Generally, a neural network needs labeled examples to learn effectively. Unsupervised learning approaches to learn from unlabeled data have not worked very well. A generative adversarial network, or simply a GAN, is part of an unsupervised learning approach but based on differentiable generator networks. GANs were first invented by Ian Goodfellow and others in 2014. Since then they have become extremely popular. This is based on game theory and has two players or networks: a generator network and b) a discriminator network, both competing against each other. This dual network game theory-based approach vastly improved the process of learning from unlabeled data. The generator network produces fake data and passes it to a discriminator. The discriminator network also sees real data and predicts whether the data it receives is fake or...
You're reading from Practical Convolutional Neural Networks
Pix2pix - Image-to-Image translation GAN
This network uses a conditional generative adversarial network (cGAN) to learn mapping from the input and output of an image. Some of the examples that can be done from the original paper are as follows:
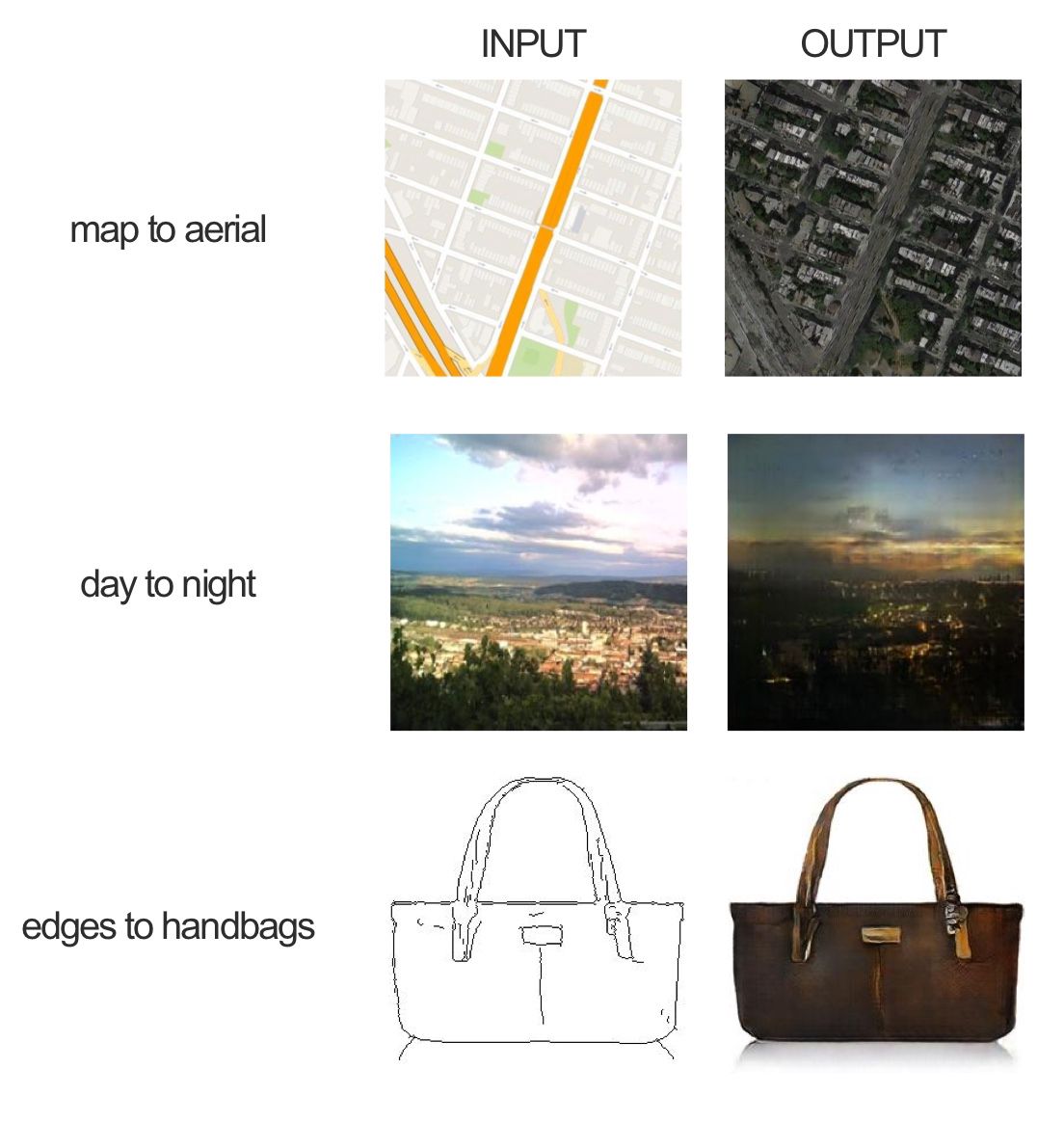
In the handbags example, the network learns how to color a black and white image. Here, the training dataset has the input image in black and white and the target image is the color version.
