What is data wrangling?
Data wrangling is the process of cleaning, transforming, and organizing dirty data into clean data that can be used to generate powerful insights to enable stakeholders to make the right decisions. It is basically the process of removing errors in data and making it ready for analysis. As the amount of data is growing exponentially throughout the world, it is becoming more and more important to store and organize these large datasets properly. Real-world data is often quite messy and unstructured, hence it needs to be cleaned before it can be used for any analysis.
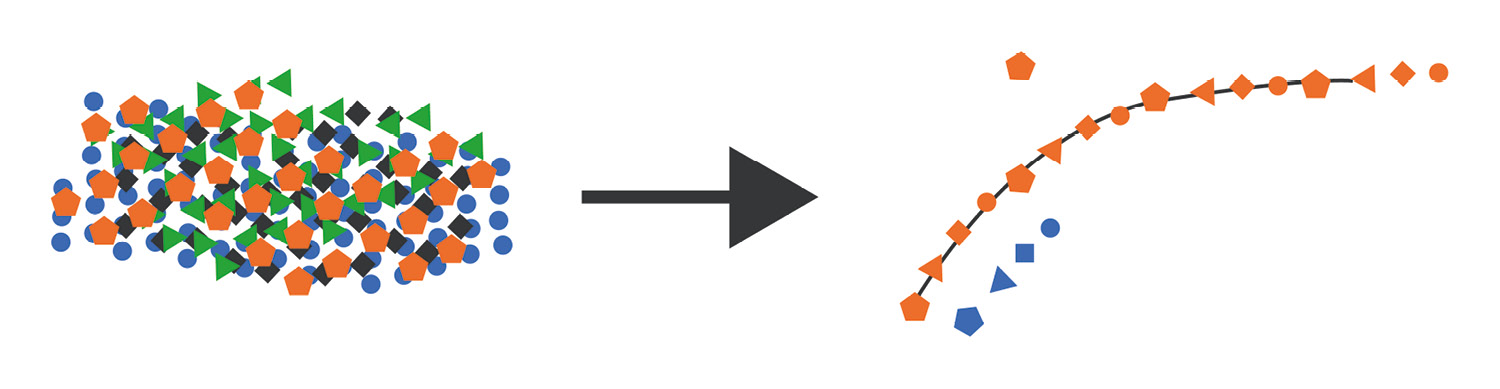
Figure 2.1 – Data wrangling
Let’s look at a few examples of data wrangling:
- Cleaning dirty data, such as missing values, bad characters, unmatched data types, and bad formatting into consistent and clean data
- Combining different datasets from multiple sources and making sure data is consistent
- Deleting data that is no longer required ...