Introducing data wrangling
For organizations to become data-driven to provide value to customers or make more informed business decisions, they need to collect a lot of data from different data sources such as clickstreams, log data, transactional systems, and flat files and store them in different data stores such as data lakes, databases, and data warehouses as raw data. Once this data is stored in different data stores, it needs to be cleansed, transformed, organized, and joined from different data sources to provide more meaningful information to downstream applications such as machine learning models to provide product recommendations or look for traffic conditions. Alternatively, it can be used by business or data analytics to extract meaningful business information:
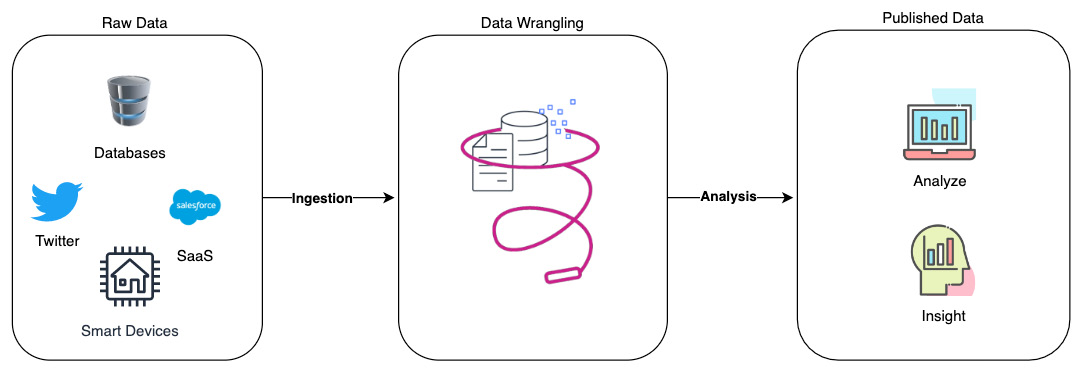
Figure 1.1: Data pipeline
The 80-20 rule of data analysis
When organizations collect data from different data sources, it is not of much use initially. It is estimated that data scientists spend about 80% of their time cleaning data. This means that only 20% of their time will be spent analyzing and creating insights from the data science process:
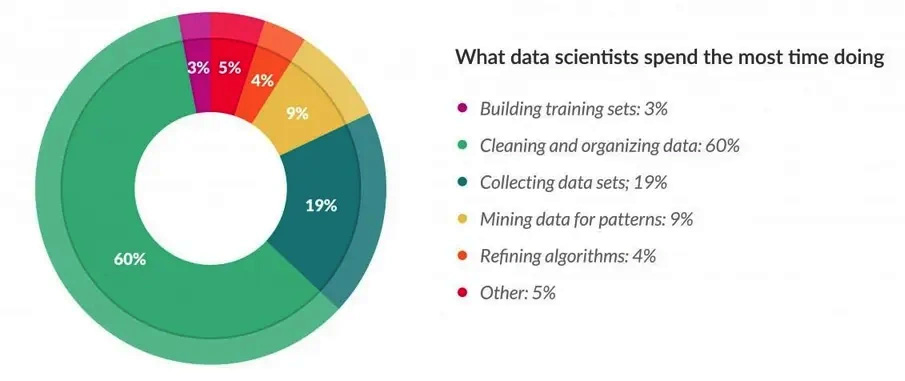
Figure 1.2: Work distribution of a data scientist
Now that we understand the basic concept of data wrangling, we’ll learn why it is essential, and the various benefits we get from it.
