We discussed in the last two chapters about big data, Hadoop, and the Hadoop ecosystem. Now, let's discuss more technical aspects about Hadoop Architecture. Hadoop Architecture is extremely flexible, scalable, and fault tolerant. The key to the success of Hadoop is its architecture that allows the data to be loaded as it is and stored in a distributed way, which has no data loss and no preprocessing is required.
We know that Hadoop is distributed computing and a parallel processing environment. Hadoop architecture can be divided in two parts: storage and processing. Storage in Hadoop is handled by Hadoop Distributed File System (HDFS), and processing is handled by MapReduce, as shown in the following image:
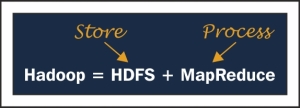
In this chapter, we will cover the basics of HDFS concept, Architecture, some key features, how Read and Write process happens, and some examples. MapReduce is the heart of Hadoop, and we will cover the Architecture, Serialization...