What are Transformers?
Transformers are industrialized, homogenized post-deep learning models designed for parallel computing on supercomputers. Through homogenization, one transformer model can carry out a wide range of tasks with no fine-tuning. Transformers can perform self-supervised learning on billions of records of raw unlabeled data with billions of parameters.
These particular architectures of post-deep learning are called foundation models. Foundation model transformers represent the epitome of the Fourth Industrial Revolution that began in 2015 with machine-to-machine automation that will connect everything to everything. Artificial intelligence in general and specifically Natural Language Processing (NLP) for Industry 4.0 (I4.0) has gone far beyond the software practices of the past.
In less than five years, AI has become an effective cloud service with seamless APIs. The former paradigm of downloading libraries and developing is becoming an educational exercise in many cases.
An Industry 4.0 project manager can go to OpenAI’s cloud platform, sign up, obtain an API key, and get to work in a few minutes. A user can then enter a text, specify the NLP task, and obtain a response sent by a GPT-3 transformer engine. Finally, a user can go to OpenAI and create applications with no knowledge of programming. Prompt engineering is a new skill that emerged from these models.
However, sometimes a GPT-3 model might not fit a specific task. For example, a project manager, consultant, or developer might want to use another system provided by Google AI, Amazon Web Services (AWS), the Allen Institute for AI, or Hugging Face.
Should a project manager choose to work locally? Or should the implementation be done directly on Google Cloud, Microsoft Azure, or AWS? Should a development team select Hugging Face, Google Trax, OpenAI, or AllenNLP? Should an artificial intelligence specialist or a data scientist use an API with practically no AI development?
The answer is all the above. You do not know what a future employer, customer, or user may want or specify. Therefore, you must be ready to adapt to any need that comes up. This book does not describe all the offers that exist on the market. However, this book provides the reader with enough solutions to adapt to Industry 4.0 AI-driven NLP challenges.
This chapter first explains what transformers are at a high level. Then the chapter explains the importance of acquiring a flexible understanding of all types of methods to implement transformers. The definition of platforms, frameworks, libraries, and languages is blurred by the number of APIs and automation available on the market.
Finally, this chapter introduces the role of an Industry 4.0 AI specialist with advances in embedded transformers.
We need to address these critical notions before starting our journey to explore the variety of transformer model implementations described in this book.
This chapter covers the following topics:
- The emergence of the Fourth Industrial Revolution, Industry 4.0
- The paradigm change of foundation models
- Introducing prompt engineering, a new skill
- The background of transformers
- The challenges of implementing transformers
- The game-changing transformer model APIs
- The difficulty of choosing a transformer library
- The difficulty of choosing a transformer model
- The new role of an Industry 4.0 artificial intelligence specialist
- Embedded transformers
Our first step will be to explore the ecosystem of transformers.
The ecosystem of transformers
Transformer models represent such a paradigm change that they require a new name to describe them: foundation models. Accordingly, Stanford University created the Center for Research on Foundation Models (CRFM). In August 2021, the CRFM published a two-hundred-page paper (see the References section) written by over one hundred scientists and professionals: On the Opportunities and Risks of Foundation Models.
Foundation models were not created by academia but by the big tech industry. For example, Google invented the transformer model, which led to Google BERT. Microsoft entered a partnership with OpenAI to produce GPT-3.
Big tech had to find a better model to face the exponential increase of petabytes of data flowing into their data centers. Transformers were thus born out of necessity.
Let’s first take Industry 4.0 into consideration to understand the need to have industrialized artificial intelligence models.
Industry 4.0
The Agricultural Revolution led to the First Industrial Revolution, which introduced machinery. The Second Industrial Revolution gave birth to electricity, the telephone, and airplanes. The Third Industrial Revolution was digital.
The Fourth Industrial Revolution, or Industry 4.0, has given birth to an unlimited number of machine to machine connections: bots, robots, connected devices, autonomous cars, smartphones, bots that collect data from social media storage, and more.
