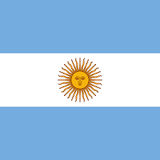
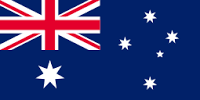
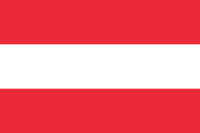
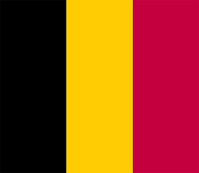
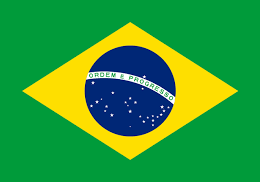
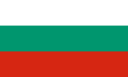
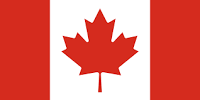
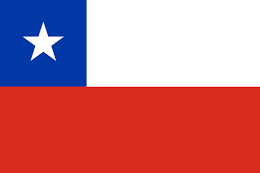
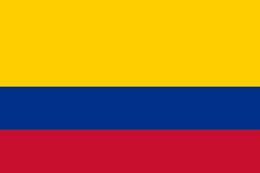
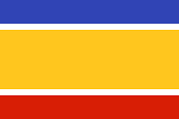
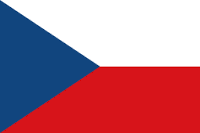
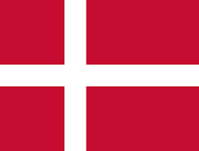
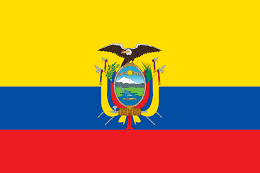
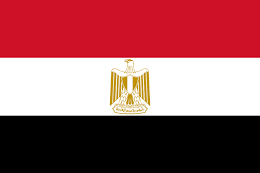
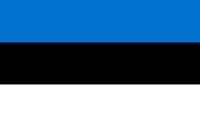
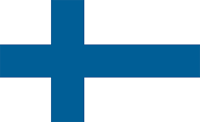
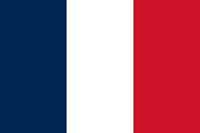
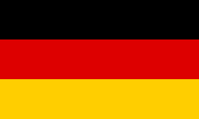
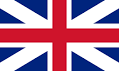
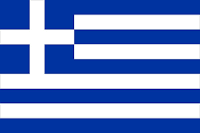
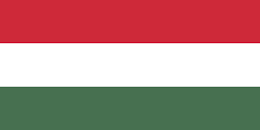
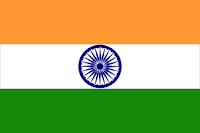
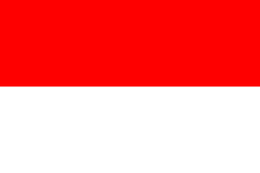
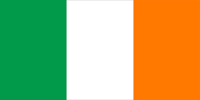
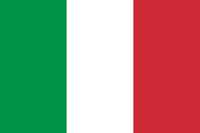
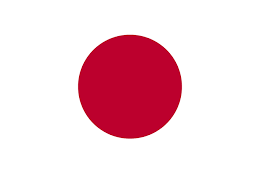
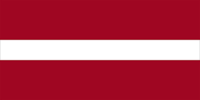
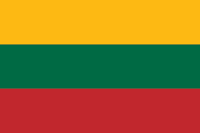
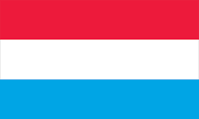
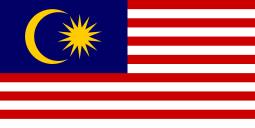
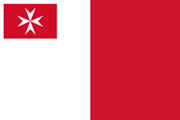
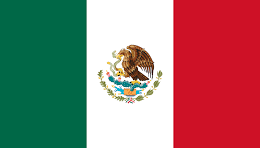
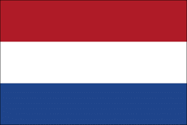
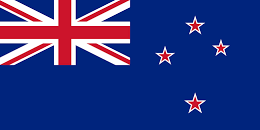
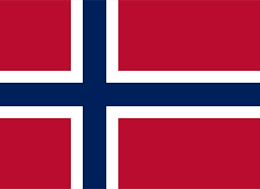
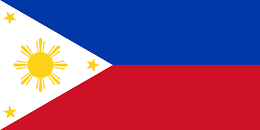
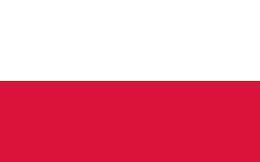
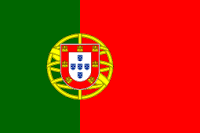
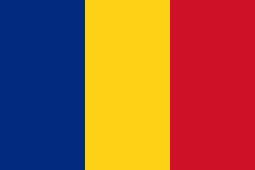
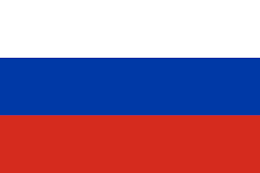

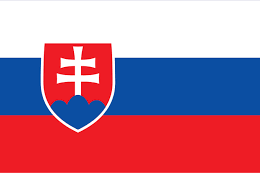
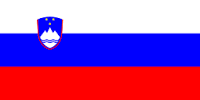
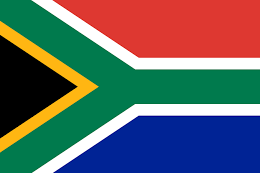
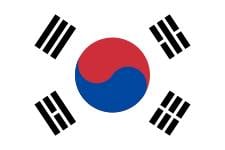
