After understanding both AdaBoost and gradient boost, readers may be curious to see the differences in detail. Here, we are presenting exactly that to quench your thirst!
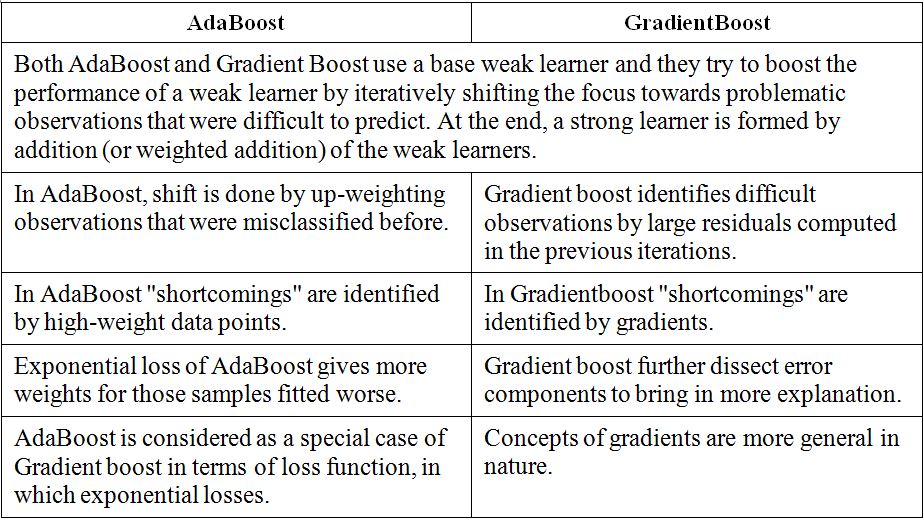
The gradient boosting classifier from the scikit-learn package has been used for computation here:
# Gradientboost Classifier >>> from sklearn.ensemble import GradientBoostingClassifier
Parameters used in the gradient boosting algorithms are as follows. Deviance has been used for loss, as the problem we are trying to solve is 0/1 binary classification. The learning rate has been chosen as 0.05, number of trees to build is 5000 trees, minimum sample per leaf/terminal node is 1, and minimum samples needed in a bucket for qualification for splitting is 2:
>>> gbc_fit = GradientBoostingClassifier (loss='deviance', learning_rate=0.05, n_estimators=5000, min_samples_split=2, min_samples_leaf=1, max_depth=1, random_state=42 ) >>> gbc_fit.fit(x_train...