In turn, these millions of machines and bots generate billions of data records every day: images, sound, words, and events, as shown in Figure 1.1:
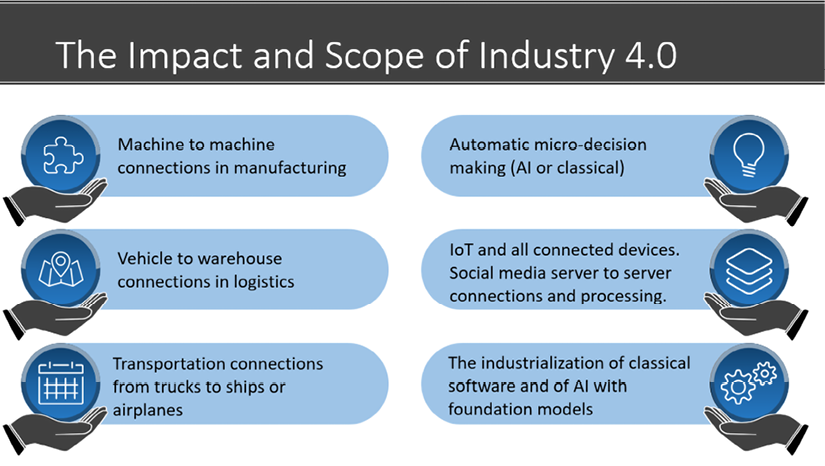
Figure 1.1: The scope of Industry 4.0
Industry 4.0 requires intelligent algorithms that process data and make decisions without human intervention on a large scale to face this unseen amount of data in the history of humanity.
Big tech needed to find a single AI model that could perform a variety of tasks that required several separate algorithms in the past.
Foundation models
Transformers have two distinct features: a high level of homogenization and mind-blowing emergence properties. Homogenization makes it possible to use one model to perform a wide variety of tasks. These abilities emerge through training billion-parameter models on supercomputers.
The paradigm change makes foundation models a post-deep learning ecosystem, as shown in Figure 1.2:
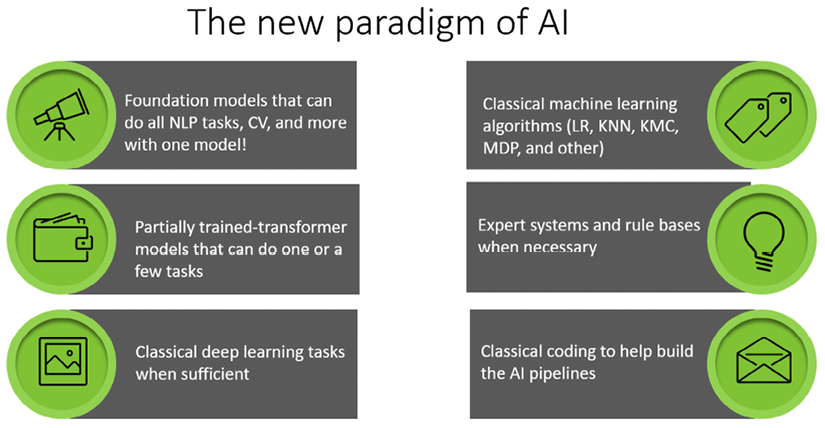
Figure 1.2: The scope of an I4.0 AI specialist
Foundation models, although designed with an innovative architecture, are built on top of the history of AI. As a result, an artificial intelligence specialist’s range of skills is stretching!
The present ecosystem of transformer models is unlike any other evolution in artificial intelligence and can be summed up with four properties:
- Model architecture
The model is industrial. The layers of the model are identical, and they are specifically designed for parallel processing. We will go through the architecture of transformers in Chapter 2, Getting Started with the Architecture of the Transformer Model.
- Data
Big tech possesses the hugest data source in the history of humanity, first generated by the Third Industrial Revolution (digital) and boosted to unfathomable sizes by Industry 4.0.
- Computing power
Big tech possesses computer power never seen before at that scale. For example, GPT-3 was trained at about 50 PetaFLOPS/second, and Google now has domain-specific supercomputers that exceed 80 PetaFLOPS/second.
