Superset uses the Flask-AppBuilder framework (fabmanager) to store and manage data for authentication, user permissions, and user roles in Superset.
After installing fabmanager in the Python virtual environment, we use the create-admin command in fabmanager and specify Superset as the app. The Flask-AppBuilder framework will create a metadata database using SQLite by default in the ~/.superset location:
# On the Terminal to setup FlaskAppBuilder for superset on GCE
# Create an admin user (you will be prompted to set username, first and last name before setting a password) (supervenv) fabmanager create-admin --app superset
After creating the admin user for the Superset app, we have to run the following commands to create tables and update columns in the metadata database:
# Initialize the database (supervenv) superset db upgrade
# Creates default roles and permissions
(supervenv) superset init
We can do a sanity check to verify that the metadata database has been created in the expected location. For this, we install sqlite3 to query the SQLite metadata database:
# Install sqlite3
(superenv) sudo apt-get install sqlite3
# Navigate to the home directory
(supervenv) cd ~/.superset
# Verify database is created
(supervenv) sqlite3
> .open superset.db
> .tables
sqlite> .tables
ab_permission annotation_layer logs
ab_permission_view clusters metrics
ab_permission_view_role columns query
ab_register_user css_templates saved_query
ab_role dashboard_slices slice_user
ab_user dashboard_user slices
ab_user_role dashboards sql_metrics
ab_view_menu datasources table_columns
access_request dbs tables
alembic_version favstar url
annotation keyvalue
Finally, let's start the Superset web server:
# run superset webserver
(supervenv) superset runserver
Go to http://<your_machines_external_ip>:8088 in your Chrome or Firefox web browser. The external IP I used is the one specified for the GCE instance I am using. Open the web app in your browser and log in with the admin credentials you entered when using the create-admin command on fabmanager.
After the login screen, you will see the welcome screen of your Superset web app:
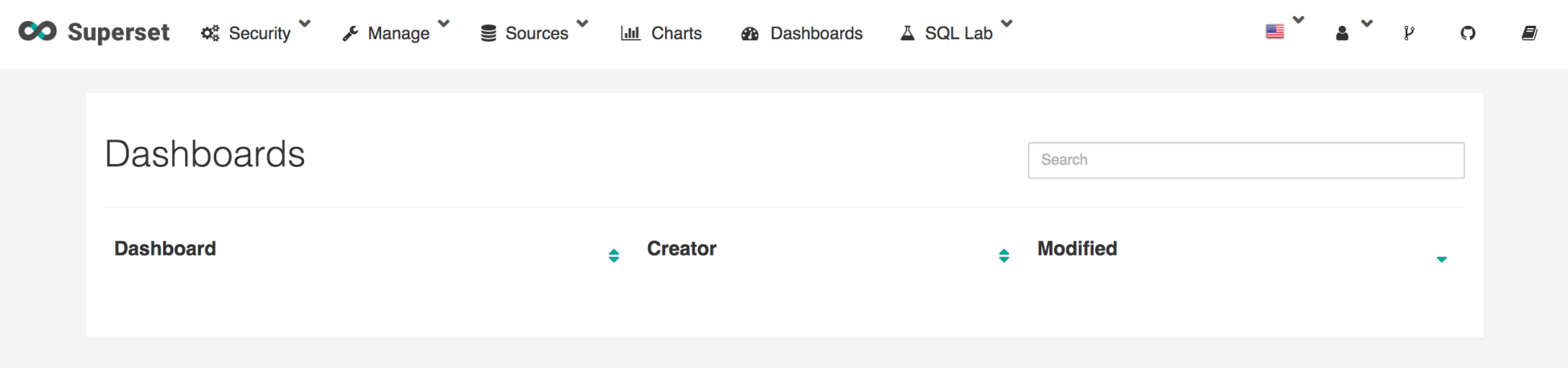