Advantages of data wrangling
If we go back to the analogy of oil, when we first extract it, it is in the form of crude oil, which is not of much use. To make it useful, it has to go through a refinery, where the crude oil is put in a distillation unit. In this distillation process, the liquids and vapors are separated into petroleum components called fractions according to their boiling points. Heavy fractions are on the bottom while light fractions are on the top, as seen here:
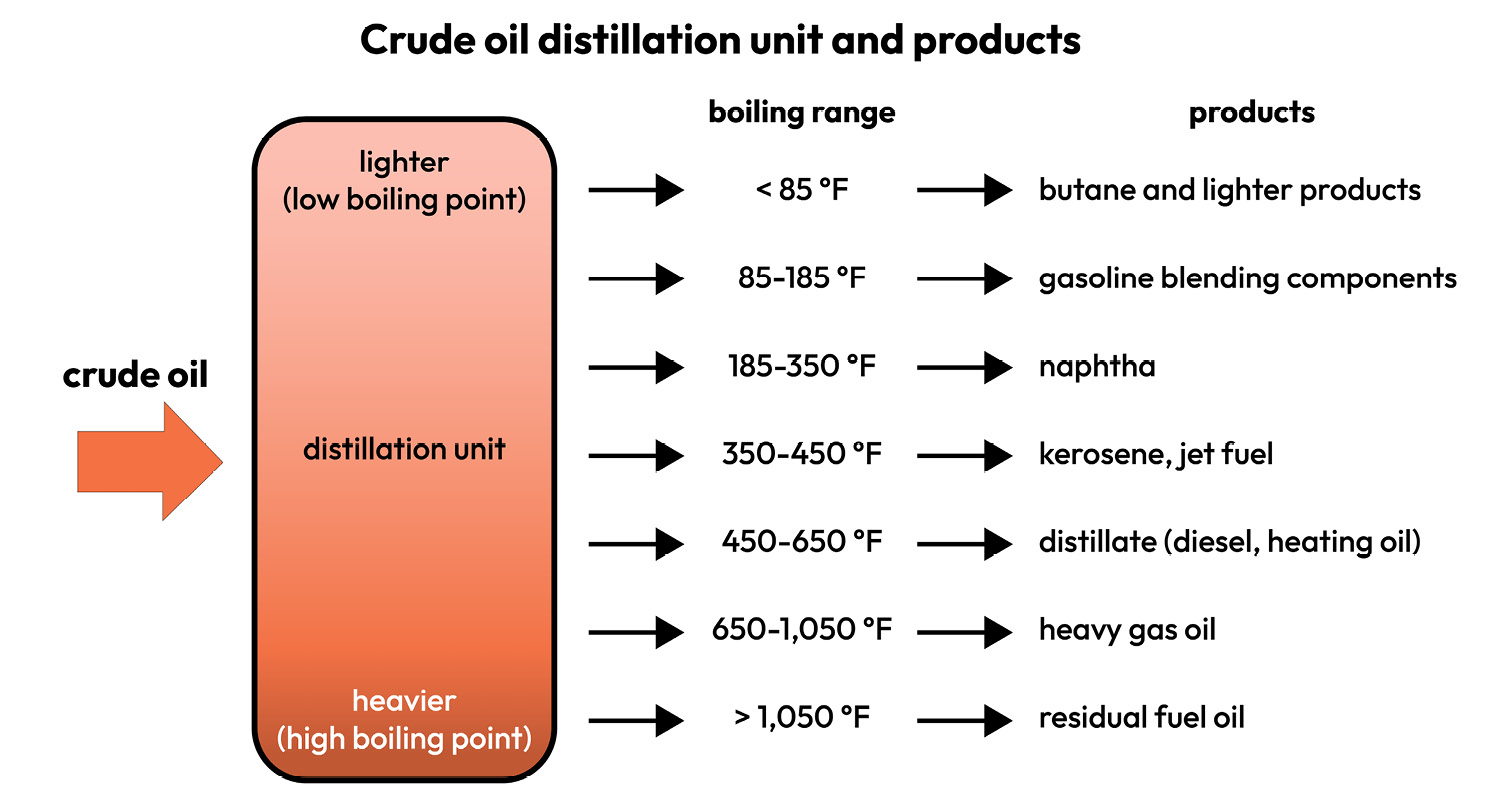
Figure 1.3: Crude oil processing
The following figure showcases how oil processing correlates to the data wrangling process:
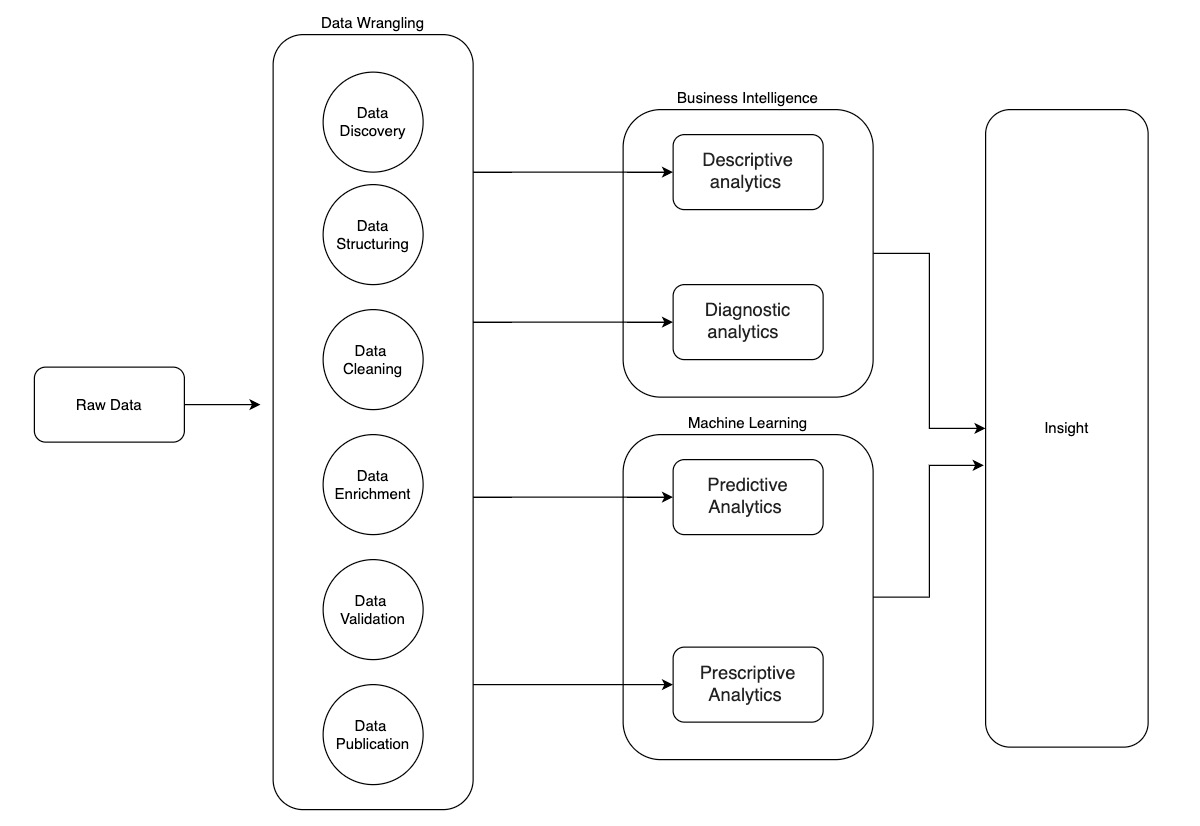
Figure 1.4: The data wrangling process
Data wrangling brings many advantages:
- Enhanced data quality: Data wrangling helps improve the overall quality of the data. It involves identifying and handling missing values, outliers, inconsistencies, and errors. By addressing these issues, data wrangling ensures that the data used for analysis is accurate and reliable, leading to more robust and trustworthy results.
- Improved data consistency: Raw data often comes from various sources or in different formats, resulting in inconsistencies in naming conventions, units of measurement, or data structure. Data wrangling allows you to standardize and harmonize the data, ensuring consistency across the dataset. Consistent data enables easier integration and comparison of information, facilitating effective analysis and interpretation.
- Increased data completeness: Incomplete data can pose challenges during analysis and modeling. Data wrangling methods allow you to handle missing data by applying techniques such as imputation, where missing values are estimated or filled in based on existing information. By dealing with missing data appropriately, data wrangling helps ensure a more complete dataset, reducing potential biases and improving the accuracy of analyses.
