Distributed computing for big data
Before the advent of big data, ELT and ETL tools usually had a server and an orchestrator that was responsible for reading the data from the OLTP systems and populating the data warehouse. Some of these tools used the compute of these intermediate servers, while others used the compute of the target to process the data. Traditionally, these ETL/ELT systems were used to pull data once a day and during off-business hours. This was done to reduce the impact of the data being pulled from the OLTP systems. When a system required higher data processing capabilities, organizations would scale up the ETL/ELT servers.
This arrangement worked fine for a few years but the volume of data kept increasing, and scaling the ETL/ELT systems became cost prohibitive. With the world increasingly becoming more data-centric, the amount of data produced continued to grow. It is estimated that 90% of the data today has been generated in the last 2 years.
Not only has the volume of data increased, but organizations also want to get the data faster for quicker decision-making.
In a connected world, the number of variables that impact a business decision has increased, so there is a need to get data from multiple different sources to make a decision. For example, for a retail company to find out the discount to be applied to a certain product, it can no longer just rely on the cost price of the product and the profit that it expects from the sale. It would be beneficial to know the cost of keeping the product on the shelf before it is sold, along with knowing the approximate time for which the product is expected to stay on the shelf. The retail company may also want to know the price of the same product on competitor websites, along with the price of similar products with better features.
Here, the cost price can be obtained from the company’s ERP data. The percentage of expected profit might be a business transformation logic that uses their “secret sauce.” The cost of keeping the product on the shelf will be based on the cumulative sum of all the costs of the store. The approximate duration for which the product will be on the shelf might come from an ML model. The price of the same product sold by the competitors can be scraped from their websites and the cost of similar products with better features can be obtained from third-party market research. So, modern decision-making involves making sense of data from a variety of sources.
Big data is a collection of data derived from various sources and is characterized by the volume, velocity, variety, veracity, and value of the data. These are known as the 5 V’s of big data. While we collect the data from a variety of sources at a certain velocity and volume like never before, we also want to make sure that the collected data is accurate and can be trusted. This can be achieved using a series of validation steps based on the data being collected. Finally, once we have the trusted data, we want to be able to derive value from it.
When importing the data into a data lake or a data warehouse, the old arrangements of scaling up do not work, so we must deal with the 5 V’s of big data. The solution to these challenges came in the form of distributed computing.
Distributed computing systems distribute the workload of any given query to multiple workers instead of a single worker. The workloads being distributed across multiple worker nodes meant that organizations could now add nodes to increase the computing power rather than vertically scaling the node. The advantage of this approach is that we can process data on multiple nodes in parallel. This allows us to keep up with the high velocity of incoming data where one single node may not be enough.
With the advent of distributed computing in big data processing and analytics, several engines and frameworks were developed to handle different aspects of data processing and analysis. One of the most popular processing and analytics engines is Apache Spark.
Apache Spark
Apache Spark is an open source unified analytics engine that was originally developed in 2009 at UC Berkeley. It became a top-level Apache project in February 2014. It has over 1.7K contributors and over 30K star gazers on GitHub. The following is a quote from the Spark documentation (https://spark.apache.org/docs/latest/index.html):
“Apache Spark is a unified analytics engine for large-scale data processing. It provides high-level APIs in Java, Scala, Python, and R, and an optimized engine that supports general execution graphs. It also supports a rich set of higher-level tools including Spark SQL for SQL and structured data processing, MLlib for machine learning, GraphX for graph processing, and Structured Streaming for incremental computation and stream processing.”
At a high level, a Spark cluster consists of a set of executors running a Java Virtual Machine (JVM). One of these executors runs the Driver program. This driver program is responsible for creating a SparkContext. A SparkContext is the entry point for Spark features. Spark applications are instances of this SparkContext, which connects to a Cluster Manager.
The following diagram shows the workflow that’s used by Apache Spark to execute the workload. Here, the user submits the workload using the spark-submit
command; then the Spark driver coordinates with the Cluster Manager to execute the workload within the executors on the worker nodes:
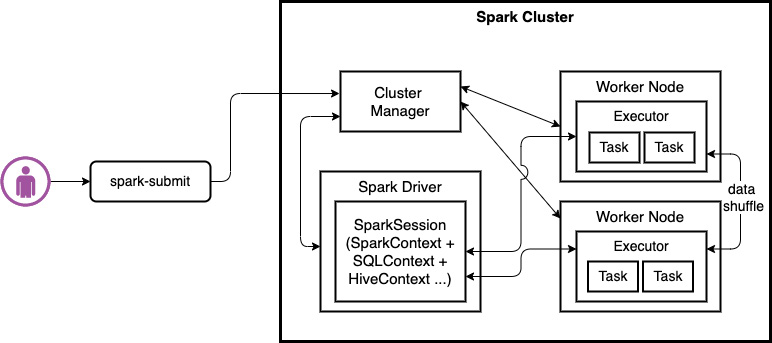
Figure 1.1 – Overview of Apache Spark’s workload execution
A Cluster Manager can be Spark’s standalone cluster manager, Mesos, Apache Hadoop Yet Another Resource Negotiator (YARN), or Kubernetes. Cluster Managers are responsible for allocating containers to various Spark applications running on the cluster. With YARN, Spark can run in either cluster mode or client mode.
In client mode, the driver program runs on the machine that submitted the Spark Job. In cluster mode, the driver program runs on one of the executors. Executors are responsible for executing the tasks that are sent through SparkContext
and run in YARN’s JVMs containers. When we invoke an action in a Spark application, a Spark Job is created. A list of actions available in Spark can be found in the Apache Spark documentation (https://spark.apache.org/docs/latest/rdd-programming-guide.html#actions). To execute a Job, an execution plan must be created based on a Directed Acyclic Graph (DAG).
A DAG scheduler converts the logical execution plan into a physical execution plan. A DAG consists of stages. A Spark stage is a set of independent tasks all computing the same function that is needed as part of a Spark Job. Each stage is further divided into tasks. All of these tasks can be run in parallel on the CPU cores of the executors. Once Spark acquires the executors, SparkContext
sends the tasks to the executors to perform.
Spark also has a component called SparkSQL
which allows users to write SQL queries for data transformation. SparkSQL
is enabled by the Catalyst and Tungsten engines.
Catalyst is responsible for creating a physical plan from a logical plan, while Tungsten is responsible for generating the byte code that will be executed on the cluster.
This new architecture of data processing came with challenges. Organizations now had to quickly develop a new skill set to manage clusters of nodes that were used for data processing. Also, what do you do with all these ETL compute nodes when they are not used for processing?
Apache Spark on the AWS cloud
The problem of unused compute resources was solved by the hyperscalers of the world. One of the leading hyperscalers is AWS. AWS has two offerings for managed Spark: Amazon EMR and AWS Glue. With Amazon EMR, customers get higher control of the underlying compute and can run Spark workloads on Amazon EC2 instances, on Amazon Elastic Kubernetes Service (EKS) clusters, or on-premises using EMR on AWS Outposts. Customers can also work with other open source tools such as Apache Spark, Apache Hive, Apache HBase, Apache Flink, Apache Hudi, and Presto on Amazon EMR.