Chapter 7: Neural Style Transfer
In the previous chapter, we started exploring generative models using PyTorch. We built machine learning models that can generate text and music by training the models without supervision on text and music data, respectively. We will continue exploring generative modeling in this chapter by applying a similar methodology to image data.
We will mix different aspects of two different images, A and B, to generate a resultant image, C, that contains the content of image A and the style of image B. This task is also popularly known as neural style transfer because, in a way, we are transferring the style of image B to image A in order to achieve image C, as illustrated in the following figure:
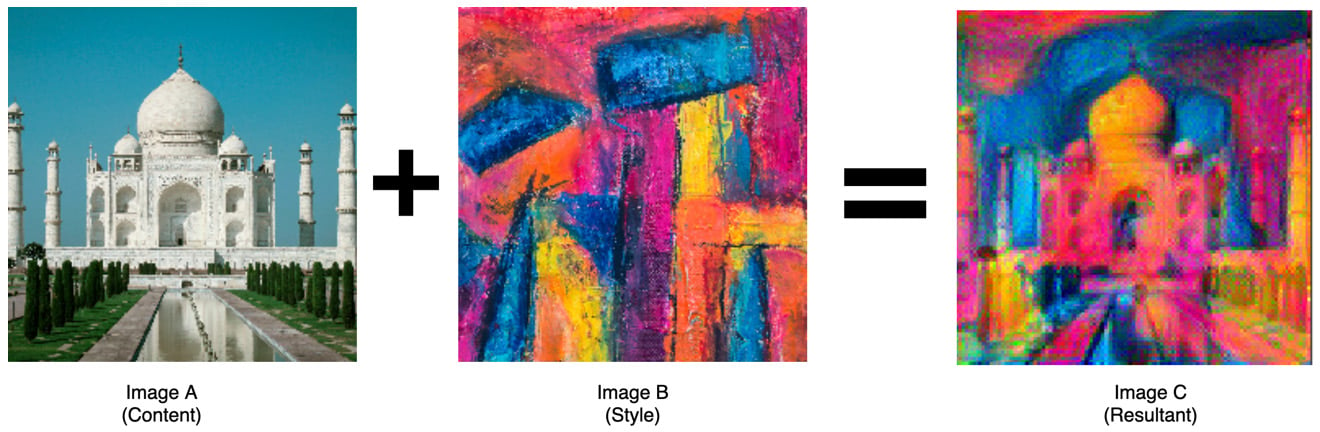
Figure 7.1 – Neural style transfer example
First, we will briefly discuss how to approach this problem and understand the idea behind achieving style transfer. Using PyTorch, we will then implement our own neural style transfer...