When performing analyses, most analysts will use what is called summary statistics. Summary statistics is defined as descriptive statistics that are used to summarize a larger set of observations. These types of statistics are usually used because the larger set of observations is too large to analyze efficiently. For instance, an SEO analyst might use the average click-through as a measure of performance for their SEO efforts on two separate days of analysis.
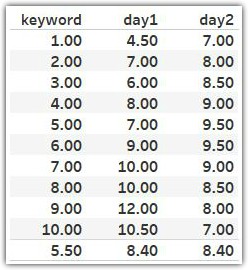
The analyst finds that the average click-through rate for both days was identical at 8.4. However, here is what is revealed when visualizing the data:
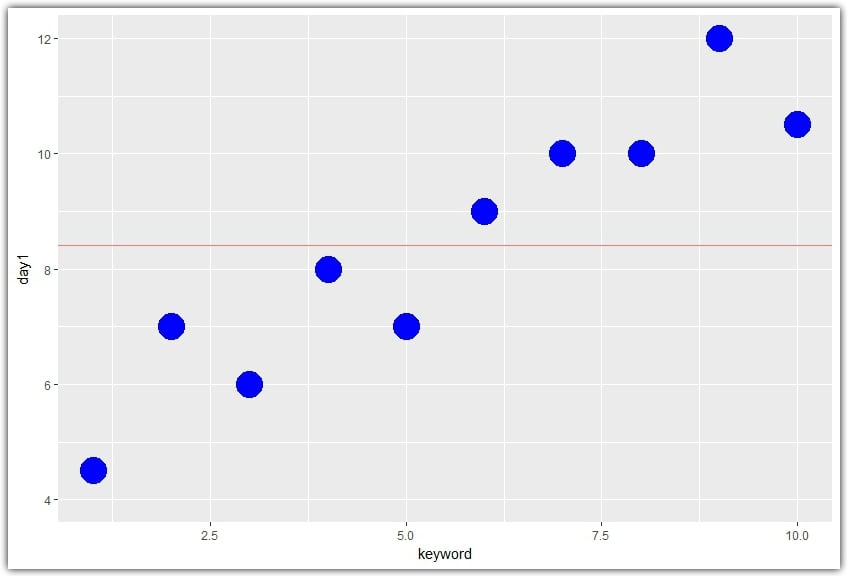
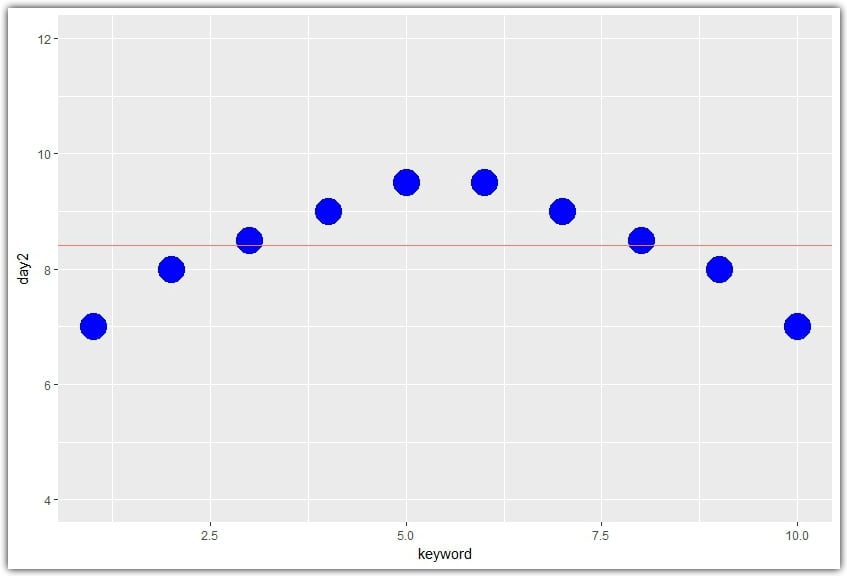
The analyst was able to see how the data was extremely different when visualized rather than just summarized.