The value iteration method
In the simplistic example you just saw, to calculate the values of the states and actions, we exploited the structure of the environment: we had no loops in transitions, so we could start from terminal states, calculate their values, and then proceed to the central state. However, just one loop in the environment builds an obstacle in our approach. Let's consider such an environment with two states:
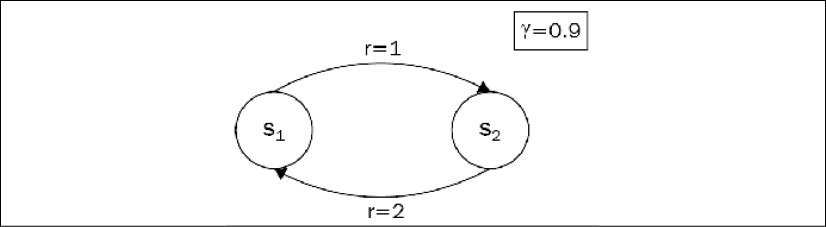
Figure 5.7: A sample environment with a loop in the transition diagram
We start from state s1, and the only action we can take leads us to state s2. We get the reward r = 1, and the only transition from s2 is an action, which brings us back to s1. So, the life of our agent is an infinite sequence of states . To deal with this infinity loop, we can use a discount factor:
. Now, the question is, what are the values for both the states? The answer is not very complicated, in fact. Every transition from s1 to s2 gives us a reward of 1 and every back...