Defining data integration
Data integration is the process of combining data from multiple sources to assist businesses in gaining insights and making educated decisions. In the age of big data, businesses generate vast volumes of structured and unstructured data regularly. To properly appreciate the value of this information, it must be incorporated in a format that enables efficient analysis and interpretation.
Take the example of extract, transform, and load (ETL) processing, which consists of multiple stages, including data extraction, transformation, and loading. Extraction entails gathering data from various sources, such as databases, data lakes, APIs, or flat files. Transformation involves cleaning, enriching, and transforming the extracted data into a standardized format, making it easier to combine and analyze. Finally, loading refers to transferring the transformed data into a target system, such as a data warehouse, where it can be stored, accessed, and analyzed by relevant stakeholders.
The data integration process not only involves handling different data types, formats, and sources, but also requires addressing challenges such as data quality, consistency, and security. Moreover, data integration must be scalable and flexible to accommodate the constantly changing data landscape. The following figure depicts the scope for data integration.
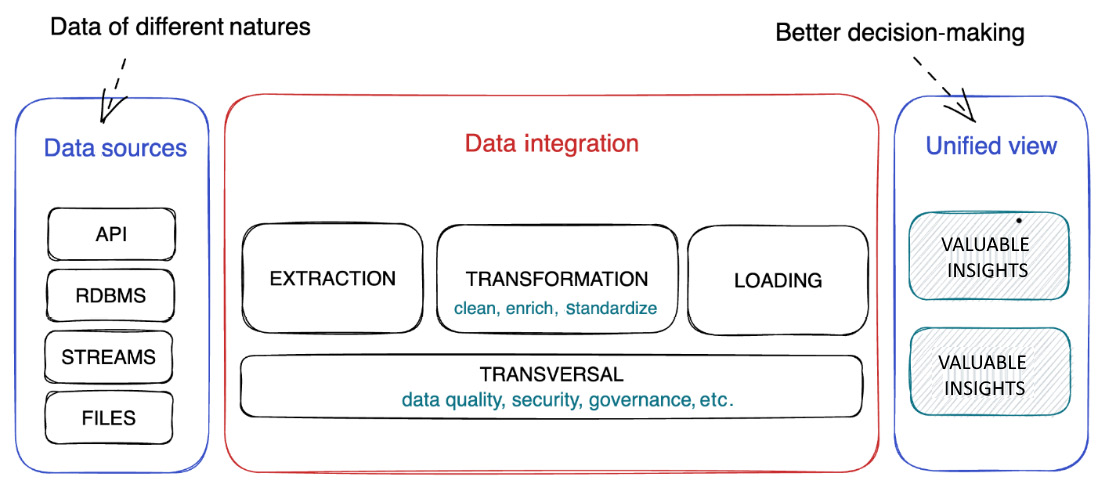
Figure 2.1 – Scope for data integration
Understanding data integration as a process is critical for businesses to harness the power of their data effectively.
Warning
Data integration should not be confused with data ingestion, which is the process of moving and replicating data from various sources and loading it into the first step of the data layer with minimal transformation. Data ingestion is a necessary but not sufficient step for data integration, which involves additional tasks such as data cleansing, enrichment, and transformation.
A well-designed and well-executed data integration strategy can help organizations break down data silos, streamline data management, and derive valuable insights for better decision-making.
The importance of data integration in modern data-driven businesses
Data integration is critical in today’s data-driven enterprises and cannot be understated. As organizations rely more on data to guide their decisions, operations, and goals, the ability to connect disparate data sources becomes increasingly important. The following principles emphasize the importance of data integration in today’s data-driven enterprises.
Organization and resources
Data integration is critical in today’s competitive business market for firms trying to leverage the power of their data and make educated decisions. Breaking down data silos is an important part of this process since disconnected and unavailable data can prevent cooperation, productivity, and the capacity to derive valuable insights. Data silos often arise when different departments or teams within an organization store their data separately, leading to a lack of cohesive understanding and analysis of the available information. Data integration tackles this issue by bringing data from several sources together in a centralized area, allowing for smooth access and analysis across the enterprise. This not only encourages greater team communication and collaboration but also builds a data-driven culture, which has the potential to greatly improve overall business performance.
Another aspect of data integration is streamlining data management, which simplifies data handling processes and eliminates the need to manually merge data from multiple sources. By automating these processes, data integration reduces the risk of errors, inconsistencies, and duplication, ensuring that stakeholders have access to accurate and up-to-date information, which allows organizations to make more informed decisions and allocate resources more effectively.
One additional benefit of data integration is the ability to acquire useful insights in real time from streaming sources such as Internet of Things (IoT) devices and social media platforms. As a result, organizations may react more quickly and efficiently to changing market conditions, consumer wants, and operational issues. Real-time data can also assist firms in identifying trends and patterns, allowing them to make proactive decisions and remain competitive.
For a world of trustworthy data
Taking into consideration the importance of a good decision for the company, it is important to enhance customer experiences by integrating data from various customer touchpoints. In this way, businesses can gain a 360-degree view of their customers, allowing them to deliver personalized experiences and targeted marketing campaigns. This can lead to increased customer satisfaction, revenue, and loyalty.
