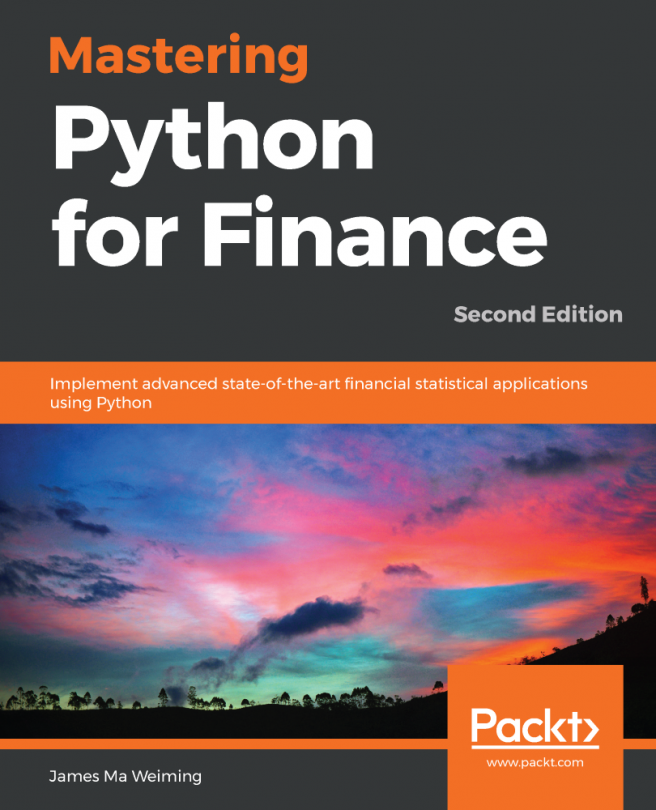
Mastering Python for Finance - Second Edition
eBook
$29.99
$20.98
Print
$43.99
Subscription
$15.99
$10 p/m for three months
What do you get with a Packt Subscription?
What do you get with a Packt Subscription?
What do you get with eBook + Subscription?
What do you get with a Packt Subscription?
What do you get with eBook?
What do I get with Print?
What do I get with Print?
What do you get with video?
What do you get with video?
What do you get with Audiobook?
What do you get with Exam Trainer?
eBook
$29.99
$20.98
Print
$43.99
Subscription
$15.99
$10 p/m for three months
What do you get with a Packt Subscription?
What do you get with a Packt Subscription?
What do you get with eBook + Subscription?
What do you get with a Packt Subscription?
What do you get with eBook?
What do I get with Print?
What do I get with Print?
What do you get with video?
What do you get with video?
What do you get with Audiobook?
What do you get with Exam Trainer?
-
Free ChapterSection 1: Getting Started with Python
-
Overview of Financial Analysis with Python
-
Section 2: Financial Concepts
-
The Importance of Linearity in Finance
- The Importance of Linearity in Finance
- The Capital Asset Pricing Model and the security market line
- The Arbitrage Pricing Theory model
- Multivariate linear regression of factor models
- Linear optimization
- Solving linear equations using matrices
- The LU decomposition
- The Cholesky decomposition
- The QR decomposition
- Solving with other matrix algebra methods
- Summary
-
Nonlinearity in Finance
-
Numerical Methods for Pricing Options
- Numerical Methods for Pricing Options
- Introduction to options
- Binomial trees in option pricing
- Pricing European options
- Writing the StockOption base class
- The Greeks for free
- Trinomial trees in option pricing
- Lattices in option pricing
- Finite differences in option pricing
- Putting it all together – implied volatility modeling
- Summary
-
Modeling Interest Rates and Derivatives
- Modeling Interest Rates and Derivatives
- Fixed-income securities
- Yield curves
- Valuing a zero-coupon bond
- Bootstrapping a yield curve
- Forward rates
- Calculating the yield to maturity
- Calculating the price of a bond
- Bond duration
- Bond convexity
- Short–rate modeling
- Bond options
- Pricing a callable bond option
- Summary
-
Statistical Analysis of Time Series Data
- Statistical Analysis of Time Series Data
- The Dow Jones industrial average and its 30 components
- Applying a kernel PCA
- Stationary and non-stationary time series
- The Augmented Dickey-Fuller Test
- Analyzing a time series with trends
- Making a time series stationary
- Forecasting and predicting a time series
- Summary
-
Section 3: A Hands-On Approach
-
Interactive Financial Analytics with the VIX
-
Building an Algorithmic Trading Platform
-
Implementing a Backtesting System
-
Machine Learning for Finance
-
Deep Learning for Finance
-
Other Books You May Enjoy
About this
book
The second edition of Mastering Python for Finance will guide you through carrying out complex financial calculations practiced in the industry of finance by using next-generation methodologies. You will master the Python ecosystem by leveraging publicly available tools to successfully perform research studies and modeling, and learn to manage risks with the help of advanced examples.
You will start by setting up your Jupyter notebook to implement the tasks throughout the book. You will learn to make efficient and powerful data-driven financial decisions using popular libraries such as TensorFlow, Keras, Numpy, SciPy, and scikit-learn. You will also learn how to build financial applications by mastering concepts such as stocks, options, interest rates and their derivatives, and risk analytics using computational methods. With these foundations, you will learn to apply statistical analysis to time series data, and understand how time series data is useful for implementing an event-driven backtesting system and for working with high-frequency data in building an algorithmic trading platform. Finally, you will explore machine learning and deep learning techniques that are applied in finance.
By the end of this book, you will be able to apply Python to different paradigms in the financial industry and perform efficient data analysis.
- Publication date:
- April 2019
- Publisher
- Packt
- Pages
- 426
- ISBN
- 9781789346466
Latest Reviews
(4 reviews total)