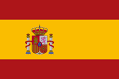
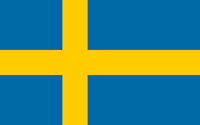
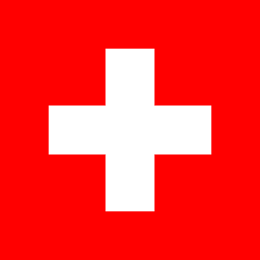
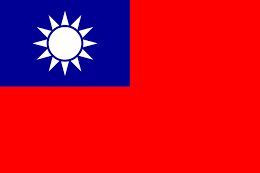
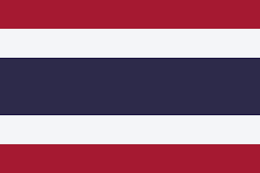
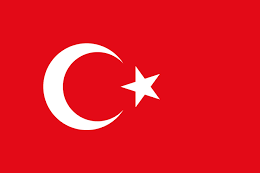
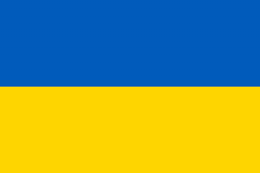
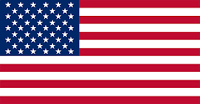
Cython is a relatively young programming language based on Python. The difference with Python is that we can optionally declare static types. Many programming languages, such as C, have static typing, which means that we have to tell C the type of variables, function parameters, and return types. Another difference is that C is a compiled language, while Python is an interpreted language. As a rule of thumb, we can say that C is faster but less flexible than Python. From Cython code, we can generate C or C++ code. After that, we can compile the generated code into Python extension modules.
In this chapter we will be learning about Cython. We will get some simple Cython programs running together with NumPy. Also we will profile Cython code.
In order to use Cython, we need to install Cython. Enthought and Sage have Cython included. For more information, see http://www.enthought.com/products/epd.php and http://sagemath.org/. We will not discuss here how to install these distributions. Obviously, we need a C compiler to compile the generated C code. On some operating systems, such as Linux, the compiler will already be present. In this recipe, we will assume that you already have the compiler installed.
Cython can be installed using any of the following methods:
Installing from tarball (.tar
archive): Cython can be installed from tarball by performing the following steps:
Download a tarball from http://cython.org/#download.
