In this section, we will be learn how to create code using the scikit-learn package to build and test decision trees. Scikit-learn contains many simple sets of functions. In fact, except for the second line of code that you can see in the following screenshot, which is specifically about decision trees, we will use the same functions for other classifiers as well, such as random forests:
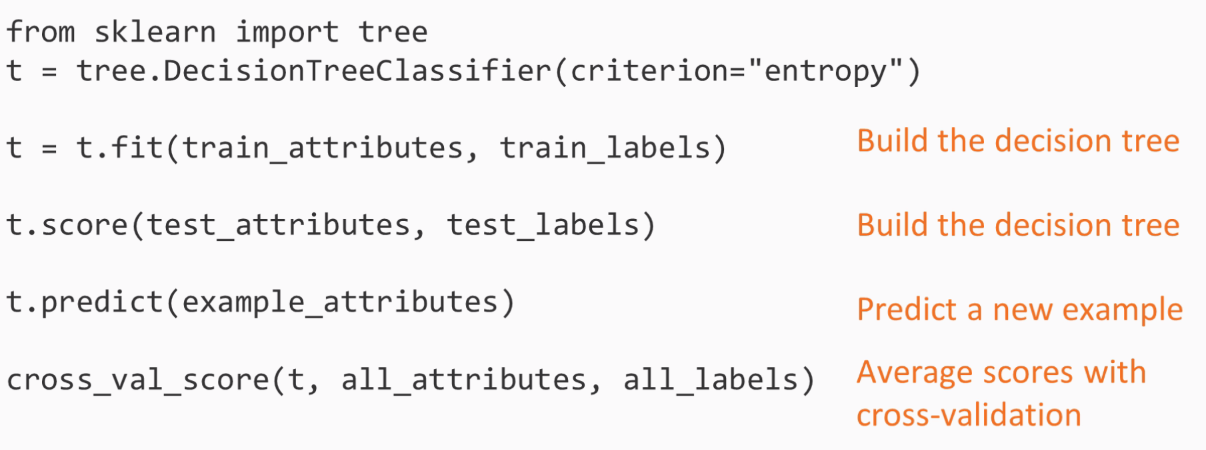
Before we jump further into technical part, let's try to understand what the lines of code mean. The first two lines of code are used to set a decision tree, but we can consider this as not yet built as we have not pointed the tree to any trained set. The third line builds the tree using the fit
function. Next, we score a list of examples and obtain an accuracy number. These two lines of code will be used to build the decision tree. After which, we predict function with a single example, which means we will take a row of data to train the model and predict the output with the survived column. Finally, we runs cross-validation, splitting the data and building an entry for each training split and evaluating the tree for each testing split. On running these code the result we have are the scores and the we average the scores.
Here you will have a question: When should we use decision trees? The answer to this can be quite simple as decision trees are simple and easy to interpret and require little data preparation, though you cannot consider them as the most accurate techniques. You can show the result of a decision tree to any subject matter expert, such as a Titanic historian (for our example). Even experts who know very little about machine learning would presumably be able to follow the tree's questions and gauge whether the tree is accurate.
Decision trees can perform better when the data has few attributes, but may perform poorly when the data has many attributes. This is because the tree may grow too large to be understandable and could easily overfit the training data by introducing branches that are too specific to the training data and don't really bear any relation to the test data created, this can reduce the chance of getting an accurate result. As, by now, you are aware of the basics of the decision tree, we are now ready to achieve our goal of creating a prediction model using student performance data.