- Facilitates data integration: Organizations often have data spread across multiple systems and sources, making integration a complex task. Data wrangling helps in merging and integrating data from various sources, allowing analysts to work with a unified dataset. This integration facilitates a holistic view of the data, enabling comprehensive analyses and insights that might not be possible when working with fragmented data.
- Streamlined data transformation: Data wrangling provides the tools and techniques to transform raw data into a format suitable for analysis. This transformation includes tasks such as data normalization, aggregation, filtering, and reformatting. By streamlining these processes, data wrangling simplifies the data preparation stage, saving time and effort for analysts and enabling them to focus more on the actual analysis and interpret the results.
- Enables effective feature engineering: Feature engineering involves creating new derived variables or transforming existing variables to improve the performance of machine learning models. Data wrangling provides a foundation for feature engineering by preparing the data in a way that allows for meaningful transformations. By performing tasks such as scaling, encoding categorical variables, or creating interaction terms, data wrangling helps derive informative features that enhance the predictive power of models.
- Supports data exploration and visualization: Data wrangling often involves exploratory data analysis (EDA), where analysts gain insights and understand patterns in the data before formal modeling. By cleaning and preparing the data, data wrangling enables effective data exploration, helping analysts uncover relationships, identify trends, and visualize the data using charts, graphs, or other visual representations. These exploratory steps are crucial for forming hypotheses, making data-driven decisions, and communicating insights effectively.
Now that we have learned about the advantages of data wrangling, let’s understand the steps involved in the data wrangling process.