Unpack it.
Browse to the directory using the cd
command.
Run the following command:
python setup.py install
Installing with setup tools or pip
We can install Cython from the PyPI repository with easy_install cython
or sudo pip install cython
.
Installing with Windows installers
We...
As is the tradition with programming languages, we will start with a Hello World example. Unlike with Python, we need to compile Cython code. We start with a
.pyx
file, from which we will generate C code. This .c
file can be compiled and then imported into a Python program.
This section describes how to build a Cython Hello World program.
First, we will write some pretty trivial code that prints "Hello World". This is just normal Python code, but the file has the pyx
extension.
def say_hello(): print "Hello World!"
Write a distutils setup.py
script.
We need to create a file named setup.py
to help us build the Cython code.
from distutils.core import setup from distutils.extension import Extension from Cython.Distutils import build_ext ext_modules = [Extension("hello", ["hello.pyx"])] setup( name = 'Hello world app', cmdclass = {'build_ext': build_ext}, ext_modules = ext_modules )
As you can...
We can integrate Cython and NumPy code in the same way that we can integrate Cython and Python code. Let's go through an example that analyzes the ratio of up days (days on which a stock closes higher than the previous day) for a stock. We will apply the formula for binomial proportion confidence. You can refer to http://en.wikipedia.org/wiki/Binomial_proportion_confidence_interval for more information. This indicates how significant the ratio is.
This section describes how we can use Cython with NumPy. To demonstrate this, perform the following steps:
Let's write a .pyx
file that contains a function to calculate the ratio of up days and associated confidence. First, this function computes the differences of the prices. Then, we count the number of positive differences, giving us a ratio for the proportion of up days. Finally, we apply the formula for the confidence from the Wikipedia page in the introduction.
import numpy def pos_confidence...
We can call C functions from Cython. For instance, in this example, we will call the C log
function. This function works on a single number only. Remember that the NumPy
log
function can also work with arrays. We will compute the so-called log returns of stock prices.
We will start by writing some Cython code:
First, we need to import the C log
function from the libc
namespace. Second, we will apply this function to numbers in a for
loop. Finally, we will use the NumPy
diff
function to get the first order difference between the log values in the second step.
from libc.math cimport log import numpy def logrets(numbers): logs = [log(x) for x in numbers] return numpy.diff(logs)
Building has been covered in the previous recipes already. We only need to change some values in the setup.py
file.
Let's download stock price data with matplotlib, again. Apply the Cython logrets
function that we just created on the...
We will profile Cython and NumPy code that tries to approximate the Euler constant. You can refer to http://en.wikipedia.org/wiki/E_%28mathematical_constant%29 for the required formula.
This section demonstrates how to profile Cython code. To do this, go through the following steps:
NumPy approximation of e: For the NumPy approximation of e perform the following steps:
First, we will create an array of 1 to n (n is 40 in our example).
Then, we will compute the cumulative product of this array, which happens to be the factorial.
After that, we take the reciprocal of the factorials.
Finally, we apply the formula from the Wikipedia page. At the end, we put standard profiling code, giving us the following program:
import numpy import cProfile import pstats def approx_e(n=40): # array of [1, 2, ... n-1] arr = numpy.arange(1, n) # calculate the factorials and convert to floats arr = arr.cumprod().astype(float) # reciprocal 1/n arr = numpy.reciprocal...
The last example is about approximating factorials with Cython. We will use two approximation methods. First, we will use the Stirling approximation method (see http://en.wikipedia.org/wiki/Stirling%27s_approximation for more information). The formula for the Stirling approximation is:
Secondly, we will be using the approximation due to Ramanujan, with the following formula:
This section describes how to approximate factorials using Cython. In this recipe, we will be using types, which as you may remember, is optional in Cython. In theory, declaring static types should speed things up. Static typing offers interesting challenges that you may not encounter when writing Python code, but don't worry, we will try to keep it simple.