In the previous chapter, we discussed classifying time series data for multi-variate features. In this chapter, we will create a long short-term memory (LSTM) neural network to classify univariate time series data. Our neural network will learn how to classify a univariate time series. We will have UCI (short for University of California Irvine) synthetic control data on top of which the neural network will be trained. There will be 600 sequences of data, with every sequence separated by a new line to make our job easier. Every sequence will have values recorded at 60 time steps. Since it is a univariate time series, we will only have columns in CSV files for every example recorded. Every sequence is an example recorded. We will split these sequences of data into train/test sets to perform training and evaluation...
You're reading from Java Deep Learning Cookbook
Technical requirements
This chapter's implementation code can be found at https://github.com/PacktPublishing/Java-Deep-Learning-Cookbook/blob/master/07_Constructing_LSTM_Neural_network_for_sequence_classification/sourceCode/cookbookapp/src/main/java/UciSequenceClassificationExample.java.
After cloning our GitHub repository, navigate to the Java-Deep-Learning-Cookbook/07_Constructing_LSTM_Neural_network_for_sequence_classification/sourceCode directory. Then import the cookbookapp project as a Maven project by importing pom.xml.
Download the data from this UCI website: https://archive.ics.uci.edu/ml/machine-learning-databases/synthetic_control-mld/synthetic_control.data.
We need to create directories to store the train and test data. Refer to the following directory structure:
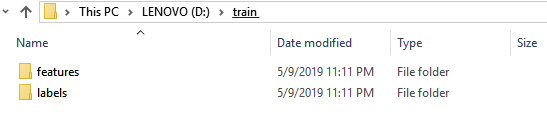
We need to create two separate folders for the train and test datasets and then create subdirectories...
Extracting time series data
We are using another time series use case, but this time we are targeting time series univariate sequence classification. ETL needs to be discussed before we configure the LSTM neural network. Data extraction is the first phase in the ETL process. This recipe covers data extraction for this use case.
How to do it...
- Categorize the sequence data programmatically:
// convert URI to string
final String data = IOUtils.toString(new URL(url),"utf-8");
// Get sequences from the raw data
final String[] sequences = data.split("\n");
final List<Pair<String,Integer>> contentAndLabels = new ArrayList<>();
int lineCount = 0;
for(String sequence : sequences) {
// Record...
Loading training data
Data transformation is, as usual, the second phase after data extraction. The time series data we're discussing doesn't have any non-numeric fields or noise (it had already been cleaned). So we can focus on constructing the iterators from the data and loading them directly into the neural network. In this recipe, we will load univariate time series data for neural network training. We have extracted the synthetic control data and stored it in a suitable format so the neural network can process it effortlessly. Every sequence is captured over 60 time steps. In this recipe, we will load the time series data into an appropriate dataset iterator, which can be fed to the neural network for further processing.
How to do it...
Normalizing training data
Data transformation alone may not improve the neural network's efficiency. The existence of large and small ranges of values within the same dataset can lead to overfitting (the model captures noise rather than signals). To avoid these situations, we normalize the dataset, and there are multiple DL4J implementations to do this. The normalization process converts and fits the raw time series data into a definite value range, for example, (0, 1). This will help the neural network process the data with less computational effort. We also discussed normalization in previous chapters, showing that it will reduce favoritism toward any specific label in the dataset while training a neural network.
How to do it...
Constructing input layers for the network
Layer configuration is an important step in neural network configuration. We need to create input layers to receive the univariate time series data that was loaded from disk. In this recipe, we will construct an input layer for our use case. We will also add an LSTM layer as a hidden layer for the neural network. We can use either a computation graph or a regular multilayer network to build the network configuration. In most cases, a regular multilayer network is more than enough; however, we are using a computation graph for our use case. In this recipe, we will configure input layers for the network.
How to do it...
- Configure the neural network with default configurations:
NeuralNetConfiguration...
Constructing output layers for the network
The very next step after the input/hidden layer design is the output layer design. As we mentioned in earlier chapters, the output layer should reflect the output you want to receive from the neural network. You may need a classifier or a regression model depending on the use case. Accordingly, the output layer has to be configured. The activation function and error function need to be justified for their use in the output layer configuration. This recipe assumes that the neural network configuration has been completed up to the input layer definition. This is going to be the last step in network configuration.
How to do it...
- Use setOutputs() to set the output labels:
compGraphBuilder...
Evaluating the LSTM network for classified output
Now that we have configured the neural network, the next step is to start the training instance, followed by evaluation. The evaluation phase is very important for the training instance. The neural network will try to optimize the gradients for optimal results. An optimal neural network will have good and stable evaluation metrics. So it is important to evaluate the neural network to direct the training process toward the desired results. We will use the test dataset to evaluate the neural network.
In the previous chapter, we explored a use case for time series binary classification. Now we have six labels against which to predict. We have discussed various ways to enhance the network's efficiency. We follow the same approach in the next recipe to evaluate the neural network for optimal results.