- Prompt engineering
Highly trained transformers can be triggered to do a task with a prompt. The prompt is entered in natural language. However, the words used require some structure, making prompts a metalanguage.
A foundation model is thus a transformer model that has been trained on supercomputers on billions of records of data and billions of parameters. The model can then perform a wide range of tasks with no further fine-tuning. Thus, the scale of foundation models is unique. These fully trained models are often called engines. Only GPT-3, Google BERT, and a handful of transformer engines can thus qualify as foundation models.
I will only refer to foundation models in this book when mentioning OpenAI’s GPT-3 or Google’s BERT model. This is because GPT-3 and Google BERT were fully trained on supercomputers. Though interesting and effective for limited use, other models do not reach the homogenization level of foundation models due to the lack of resources.
Let’s now explore an example of how foundation models work and have changed the way we develop programs.
Is programming becoming a sub-domain of NLP?
Chen et al. (2021) published a bombshell paper in August 2021 on Codex, a GPT-3 model that can convert natural language into source code. Codex was trained on 54 million public GitHub software repositories.
Is programming now a translation task from natural language to source code languages?
Is programming becoming an NLP task for GPT-3 engines?
Let’s look into an example before answering that question.
Bear in mind that Codex is a stochastic algorithm, so the metalanguage is tricky. You might not generate what you expect if you are not careful to engineer the prompt correctly.
I created some prompts as I was experimenting with Codex. This example is just to give an idea of how Codex works and is purely for educational purposes.
My prompts were:
- “generate a random distribution of 200 integers between 1 and 100” in Python
- “plot the data using matplotlib”
- “create a k-means clustering model with 3 centroids and fit the model”
- “print the cluster labels”
- “plot the clusters”
- “plot the clusters with centroids”
Codex translated my natural metalanguage prompts into Python automatically!
Codex is a stochastic model, so it might not reproduce exactly the same code if you try again. You will have to learn the metalanguage through experimentation until you can drive it like a race car!
The Python program is generated automatically and can be copied and tested:
import numpy as np
import matplotlib.pyplot as plt
from sklearn.cluster import KMeans
from sklearn.datasets.samples_generator import make_blobs
# generate random data
np.random.seed(0)
X, y = make_blobs(n_samples=200, centers=3, n_features=2, cluster_std=2, random_state=0)
# plot the data
plt.scatter(X[:, 0], X[:, 1], s=50)
plt.show()
# create the k-means model
kmeans = KMeans(n_clusters=3, random_state=0)
# fit the model to the data
kmeans.fit(X)
# print the cluster labels
print(kmeans.labels_)
#plot the clusters
plt.scatter(X[:, 0], X[:, 1], c=kmeans.labels_, cmap='rainbow')
plt.show()
# plot the clusters with centroids
plt.scatter(X[:, 0], X[:, 1], c=kmeans.labels_, cmap='rainbow')
plt.scatter(kmeans.cluster_centers_[:, 0], kmeans.cluster_centers_[:, 1], c='black', s=100, alpha=0.5)
plt.show()
You can copy and paste this program. It works. You can also try JavaScript, among other experiments.
GitHub Copilot is now available with some Microsoft developing tools, as we will see in Chapter 16, The Emergence of Transformer-Driven Copilots. If you learn the prompt engineering metalanguage, you will reduce your development time in years to come.
End users can create prototypes and or small tasks if they master the metalanguage. In the future, coding copilots will expand.
At this point, let’s take a glimpse into the bright future of artificial intelligence specialists.
The future of artificial intelligence specialists
The societal impact of foundation models should not be underestimated. Prompt engineering has become a skill required for artificial intelligence specialists. However, the future of AI specialists cannot be limited to transformers. AI and data science overlap in I4.0.
An AI specialist will be involved in machine to machine algorithms using classical AI, IoT, edge computing, and more. An AI specialist will also design and develop fascinating connections between bots, robots, servers, and all types of connected devices using classical algorithms.
This book is thus not limited to prompt engineering but to a wide range of design skills required to be an “Industry 4.0 artificial intelligence specialist” or “I4.0 AI specialist.”
