046)),
...
The cost function or loss function is a very important function in machine learning algorithms. Most algorithms have some form of cost function, and the goal is to minimize this. Parameters, which affect cost functions, such as stepSize
, are called hyperparameters; they need to be set by hand. Therefore, understanding the whole concept of the cost function is very important.
In this recipe, we are going to analyze the cost function in linear regression. Linear regression is a simple algorithm to understand, and it will help you understand the role of cost functions for even complex algorithms.
Let's go back to linear regression. The goal is to find the best-fitting line so that the mean square of the error would be minimum. Here, we are referring to an error as the difference between the value as per the best-fitting line and the actual value of the response variable of the training dataset.
For a simple case of a single predicate variable, the best-fitting line...
Lasso is a shrinkage and selection method for linear regression. It minimizes the usual sum of squared errors with an upper bound on the sum of the absolute values of the coefficients. It is based on the original lasso paper found at http://statweb.stanford.edu/~tibs/lasso/lasso.pdf.
The least square method we used in the last recipe is also called ordinary least squares (OLS). OLS has two challenges:
An alternate way to improve prediction quality is to do ridge regression. In lasso, a lot of the features get their coefficients set to zero and, therefore, eliminated from the equation. In ridge, predictors or features are penalized, but never set to zero. How to do it...
$ spark-shell
scala> import org.apache.spark.ml.linalg.Vectors
scala> import org.apache.spark.ml.regression.LinearRegression
scala> val points = spark.createDataFrame(Seq(
(1d,Vectors.dense(5,3,1,2,1,3,2,2,1)),
(2d,Vectors.dense(9,8,8,9,7,9,8,7,9))
)).toDF("label","features")
scala> val lr = new LinearRegression().setMaxIter(10).setRegParam(.3).setFitIntercept(false).setElasticNetParam(0.0)
scala> val model = lr.fit(points)