CycleGAN
CycleGAN is also an image-to-image translator but without input/output pairs. For example, to generate photos from paintings, convert a horse image into a zebra image:
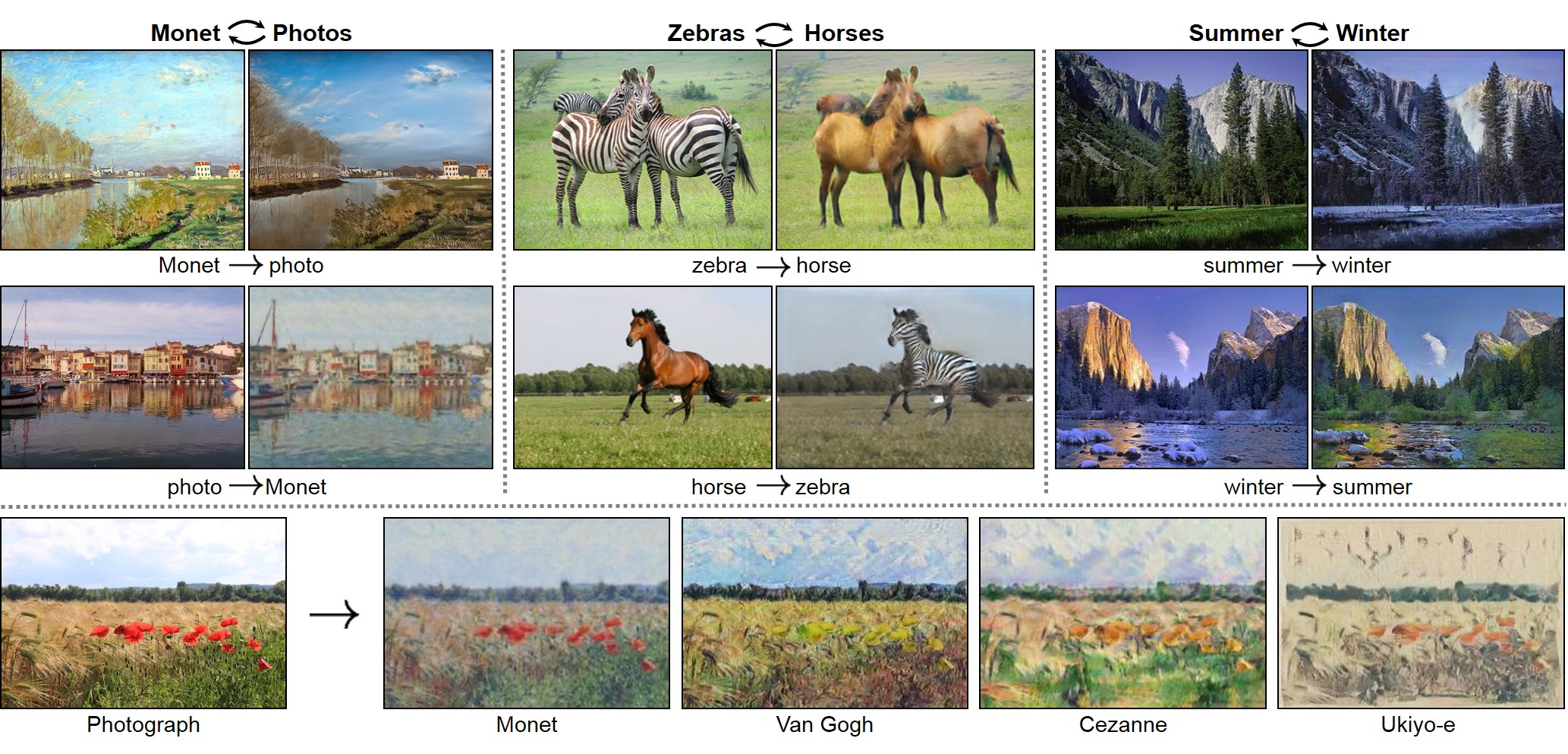
This network uses a conditional generative adversarial network (cGAN) to learn mapping from the input and output of an