Prompt engineering is a subset of the design skills an AI specialist will have to develop. In this book, I will thus refer to the future AI specialist as an “Industry 4.0 artificial intelligence specialist.”
Let’s now get a general view of how transformers optimize NLP models.
Optimizing NLP models with transformers
Recurrent Neural Networks (RNNs), including LSTMs, have applied neural networks to NLP sequence models for decades. However, using recurrent functionality reaches its limit when faced with long sequences and large numbers of parameters. Thus, state-of-the-art transformer models now prevail.
This section goes through a brief background of NLP that led to transformers, which we’ll describe in more detail in Chapter 2, Getting Started with the Architecture of the Transformer Model. First, however, let’s have an intuitive look at the attention head of a transformer that has replaced the RNN layers of an NLP neural network.
The core concept of a transformer can be summed up loosely as “mixing tokens.” NLP models first convert word sequences into tokens. RNNs analyze tokens in recurrent functions. Transformers do not analyze tokens in sequences but relate every token to the other tokens in a sequence, as shown in Figure 1.3:
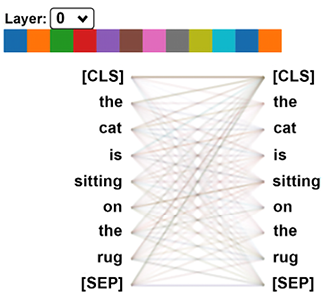
Figure 1.3: An attention head of a layer of a transformer
We will go through the details of an attention head in Chapter 2. For the moment, the takeaway of Figure 1.3 is that each word (token) of a sequence is related to all the other words of a sequence. This model opens the door to Industry 4.0 NLP.
Let’s briefly go through the background of transformers.
The background of transformers
Over the past 100+ years, many great minds have worked on sequence patterns and language modeling. As a result, machines progressively learned how to predict probable sequences of words. It would take a whole book to cite all the giants that made this happen.
In this section, I will share some of my favorite researchers with you to lay the grounds for the arrival of the Transformer.
In the early 20th century, Andrey Markov introduced the concept of random values and created a theory of stochastic processes. We know them in AI as the Markov Decision Process (MDP), Markov Chains, and Markov Processes. In the early 20th century, Markov showed that we could predict the next element of a chain, a sequence, using only the last past elements of that chain. He applied his method to a dataset containing thousands of letters using past sequences to predict the following letters of a sentence. Bear in mind that he had no computer but proved a theory still in use today in artificial intelligence.
In 1948, Claude Shannon’s The Mathematical Theory of Communication was published. Claude Shannon laid the grounds for a communication model based on a source encoder, transmitter, and a receiver or semantic decoder. He created information theory as we know it today.
In 1950, Alan Turing published his seminal article: Computing Machinery and Intelligence. Alan Turing based this article on machine intelligence on the successful Turing machine, which decrypted German messages during World War II. The messages consisted of sequences of words and numbers.
In 1954, the Georgetown-IBM experiment used computers to translate Russian sentences into English using a rule system. A rule system is a program that runs a list of rules that will analyze language structures. Rule systems still exist and are everywhere. However, in some cases, machine intelligence can replace rule lists for the billions of language combinations by automatically learning the patterns.
The expression “Artificial Intelligence” was first used by John McCarthy in 1956 when it was established that machines could learn.
In 1982, John Hopfield introduced an RNN, known as Hopfield networks or “associative” neural networks. John Hopfield was inspired by W.A. Little, who wrote The existence of persistent states in the brain in 1974, which laid the theoretical grounds of learning processes for decades. RNNs evolved, and LSTMs emerged as we know them today.
An RNN memorizes the persistent states of a sequence efficiently, as shown in Figure 1.4:

Figure 1.4: The RNN process
Each state Sn captures the information of Sn-1. When the network’s end is reached, a function F will perform an action: transduction, modeling, or any other type of sequence-based task.
