Analyzing the application
A common way to understand what happens in an application is to replay its course after it’s run. A good example would be a SQL application. When you query a SQL database, for instance, through a JDBC connector, you are creating access logs in the database. These logs may contain lots of information, especially regarding who has queried the database, what they queried, when it was executed, and sometimes information on how long it took to process the query, how many bytes were retrieved, and so on.
This situation is explained in Figure 3.4. Users are continuously querying a central SQL database. This creates a log file, which is a kind of journal that contains the records of the queries:
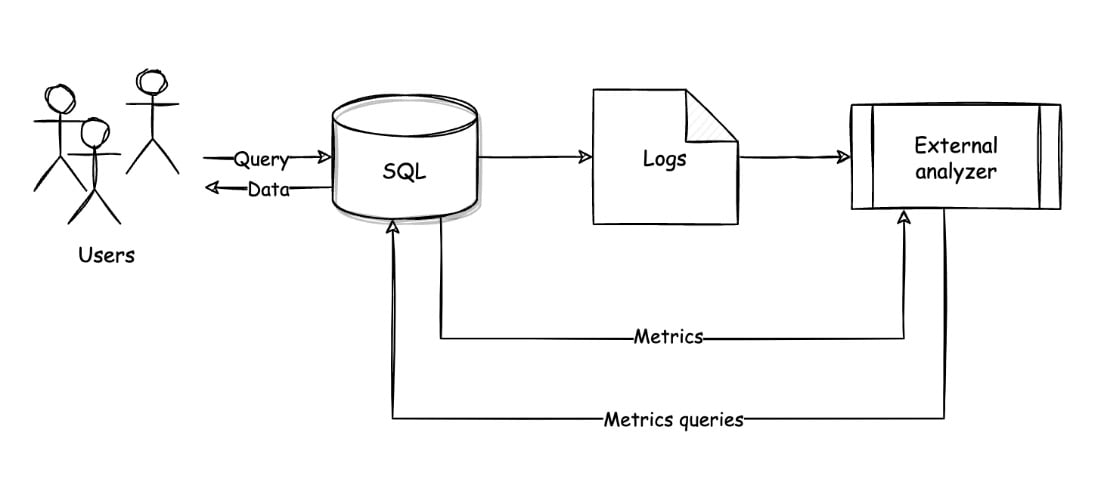
Figure 3.4 – Logging strategy for a SQL logs analyzer
This said, these logs can be extremely valuable for observability purposes. By using strategies to retrieve and analyze the logs, the data team can rebuild data transformation...