Data Types and Conversions
If you inspect any of the output data tables from any of the nodes described previously, you will see a table-like representation of the data. Here, each value is identified via RowID
, the identification number for the record, and via a column header, the name of the attribute (Figure 2.6). So, the gender of CustomerKey 11000
is M
, as identified via the Gender
column header, and the row ID is Row0
. In a reader node, the row ID and column header can be generated automatically or assigned from the values in a column or a row in the data.
The following is a screenshot of the data table output by the File Reader node:
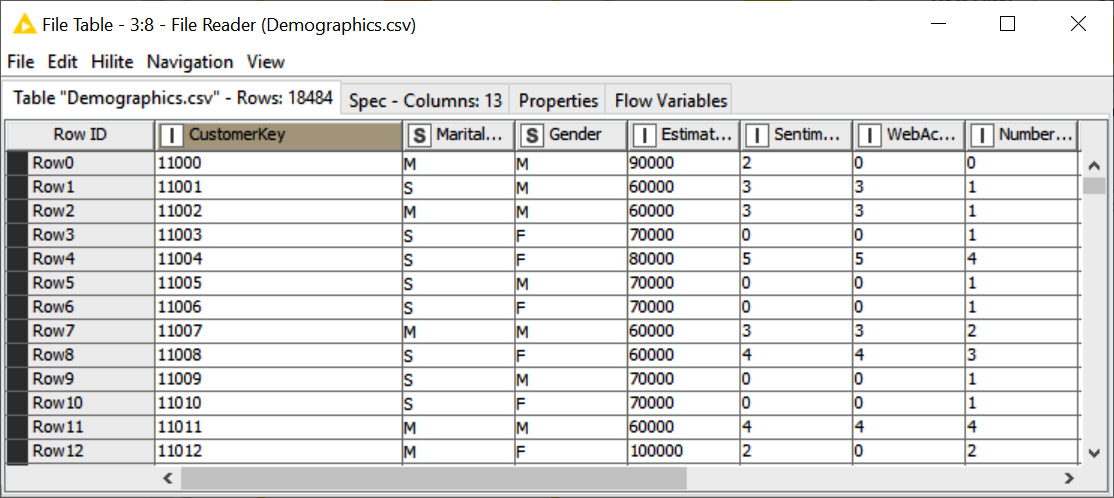
Figure 2.6 – A KNIME data table. Here, a cell is identified via its RowID value and column header
Each data column also has a data type, as you can see in Figure 2.6 from the icons in the column headers. Basic data types are Integer, Double, Boolean (true/false
), and String. However, more complex data types...