In the 1980s, Yann LeCun designed the multipurpose Convolutional Neural Network (CNN). He applied CNNs to text sequences, and they also apply to sequence transduction and modeling. They are also based on W.A. Little’s persistent states that process information layer by layer. In the 1990s, summing up several years of work, Yann LeCun produced LeNet-5, which led to the many CNN models we know today. However, a CNN’s otherwise efficient architecture faces problems when dealing with long-term dependencies in lengthy and complex sequences.
We could mention many other great names, papers, and models that would humble any AI specialist. It seemed that everybody in AI was on the right track for all these years. Markov Fields, RNNs, and CNNs evolved into multiple other models. The notion of attention appeared: peeking at other tokens in a sequence, not just the last one. It was added to the RNN and CNN models.
After that, if AI models needed to analyze longer sequences requiring increasing computer power, AI developers used more powerful machines and found ways to optimize gradients.
Some research was done on sequence-to-sequence models, but they did not meet expectations.
It seemed that nothing else could be done to make more progress. Thirty years passed this way. And then, starting late 2017, the industrialized state-of-the-art Transformer came with its attention head sublayers and more. RNNs did not appear as a pre-requisite for sequence modeling anymore.
Before diving into the original Transformer’s architecture, which we will do in Chapter 2, Getting Started with the Architecture of the Transformer Model, let’s start at a high level by examining the paradigm change in software resources we should use to learn and implement transformer models.
What resources should we use?
Industry 4.0 AI has blurred the lines between cloud platforms, frameworks, libraries, languages, and models. Transformers are new, and the range and number of ecosystems are mind-blowing. Google Cloud provides ready-to-use transformer models.
OpenAI has deployed a “Transformer” API that requires practically no programming. Hugging Face provides a cloud library service, and the list is endless.
This chapter will go through a high-level analysis of some of the transformer ecosystems we will be implementing throughout this book.
Your choice of resources to implement transformers for NLP is critical. It is a question of survival in a project. Imagine a real-life interview or presentation. Imagine you are talking to your future employer, your employer, your team, or a customer.
You begin your presentation with an excellent PowerPoint with Hugging Face, for example. You might get an adverse reaction from a manager who may say, “I’m sorry, but we use Google Trax here for this type of project, not Hugging Face. Can you implement Google Trax, please?” If you don’t, it’s game over for you.
The same problem could have arisen by specializing in Google Trax. But, instead, you might get the reaction of a manager who wants to use OpenAI’s GPT-3 engines with an API and no development. If you specialize in OpenAI’s GPT-3 engines with APIs and no development, you might face a project manager or customer who prefers Hugging Face’s AutoML APIs. The worst thing that could happen to you is that a manager accepts your solution, but in the end, it does not work at all for the NLP tasks of that project.
The key concept to keep in mind is that if you only focus on the solution that you like, you will most likely sink with the ship at some point.
Focus on the system you need, not the one you like.
This book is not designed to explain every transformer solution that exists on the market. Instead, this book aims to explain enough transformer ecosystems for you to be flexible and adapt to any situation you face in an NLP project.
In this section, we will go through some of the challenges that you’ll face. But first, let’s begin with APIs.
The rise of Transformer 4.0 seamless APIs
We are now well into the industrialization era of artificial intelligence. Microsoft, Google, Amazon Web Services (AWS), and IBM, among others, offer AI services that no developer or team of developers could hope to outperform. Tech giants have million-dollar supercomputers with massive datasets to train transformer models and AI models in general.
Big tech giants have a wide range of corporate customers that already use their cloud services. As a result, adding a transformer API to an existing cloud architecture requires less effort than any other solution.
A small company or even an individual can access the most powerful transformer models through an API with practically no investment in development. An intern can implement the API in a few days. There is no need to be an engineer or have a Ph.D. for such a simple implementation.
For example, the OpenAI platform now has a SaaS (Software as a Service) API for some of the most effective transformer models on the market.
OpenAI transformer models are so effective and humanlike that the present policy requires a potential user to fill out a request form. Once the request has been accepted, the user can access a universe of natural language processing!
