Now that we are comfortable with creating multiclass classifiers using logistic regression and are getting reasonable performance with these models, we will turn our attention to another type of classifier: the K-nearest neighbors (K-NN) clustering method of classification. This is a handy method, as it can be used in both supervised classification problems as well as in unsupervised problems.
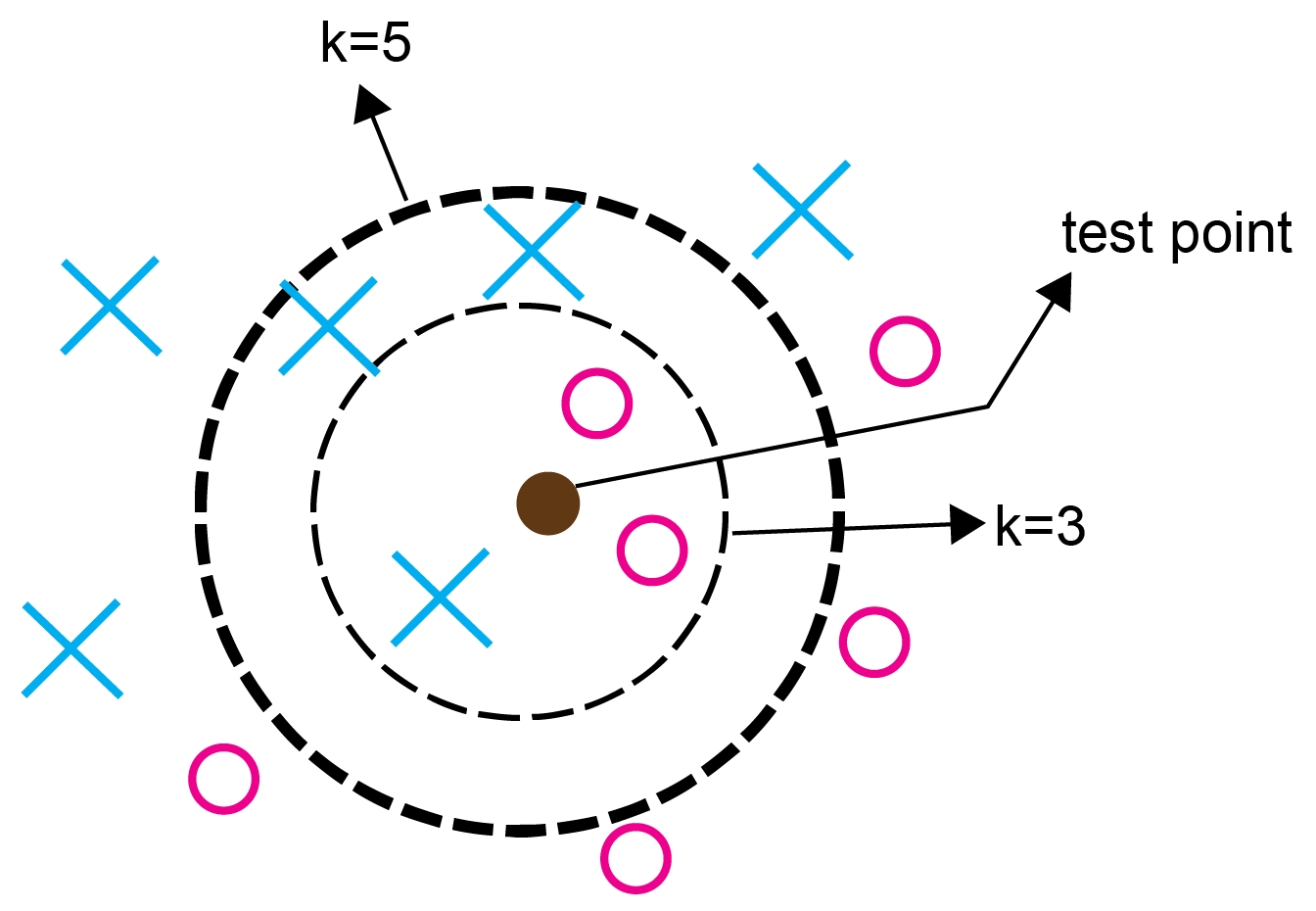
Figure 4.32: Visual representation of K-NN
The solid circle approximately in the center is the test point requiring classification, while the inner circle shows the classification process where K=3 and the outer circle where K=5.
K-NN is one of the simplest "learning" algorithms available for data classification. The use of learning in quotation marks is explicit, as K-NN doesn't really learn from the data and encode these learnings in parameters or weights like other methods, such as logistic regression. K-NN uses instance-based or lazy learning in that it simply...