In the same way, quality improvement involves cleaning, enriching, and standardizing data, which can significantly improve its quality. High-quality data is essential for accurate and reliable analysis, leading to better business outcomes.
Finally, it is necessary to take into consideration the aspects of governance and compliance with the laws. Data integration helps organizations maintain compliance with data protection regulations, such as the General Data Protection Regulation (GDPR) and California Consumer Privacy Act (CCPA). By consolidating data in a centralized location, businesses can more effectively track, monitor, and control access to sensitive information.
Strategic decision-making solutions
Effective data integration enables businesses to gain a comprehensive view of their data, which is needed for informed decision-making. By combining data from various sources, organizations can uncover hidden patterns, trends, and insights that would have been difficult to identify otherwise.
Furthermore, with data integration, you allow organizations to combine data from different sources, enabling the discovery of new insights and fostering innovation.
The following figure depicts the position of data integration in modern business.
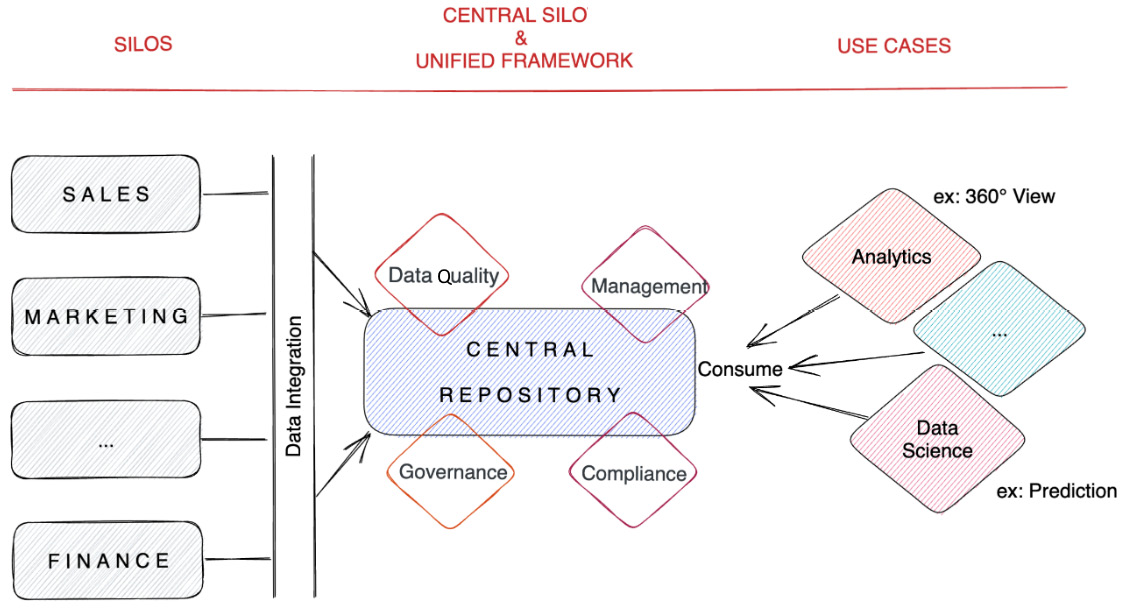
Figure 2.2 – The position of data integration in modern business
Companies can leverage these insights to develop new products, services, and business models, driving growth and competitive advantage.
Differentiating data integration from other data management practices
The topics surrounding data are quite vast, and it is very easy to get lost in this ecosystem. We will attempt to clarify some of the terms currently used that may or may not be a part of data integration for you:
- Data warehousing: Data warehousing refers to the process of collecting, storing, and managing large volumes of data from various sources in a centralized repository. Although data integration is a critical component of building a data warehouse, the latter involves additional tasks such as data modeling, indexing, and query optimization to enable efficient data retrieval and analysis.
- Data migration: Data migration is the process of transferring data from one system or storage location to another, usually during system upgrades or consolidation. While data integration may involve some data migration tasks, such as data transformation and cleansing, the primary goal of data migration is to move data without altering its structure or content fundamentally.
- Data virtualization: Data virtualization is an approach to data management that allows organizations to access, aggregate, and manipulate data from different sources without the need for physical data movement or storage. This method provides a unified, real-time view of data, enabling users to make better-informed decisions without the complexities of traditional data integration techniques.
- Data federation: Data federation, a subset of data virtualization, is a technique that offers a unified view of data from multiple sources without the need to physically move or store the data in a central repository. Primarily, it involves the virtualization of autonomous data stores into a larger singular data store, with a frequent focus on relational data stores. This contrasts with data virtualization, which is more versatile, as it can work with various types of data ranging from RDBMS to NoSQL.
- Data synchronization: Data synchronization is the process of maintaining consistency and accuracy across multiple copies of data stored in different locations or systems. Data synchronization ensures that changes made to one data source are automatically reflected in all other copies. While data integration may involve some synchronization tasks, its primary focus is on combining data from multiple sources to create a unified view.