The simplicity of OpenAI’s API takes the user by surprise:
- Obtain an API key in one click
- Import OpenAI in a notebook in one lin
- Enter any NLP task you wish in a prompt
- You will receive the response as a completion in a certain number of tokens (length)
And that’s it! Welcome to the Fourth Industrial Revolution and AI 4.0!
Industry 3.0 developers that focus on code-only solutions will evolve into Industry 4.0 developers with cross-disciplinary mindsets.
The 4.0 developer will learn how to design ways to show a transformer model what is expected and not intuitively tell it what to do, like a 3.0 developer would do. We will explore this new approach through GPT-2 and GPT-3 models in Chapter 7, The Rise of Suprahuman Transformers with GPT-3 Engines.
AllenNLP offers the free use of an online educational interface for transformers. AllenNLP also provides a library that can be installed in a notebook. For example, suppose we are asked to implement coreference resolution. We can start by running an example online.
Coreference resolution tasks involve finding the entity to which a word refers, as in the sentence shown in Figure 1.5:
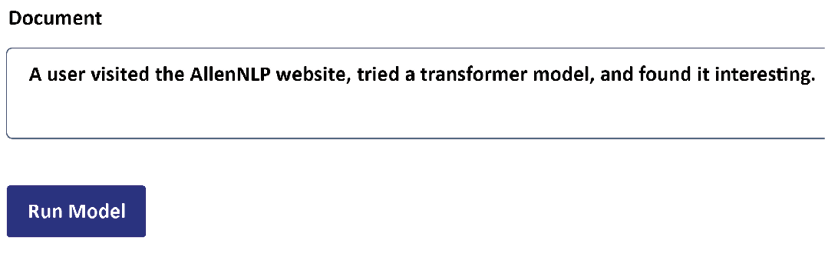
Figure 1.5: Running an NLP task online
The word “it” could refer to the website or the transformer model. In this case, the BERT-like model decided to link “it” to the transformer model. AllenNLP provides a formatted output, as shown in Figure 1.6:

Figure 1.6: The output of an AllenNLP transformer model
This example can be run at https://demo.allennlp.org/coreference-resolution. Transformer models are continuously updated, so you might obtain a different result.
Though APIs may satisfy many needs, they also have limits. A multipurpose API might be reasonably good in all tasks but not good enough for a specific NLP task. Translating with transformers is no easy task. In that case, a 4.0 developer, consultant, or project manager will have to prove that an API alone cannot solve the specific NLP task required. We need to search for a solid library.
Choosing ready-to-use API-driven libraries
In this book, we will explore several libraries. For example, Google has some of the most advanced AI labs in the world. Google Trax can be installed in a few lines in Google Colab. You can choose free or paid services. We can get our hands on source code, tweak the models, and even train them on our servers or Google Cloud. For example, it’s a step down from ready-to-use APIs to customize a transformer model for translation tasks.
However, it can prove to be both educational and effective in some cases. We will explore the recent evolution of Google in translations and implement Google Trax in Chapter 6, Machine Translation with the Transformer.
We have seen that APIs such as OpenAI require limited developer skills, and libraries such as Google Trax dig a bit deeper into code. Both approaches show that AI 4.0 APIs will require more development on the editor side of the API but much less effort when implementing transformers.
One of the most famous online applications that use transformers, among other algorithms, is Google Translate. Google Translate can be used online or through an API.
Let’s try to translate a sentence requiring coreference resolution in an English to French translation using Google Translate:
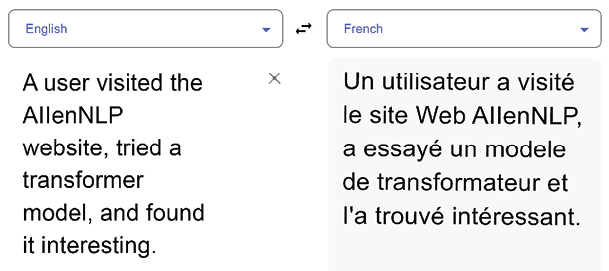
Figure 1.7: Coreference resolution in a translation using Google Translate
Google Translate appears to have solved the coreference resolution, but the word transformateur in French means an electric device. The word transformer is a neologism (new word) in French. An artificial intelligence specialist might be required to have language and linguistic skills for a specific project. Significant development is not required in this case. However, the project might require clarifying the input before requesting a translation.
