So far, we have learned about how reinforcement learning (RL) works. In the upcoming chapters, we will learn about Deep reinforcement learning (DRL), which is a combination of deep learning and RL. DRL is creating a lot of buzz around the RL community and is making a serious impact on solving many RL tasks. To understand DRL, we need to have a strong foundation in deep learning. Deep learning is actually a subset of machine learning and it is all about neural networks. Deep learning has been around for a decade, but the reason it is so popular right now is because of the computational advancements and availability of a huge volume of data. With this huge volume of data, deep learning algorithms will outperform all classic machine learning algorithms. Therefore, in this chapter, we will learn about several deep learning algorithms like recurrent neural...
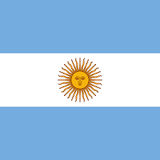
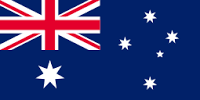
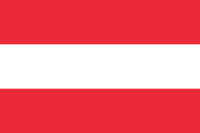
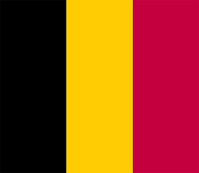
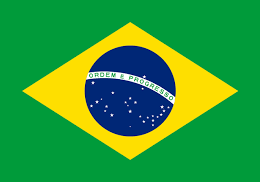
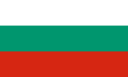
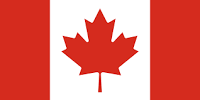
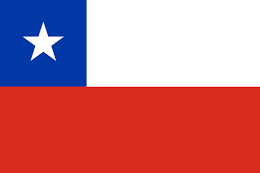
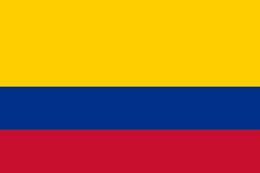
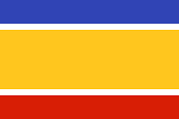
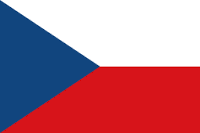
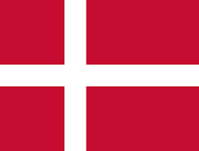
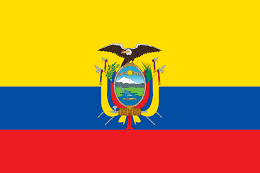
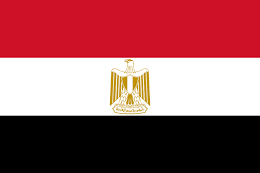
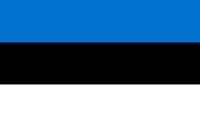
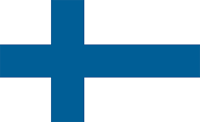
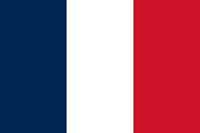
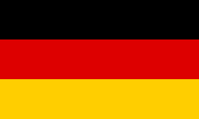
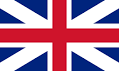
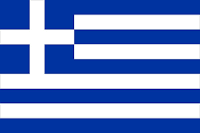
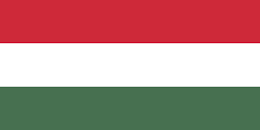
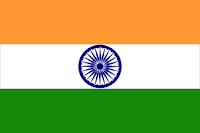
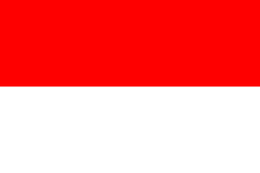
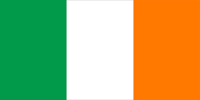
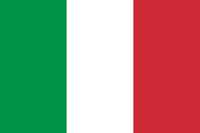
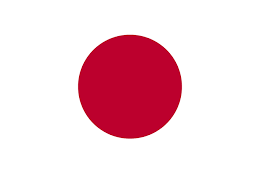
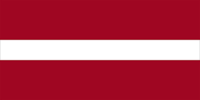
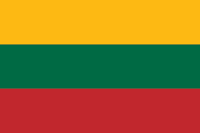
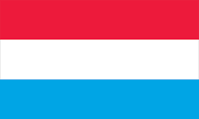
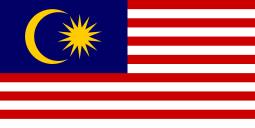
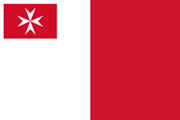
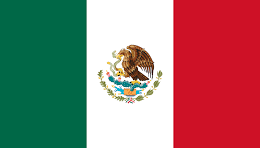
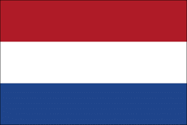
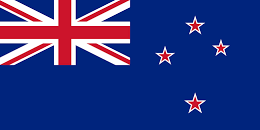
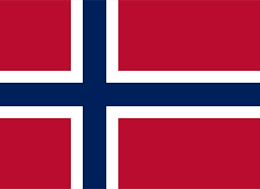
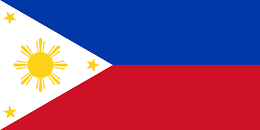
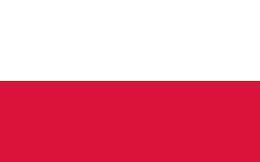
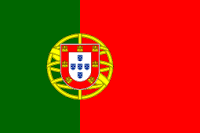
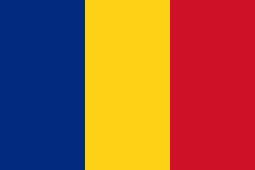
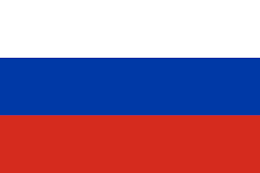

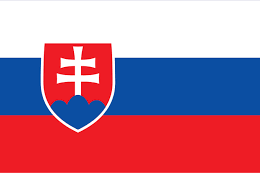
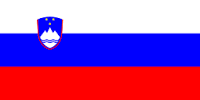
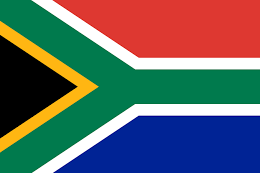
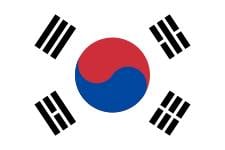
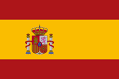
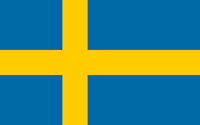
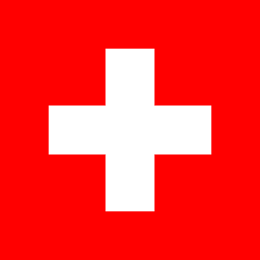
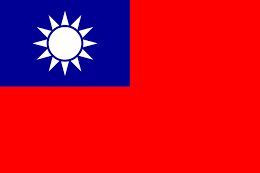
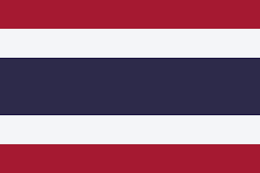
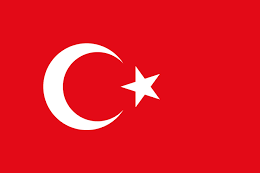
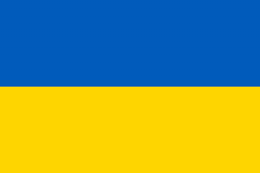
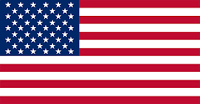