You're reading from Applied Deep Learning with Keras
Neural networks are the building blocks of all deep learning models. In traditional neural networks, all the inputs and outputs are independent. However, there are instances where a particular output is dependent on the previous output of the system. Consider the stock price of a company as an example – the output at the end of any given day is related to the output of the previous day. Similarly, in Natural Language Processing (NLP), the final words in a sentence are dependent on the previous words in the sentence. A special type of neural network, called a Recurrent Neural Network (RNN), is used to solve these types of problems where the network needs to remember previous outputs. This chapter introduces and explores the concepts and applications of RNNs. It also explains how RNNs are different from standard feedforward neural networks. You will also gain an understanding of what the vanishing gradient problem is and a Long-Short-Term-Memory (LSTM) network. This chapter also...
If we analyze the stock price of Apple for the past five months, as shown in Figure 9.1, we can see that there is a trend. To predict or forecast future stock prices, we need to gain an understanding of this trend and then do our mathematical computations while keeping this trend in mind:
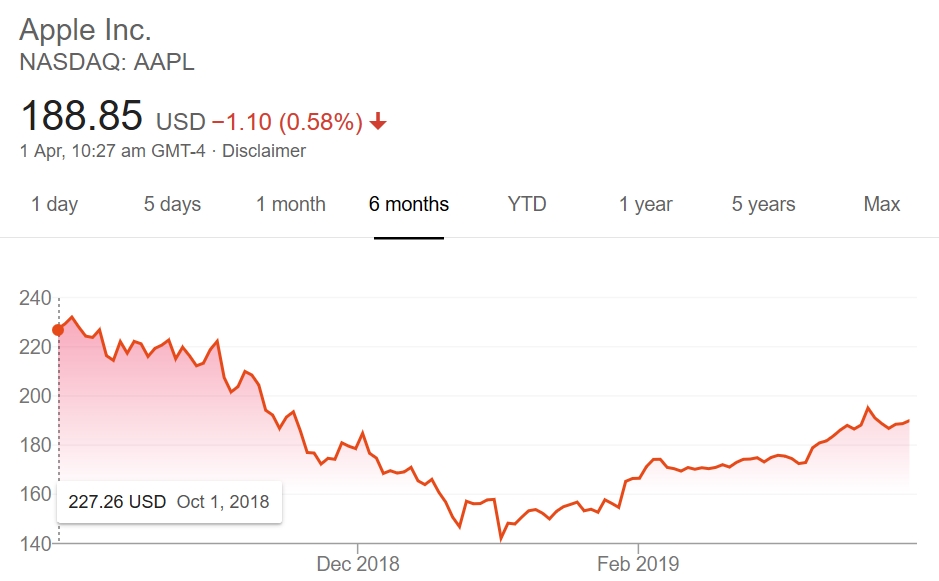
Figure 9.1: Apple's stock price over the last five months
This trend is deeply related to sequential memory and sequential modeling. If you have a model that can remember the previous outputs and then predict the next output based on the previous outputs, we say that the model has sequential memory.
The modeling that is done to process this sequential memory is known as sequential modeling. This is not only true for stock market data, but it is also true in NLP applications; we will look at one such example later, when we study RNNs.
RNNs are a class of neural networks that are built on the concept of sequential memory. Unlike in traditional neural networks, an RNN predicts the results in sequential data. Currently, an RNN is the most robust and powerful technique available for processing sequential data.
If you have access to a smartphone that has Google Assistant, try opening it and asking the question: "When was the United Nations formed?". The answer is displayed in the following screenshot:
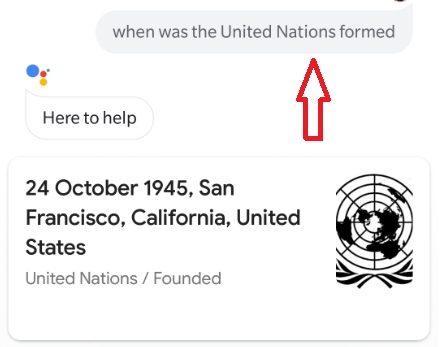
Figure 9.2: Google Assistant's output
Now ask a second question, "Why was it formed?", as follows:
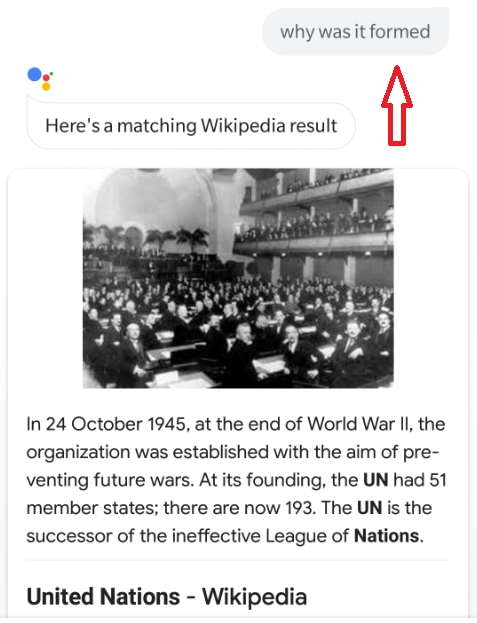
Figure 9.3: Google Assistant's contextual output
Now ask a third question, "Where are its headquarters?", and you should get the following answer:
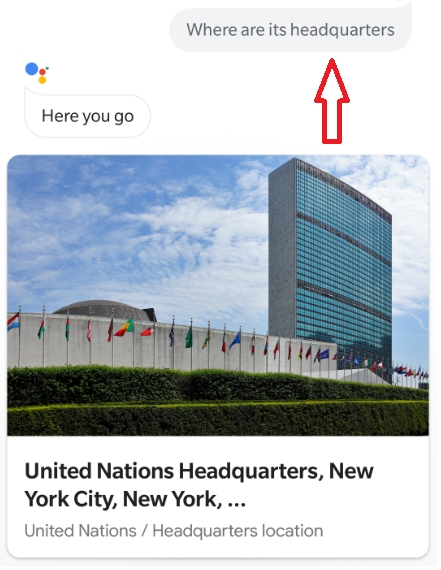
Figure 9.4: Google Assistant's output
One interesting thing to note here is that we only mentioned "United Nations" in the first question. In the second and third question, we simply asked the assistant why it was formed and where the headquarters were, respectively...