This example shows that you might have to team up with a linguist or acquire linguistic skills to work on an input context. In addition, it might take a lot of development to enhance the input with an interface for contexts.
So, we still might have to get our hands dirty to add scripts to use Google Translate. Or we might have to find a transformer model for a specific translation need, such as BERT, T5, or other models we will explore in this book.
Choosing a model is no easy task with the increasing range of solutions.
Choosing a Transformer Model
Big tech corporations dominate the NLP market. Google, Facebook, and Microsoft alone run billions of NLP routines per day, increasing their AI models’ unequaled power. The big giants now offer a wide range of transformer models and have top-ranking foundation models.
However, smaller companies, spotting the vast NLP market, have entered the game. Hugging Face now has a free or paid service approach too. It will be challenging for Hugging Face to reach the level of efficiency acquired through the billions of dollars poured into Google’s research labs and Microsoft’s funding of OpenAI. The entry point of foundation models is fully trained transformers on supercomputers such as GPT-3 or Google BERT.
Hugging Face has a different approach and offers a wide range and number of transformer models for a task, which is an interesting philosophy. Hugging Face offers flexible models. In addition, Hugging Face offers high-level APIs and developer-controlled APIs. We will explore Hugging Face in several chapters of this book as an educational tool and a possible solution for specific tasks.
Yet, OpenAI has focused on a handful of the most potent transformer engines globally and can perform many NLP tasks at human levels. We will show the power of OpenAI’s GPT-3 engines in Chapter 7, The Rise of Suprahuman Transformers with GPT-3 Engines.
These opposing and often conflicting strategies leave us with a wide range of possible implementations. We must thus define the role of Industry 4.0 artificial intelligence specialists.
The role of Industry 4.0 artificial intelligence specialists
Industry 4.0 is connecting everything to everything, everywhere. Machines communicate directly with other machines. AI-driven IoT signals trigger automated decisions without human intervention. NLP algorithms send automated reports, summaries, emails, advertisements, and more.
Artificial intelligence specialists will have to adapt to this new era of increasingly automated tasks, including transformer model implementations. Artificial intelligence specialists will have new functions. If we list transformer NLP tasks that an AI specialist will have to do, from top to bottom, it appears that some high-level tasks require little to no development on the part of an artificial intelligence specialist. An AI specialist can be an AI guru, providing design ideas, explanations, and implementations.
The pragmatic definition of what a transformer represents for an artificial intelligence specialist will vary with the ecosystem.
Let’s go through a few examples:
- API: The OpenAI API does not require an AI developer. A web designer can create a form, and a linguist or Subject Matter Expert (SME) can prepare the prompt input texts. The primary role of an AI specialist will require linguistic skills to show, not just tell, the GPT-3 engines how to accomplish a task. Showing, for example, involves working on the context of the input. This new task is named prompt engineering. A prompt engineer has quite a future in AI!
- Library: The Google Trax library requires a limited amount of development to start with ready-to-use models. An AI specialist mastering linguistics and NLP tasks can work on the datasets and the outputs.
- Training and fine-tuning: Some of the Hugging Face functionality requires a limited amount of development, providing both APIs and libraries. However, in some cases, we still have to get our hands dirty. In that case, training, fine-tuning the models, and finding the correct hyperparameters will require the expertise of an artificial intelligence specialist.
- Development-level skills: In some projects, the tokenizers and the datasets do not match, as explained in Chapter 9, Matching Tokenizers and Datasets. In this case, an artificial intelligence developer working with a linguist, for example, can play a crucial role. Therefore, computational linguistics training can come in very handy at this level.
The recent evolution of NLP AI can be termed as “embedded transformers,” which is disrupting the AI development ecosystem:
- GPT-3 transformers are currently embedded in several Microsoft Azure applications with GitHub Copilot, for example.
