In the last chapter, we learned how MAML is used for finding an optimal parameter that's generalizable across several tasks. We saw how MAML computes this optimal parameter by calculating meta gradients and performing meta optimization. We also saw adversarial meta learning, which acts as an enhancement to MAML by adding adversarial samples and allowing MAML to wrestle between clean and adversarial samples to find the optimal parameter. We also saw CAML—or, context adaptation for meta learning. In this chapter, we'll learn about Meta-SGD, another meta learning algorithm that's used for performing learning quickly. Unlike MAML, Meta-SGD will not just find the optimal parameter, it will also find the optimal learning rate and an update direction. We'll see how to use Meta-SGD in supervised and reinforcement learning settings. We'll also see how to build Meta-SGD from scratch. Going ahead, we'll learn about the Reptile algorithm, which acts an improvement to...
You're reading from Hands-On Meta Learning with Python
Let's say we have some task, T. We use a model,
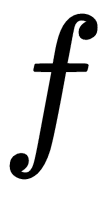
, parameterized by some parameter,
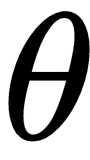
, and train the model to minimize the loss. We minimize the loss using gradient descent and find the optimal parameter
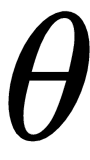
for the model.
Let's recall the update rule of a gradient descent:

So, what are the key elements that make up our gradient descent? Let's see:
- Parameter
- Learning rate
- Update direction
We usually set the parameter
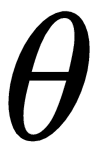
to some random value and try to find the optimal value during our training process, and we set the value of learning rate
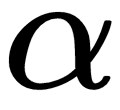
to a small number or decay it over time and an update direction that follows the gradient. Can we learn all of these key elements of the gradient descent by meta learning so that we can learn quickly from a few data points? We've already seen, in the last chapter, how MAML finds the optimal initial parameter
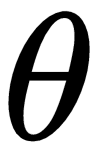
that's generalizable across tasks. With the optimal initial parameter, we can take fewer gradient steps and learn quickly on a new task.
So, now can...
The Reptile algorithm has been proposed as an improvement to MAML by OpenAI. It's simple and easier to implement. We know that, in MAML, we calculate second order derivatives—that is, the gradient of gradients. But computationally, this isn't an efficient task. So, OpenAI came up with an improvement over MAML called Reptile. The algorithm of Reptile is very simple. Sample some n number of tasks and run Stochastic Gradient Descent (SGD) for fewer iterations on each of the sampled tasks and then update our model parameter in a direction that's common to all of the tasks. Since we're performing SGD for fewer iterations on each task, it indirectly implies we're calculating the second order derivative over the loss. Unlike MAML, it's computationally effective as we're not calculating the second order derivative directly nor unrolling the computational graph, and so it is easier to implement.
Let's say we sampled two tasks,
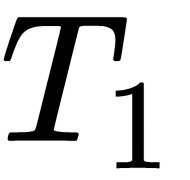
and
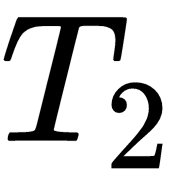
, from the task distribution and we randomly initialize the...
In this chapter, we've learned about Meta-SGD and the Reptile algorithm. We saw how Meta-SGD differs from MAML and how Meta-SGD is used in supervised and reinforcement learning settings. We saw how Meta-SGD learns the model parameter along with learning rate and update direction. We also saw how to build Meta-SGD from scratch. Then, we learned about the Reptile algorithm. We saw how Reptile differs from MAML and how Reptile acts as an improvement over the MAML algorithm. We also learned how to use Reptile in a sine wave regression task.
In the next chapter, we'll learn how we can use gradient agreement as an optimization objective in meta learning.
- Meta-SGD: https://arxiv.org/pdf/1707.09835.pdf
- Reptile: https://arxiv.org/pdf/1803.02999.pdf