- Data quality management: Data quality management is the practice of maintaining and improving the accuracy, consistency, and reliability of data throughout its life cycle. Data quality management involves data cleansing, deduplication, validation, and enrichment. Although data quality is a crucial aspect of data integration, it is a broader concept that encompasses several other data management practices.
- Data vault: Data vault modeling is an approach to designing enterprise data warehouses, introduced by Dan Linstedt. It is a detail-oriented hybrid data modeling technique that combines the best aspects of third normal form (3NF), which we will cover in Chapter 4, Data Sources and Types, dimensional modeling, and other design principles. The primary focus of data vault modeling is to create a flexible, scalable, and adaptable data architecture that can accommodate rapidly changing business requirements and easily integrate new data sources.
By differentiating data integration from these related data management practices, we can better understand its unique role in the modern data stack. Data integration is vital for businesses to derive valuable insights from diverse data sources, ensuring that information is accurate, up to date, and readily accessible for decision-making.
Challenges faced in data integration
Data integration is a complex process that requires enterprises and data services to tackle various challenges to effectively combine data from multiple sources and create a unified view.
Technical challenges
As an organization’s size increases, so does the variety and volume of data, resulting in greater technical complexity. Addressing this challenge requires a comprehensive approach to ensure seamless integration across all data types:
- Data heterogeneity: Data comes in various formats, structures, and types, which can make integrating it difficult. Combining structured data, such as that from relational databases, with unstructured data, such as text documents or social media posts, requires advanced data transformation techniques to create a unified view.
- Data volume: The sheer volume of data that enterprises and data services deal with today can be overwhelming. Large-scale data integration projects involving terabytes or petabytes of data require scalable and efficient data integration techniques and tools to handle such volumes without compromising performance.
- Data latency: For businesses to make timely choices, real-time or near-real-time data integration is becoming essential. Integrating data from numerous sources with low latency, on the other hand, can be difficult, especially when dealing with enormous amounts of data. To reduce latency and provide quick access to integrated data, data services must use real-time data integration methodologies and technologies.
Industry good practice
To overcome technical challenges such as data heterogeneity, volume, and latency, organizations can leverage cloud-based technologies that offer scalability, flexibility, and speed. Cloud-based solutions can also reduce infrastructure costs and maintenance efforts, allowing organizations to focus on their core business processes.
Integrity challenges
Once data capture is implemented, preferably during the setup process, maintaining data integrity becomes important to ensure accurate decision-making based on reliable indicators. Additionally, it’s essential to guarantee that the right individuals have access to the appropriate data:
- Data quality: Ensuring data quality is a significant challenge during data integration. Poor data quality, such as missing, duplicate, or inconsistent data, can negatively impact the insights derived from the integrated dataset. Enterprises must implement data cleansing, validation, and enrichment techniques to maintain and improve data quality throughout the integration process.
- Data security and privacy: Ensuring data security and privacy is a critical concern during data integration. Enterprises must comply with data protection regulations, such as GDPR or the Health Insurance Portability and Accountability Act (HIPAA), while integrating sensitive information. This challenge requires implementing data encryption, access control mechanisms, and data anonymization techniques to protect sensitive data during the integration process.
- Master data management (MDM): Implementing MDM is crucial to ensure consistency, accuracy, and accountability in non-transactional data entities such as customers, products, and vendors. MDM helps in creating a single source of truth, reducing data duplication, and ensuring data accuracy across different systems and databases during data integration. MDM strategies also aid in aligning various data models from different sources, ensuring that all integrated systems use a consistent set of master data, which is vital for effective data analysis and decision-making.
- Referential integrity: Maintaining referential integrity involves ensuring that relationships among data in different databases are preserved and remain consistent during and after integration. This includes making sure that foreign keys accurately and reliably point to primary keys in related tables. Implementing referential integrity controls is essential to avoid data anomalies and integrity issues, such as orphaned records or inconsistent data references, which can lead to inaccurate data analytics and business intelligence outcomes.
Note
Data quality is a crucial aspect of data integration, as poor data quality can negatively impact the insights derived from the integrated dataset. Organizations should implement data quality tools and techniques to ensure that their data is accurate, complete, and consistent throughout the integration process.
Knowledge challenges
Implementing and sustaining a comprehensive data integration platform requires the establishment, accumulation, and preservation of knowledge and skills over time:
- Integration complexity: Integrating data from various sources, systems, and technologies can be a substantial task. To streamline and decrease complexity, businesses must use strong data integration tools and platforms that handle multiple data sources and integration protocols.
- Resource constraints: Data integration initiatives frequently necessitate the use of expert data engineers and architects, as well as specific tools and infrastructure. Enterprises may have resource restrictions, such as a shortage of experienced staff, budget limits, or insufficient infrastructure, which can hinder data integration initiatives.
Enterprises may establish effective data integration strategies and realize the full potential of their data assets by understanding and tackling these problems. Implementing strong data integration processes will allow firms to gain useful insights and make better decisions.
Tip
To address knowledge challenges such as integration complexity and resource constraints, organizations can use user-friendly and collaborative tools that simplify the design and execution of data integration workflows. These tools can also help reduce the dependency on expert staff and enable non-technical users to access and use data as needed.