Python for Data Wrangling
There is always a debate regarding whether to perform the wrangling process using an enterprise tool or a programming language and its associated frameworks. There are many commercial, enterprise-level tools for data formatting and preprocessing that do not involve much coding on the user's part. Some of these examples include the following:
- General-purpose data analysis platforms, such as Microsoft Excel (with add-ins)
- Statistical discovery package, such as JMP (from SAS)
- Modeling platforms, such as RapidMiner
- Analytics platforms from niche players that focus on data wrangling, such as Trifacta, Paxata, and Alteryx
However, programming languages such as Python and R provide more flexibility, control, and power compared to these off-the-shelf tools. This also explains their tremendous popularity in the data science domain:
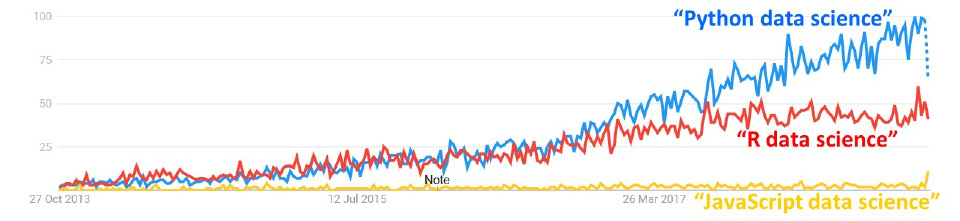
Figure 1.2: Google trends worldwide over the last 5 years
Furthermore, as the volume, velocity, and variety (the three Vs of big data) of data undergo rapid changes, it is always a good idea to develop and nurture a significant amount of in-house expertise in data wrangling using fundamental programming frameworks so that an organization is not beholden to the whims and fancies of any particular enterprise platform for as basic a task as data wrangling.
A few of the obvious advantages of using an open source, free programming paradigm for data wrangling are as follows:
- A general-purpose open-source paradigm puts no restrictions on any of the methods you can develop for the specific problem at hand.
- There's a great ecosystem of fast, optimized, open-source libraries, focused on data analytics.
- There's also growing support for connecting Python to every conceivable data source type.
- There's an easy interface to basic statistical testing and quick visualization libraries to check data quality.
- And there's a seamless interface of the data wrangling output with advanced machine learning models.
Python is the most popular language for machine learning and artificial intelligence these days. Let's take a look at a few data structures in Python.