- The embedded transformers are not accessible directly but provide automatic development support such as automatic code generation.
- The usage of embedded transformers is seamless for the end user with assisted text completion.
To access GPT-3 engines directly, you must first create an OpenAI account. Then you can use the API or directly run examples in the OpenAI user interface.
We will explore this fascinating new world of embedded transformers in Chapter 16. But to get the best out of that chapter, you should first master the previous chapters’ concepts, examples, and programs.
The skillset of an Industry 4.0 AI specialist requires flexibility, cross-disciplinary knowledge, and above all, flexibility. This book will provide the artificial intelligence specialist with a variety of transformer ecosystems to adapt to the new paradigms of the market.
It’s time to summarize the ideas of this chapter before diving into the fascinating architecture of the original Transformer in Chapter 2.
Summary
The Fourth Industrial Revolution, or Industry 4.0, has forced artificial intelligence to make profound evolutions. The Third Industrial Revolution was digital. Industry 4.0 is built on top of the digital revolution connecting everything to everything, everywhere. Automated processes are replacing human decisions in critical areas, including NLP.
RNNs had limitations that slowed the progression of automated NLP tasks required in a fast-moving world. Transformers filled the gap. A corporation needs summarization, translation, and a wide range of NLP tools to meet the challenges of Industry 4.0.
Industry 4.0 (I4.0) has thus spurred an age of artificial intelligence industrialization. The evolution of the concepts of platforms, frameworks, language, and models represents a challenge for an industry 4.0 developer. Foundation models bridge the gap between the Third Industrial Revolution and I4.0 by providing homogenous models that can carry out a wide range of tasks without further training or fine-tuning.
Websites such as AllenNLP, for example, provide educational NLP tasks with no installation, but it also provides resources to implement a transformer model in customized programs. OpenAI provides an API requiring only a few code lines to run one of the powerful GPT-3 engines. Google Trax provides an end-to-end library, and Hugging Face offers various transformer models and implementations. We will be exploring these ecosystems throughout this book.
Industry 4.0 is a radical deviation from former AI with a broader skillset. For example, a project manager can decide to implement transformers by asking a web designer to create an interface for OpenAI’s API through prompt engineering. Or, when required, a project manager can ask an artificial intelligence specialist to download Google Trax or Hugging Face to develop a full-blown project with a customized transformer model.
Industry 4.0 is a game-changer for developers whose role will expand and require more designing than programming. In addition, embedded transformers will provide assisted code development and usage. These new skillsets are a challenge but open new exciting horizons.
In Chapter 2, Getting Started with the Architecture of the Transformer Model, we will get started with the architecture of the original Transformer.
Questions
- We are still in the Third Industrial Revolution. (True/False)
- The Fourth Industrial Revolution is connecting everything to everything. (True/False)
- Industry 4.0 developers will sometimes have no AI development to do. (True/False)
- Industry 4.0 developers might have to implement transformers from scratch. (True/False)
- It’s not necessary to learn more than one transformer ecosystem, such as Hugging Face, for example. (True/False)
- A ready-to-use transformer API can satisfy all needs. (True/False)
- A company will accept the transformer ecosystem a developer knows best. (True/False)
- Cloud transformers have become mainstream. (True/False)
- A transformer project can be run on a laptop. (True/False)
- Industry 4.0 artificial intelligence specialists will have to be more flexible (True/False)
References
- Bommansani et al. 2021, On the Opportunities and Risks of Foundation Models, https://arxiv.org/abs/2108.07258
- Chen et al.,2021, Evaluating Large Language Models Trained on Code, https://arxiv.org/abs/2107.03374
- Microsoft AI: https://innovation.microsoft.com/en-us/ai-at-scale
- OpenAI: https://openai.com/
- Google AI: https://ai.google/
- Google Trax: https://github.com/google/trax
- AllenNLP: https://allennlp.org/
- Hugging Face: https://huggingface.co/
Join our book’s Discord space
Join the book’s Discord workspace:
https://www.packt.link/Transformers