image. Some of the examples that can be done from the original paper are as follows:
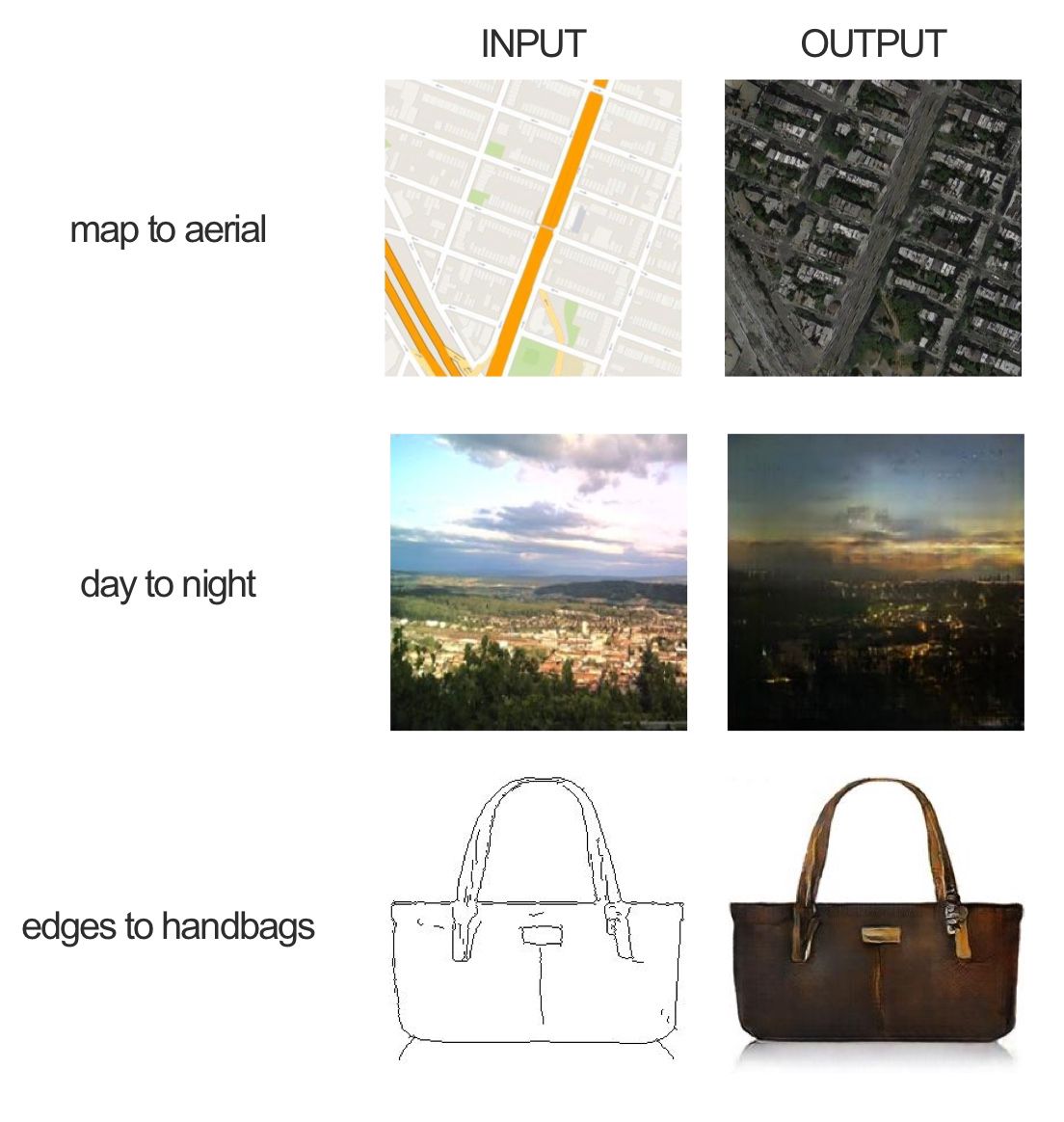
Pix2pix examples of cGANs
In the handbags example, the network learns how to color a black and white image. Here, the training dataset has the input image in black and white and the target image is the color version.