LSTMs are RNNs whose main objective is to overcome the shortcomings of the vanishing gradient and exploding gradient problem. The architecture is built such that they remember data and information for a long period of time.
LSTMs were designed to overcome the limitation of the vanishing and exploding gradient problems. LSTM networks are a special kind of RNN, which are capable of learning long-term dependencies. They are designed to avoid the long-term dependency problem; being able to remember information for long intervals of time is how they are wired. The following diagram displays a standard recurrent network where the repeating module has a tanh activation function. This is a simple RNN; in this architecture, we often have to face the vanishing gradient problem:
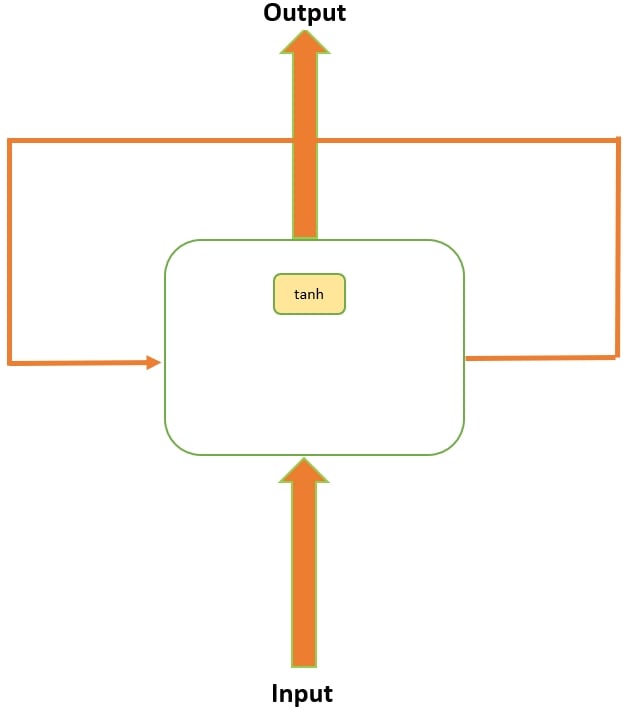
Figure 9.12: A simple RNN model
LSTM architecture is similar to simple RNNs but their repeating module has different components, as shown in the following diagram:
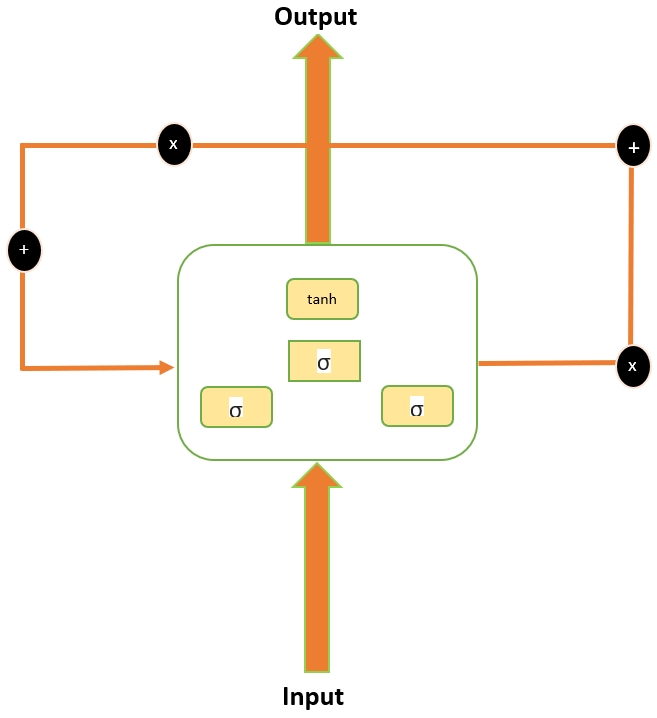
Figure 9.13: The LSTM model architecture...
In this chapter, we learned about sequential modeling and sequential memory by examining some real-life cases with Google Assistant. We further learned how sequential modeling is related to RNNs. We also learned how RNNs are different from traditional feedforward networks. We learned about the vanishing gradient problem in detail, and learned how using an LSTM is better than a simple RNN to overcome the vanishing gradient problem. We applied the learning to time series problems by predicting stock trends.
In this book, we learned the basics of machine learning and Python, while also gaining an in-depth understanding of applying Keras to develop efficient deep learning solutions. We understood the difference between machine and deep learning. We learned how to build a logistic regression model, first with scikit-learn, and then with Keras. We further explored Keras and its different models by creating prediction models for various real-world scenarios, such as disease prediction. Then...