In previous chapters, we learned about various object detection techniques, such as the R-CNN family of algorithms, YOLO, SSD, and the U-Net and Mask R-CNN image segmentation algorithms. In this chapter, we will take our learning a step further – we will work on more realistic scenarios and learn about frameworks/architectures that are more optimized to solve detection and segmentation problems. We will start by leveraging the Detectron2 framework to train and detect custom objects present in an image. We will also predict the pose of humans present in an image using a pre-trained model. Furthermore, we will learn how to count the number of people in a crowd in an image and then learn about leveraging segmentation techniques to perform image colorization. Finally, we will learn about a modified version of YOLO to predict...
You're reading from Modern Computer Vision with PyTorch
Multi-object instance segmentation
In previous chapters, we learned about various object detection algorithms. In this section, we will learn about the Detectron2 platform (https://ai.facebook.com/blog/-detectron2-a-pytorch-based-modular-object-detection-library-/) before we implement it using the Google Open Images dataset. Detectron2 is a platform built by the Facebook team. Detectron2 includes high-quality implementations of state-of-the-art object detection algorithms, including DensePose of the Mask R-CNN model family. The original Detectron framework was written in Caffe2, while the Detectron2 framework is written using PyTorch.
Detectron2 supports a range of tasks related to object detection. Like the original Detectron, it supports object detection with boxes and instance segmentation masks, as well as human pose prediction. Beyond that, Detectron2 adds support for semantic segmentation and panoptic segmentation (a task that combines both semantic and instance segmentation). By...
Human pose detection
In the previous section, we learned about detecting multiple objects and segmenting them. In this section, we will learn about detecting multiple people in an image, as well as detecting the keypoints of various body parts of the people present in the image using Detectron2. Detecting keypoints comes in handy in multiple use cases. such as in sports analytics and security.
For this exercise, we will be leveraging the pre-trained keypoint model that is available in the configuration file:
- Install all the requirements as shown in the previous section:
!pip install detectron2 -f \
https://dl...
Crowd counting
Imagine a scenario where you are given a picture of a crowd and are asked to estimate the number of people present in the image. A crowd counting model comes in handy in such a scenario. Before we go ahead and build a model to perform crowd counting, let's understand the data available and the model architecture first.
In order to train a model that predicts the number of people in an image, we will have to load the images first. The images should constitute the location of the center of the heads of all the people present in the image. A sample of the input image and the location of the center of the heads of the respective people in the image is as follows (source: ShanghaiTech dataset (https://github.com/desenzhou/ShanghaiTechDataset)):
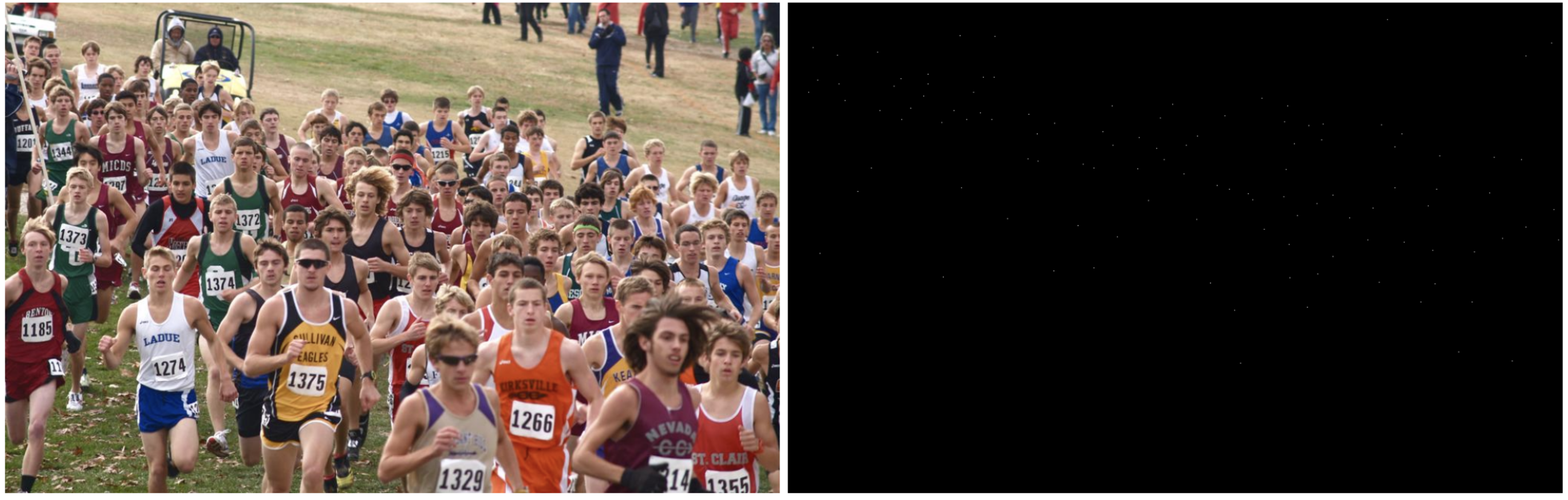
In the preceding example, the image representing ground truth (the image on the right – the center of the heads of the people present in the image) is extremely sparse. There are exactly N white pixels, where N is the number...
Image colorization
Imagine a scenario where you are given a bunch of black-and-white images and are asked to turn them into color images. How would you solve this problem? One way to solve this is by using a pseudo-supervised pipeline where we take a raw image, convert it into black and white, and treat them as input-output pairs. We will demonstrate this by leveraging the CIFAR-10 dataset to perform colorization on images.
The strategy that we will adopt as we code up the image colorization network is as follows:
- Take the original color image in the training dataset and convert it into grayscale to fetch the input (grayscale) and output (original colored image) combination.
- Normalize the input and output.
- Build a U-Net architecture.
- Train the model over increasing epochs.
With the preceding strategy in place, let's go ahead and code up the model as follows:
3D object detection with point clouds
So far, we have learned how to predict a bounding rectangle on 2D images using algorithms that have the core underlying concept of anchor boxes. We will now learn how the same concept can be extended to predict 3D bounding boxes around objects.
In a self-driving car, tasks such as pedestrian/obstacle detection and route planning cannot happen without knowing the environment. Predicting 3D object locations along with their orientations becomes an important task. Not only is the 2D bounding box around obstacles important, but also knowing the distance from the object, height, width, and orientation of the obstacle are critical to navigating safely in the 3D world.
In this section, we will learn how YOLO is used to predict the 3D orientation and position of cars and pedestrians on a real-world dataset.
Summary
In this chapter, we learned about the various practical aspects of dealing with object localization and segmentation. Specifically, we learned about how the Detectron2 platform is leveraged to perform image segmentation and detection, and keypoint detection. In addition, we also learned about some of the intricacies involved in working with large datasets when we were working on fetching images from the Open Images dataset. Next, we worked on leveraging the VGG and U-Net architectures for crowd counting and image colorization, respectively. Finally, we understood the theory and implementation steps behind 3D object detection using point cloud images. As you can see from all these examples, the underlying basics are the same as those described in the previous chapters, with modifications only in the input/output of the networks to accommodate the task at hand.
In the next chapter, we will switch gears and learn about image encoding, which helps in identifying similar images as...