CycleGAN is also an image-to-image translator but without input/output pairs. For example, to generate photos from paintings, convert a horse image into a zebra image:
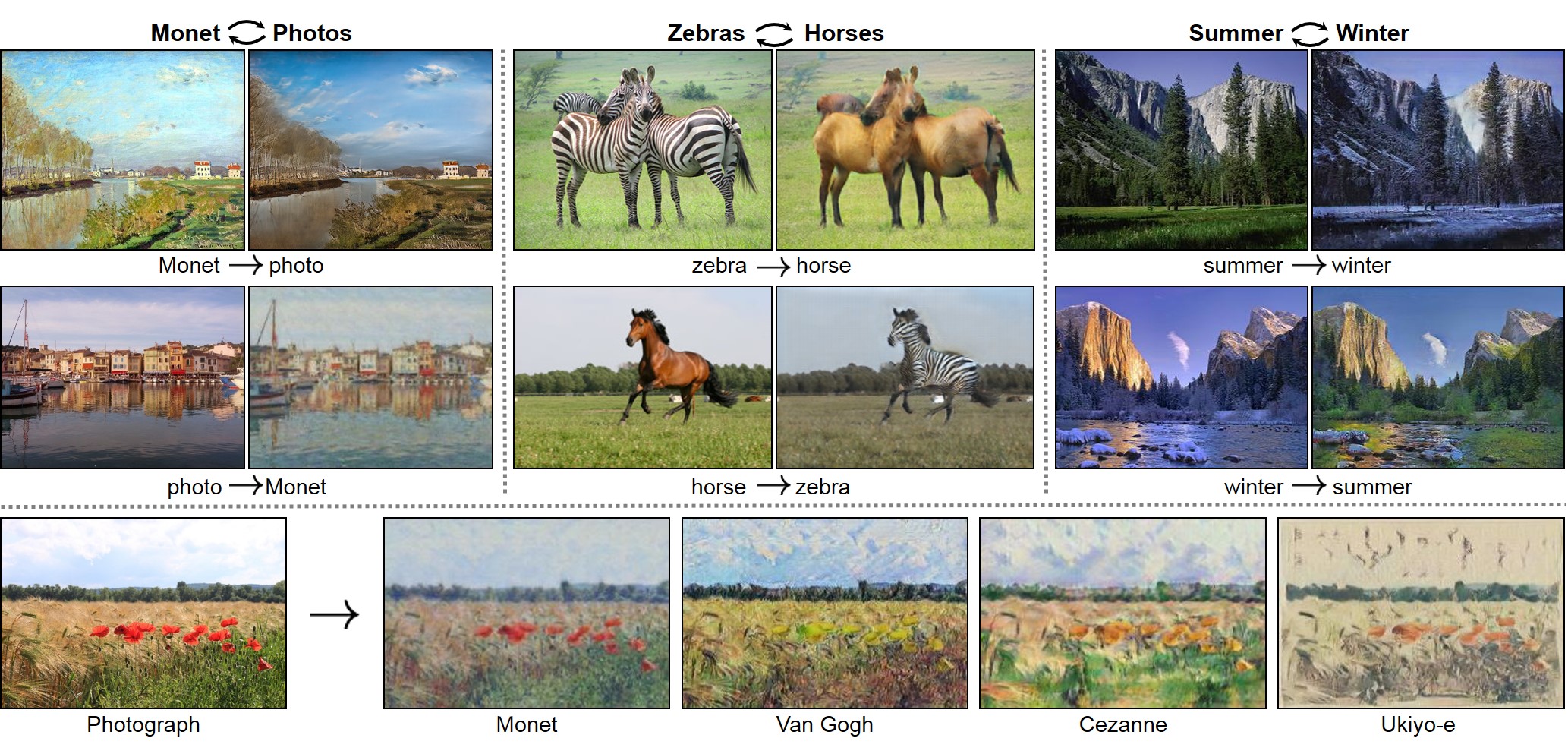
Note
In a discriminator network, use of dropout is important. Otherwise, it may produce a poor result.
The generator network takes random noise as input and produces a realistic image as output. Running a generator network for different kinds of random noise produces different types of realistic images. The second network, which is known as the discriminator network...
In the following example, we build and train a GAN model using an MNIST dataset and using TensorFlow. Here, we will use a special version of the ReLU activation function known as Leaky ReLU. The output is a new type of handwritten digit:
Note
Leaky ReLU is a variation of the ReLU activation function given by the formula f(x) = max(α∗x,x). So the output for the negative value for x is alpha * x and the output for positive x is x.
#import all necessary libraries and load data set
%matplotlib inline
import pickle as pkl
import numpy as np
import tensorflow as tf
import matplotlib.pyplot as plt
from tensorflow.examples.tutorials.mnist import input_data
mnist = input_data.read_data_sets('MNIST_data')
In order to build this network, we need two inputs, one for the generator and one for the discriminator. In the following code, we create placeholders for real_input
for the discriminator and z_input
for the generator, with the input sizes as dim_real
and dim_z
, respectively:
#place...
The idea of feature matching is to add an extra variable to the cost function of the generator in order to penalize the difference between absolute errors in the test data and training data.
In this section, we explain how to use GAN to build a classifier with the semi-supervised learning approach.
In supervised learning, we have a training set of inputs X
and class labels y
. We train a model that takes X
as input and gives y
as output.
In semi-supervised learning, our goal is still to train a model that takes X
as input and generates y
as output. However, not all of our training examples have a label y
. ;
We use the SVHN dataset. We'll turn the GAN discriminator into an 11 class discriminator (0 to 9 and one label for the fake image). It will recognize the 10 different classes of real SVHN digits, as well as an eleventh class of fake images that come from the generator. The discriminator will get to train on real labeled images...