Building and verifying code manually
Before GitLab CI/CD pipelines appeared, you needed to build and verify your code manually. This was often a terrible, soul-crushing experience, for reasons we’ll discuss here.
Building code manually
Building code depends on what language you use. If you use an interpreted language such as Python or Ruby, then building might not be necessary at all. But if you’re writing in a compiled language, you’d need to build your app by compiling its source code.
Imagine that you’re using Java. The following are just some of the different ways to compile Java source code into executable Java classes:
- You could use the
javac
Java compiler that ships with the Java Development Kit - You could use the Maven build tool
- You could use the Gradle build tool
There are lots of reasons that this manual build process is a tedious, annoying chore that most developers would happily leave behind:
- It’s subject to user error: how many times have you forgotten whether you need to point
javac
at the top-level package that your classes are in, or at the individual class files? - It’s slow, taking anywhere from a few seconds to several minutes, depending on how big your application is. That can add up to a lot of downtime.
- It’s easy to forget, causing confusion when you accidentally execute old code that doesn’t behave like you thought it would.
- Badly written code can fail to compile, causing everyone to waste time as the build engineer sends the code back to the developers for fixes, and waits for those fixes to arrive.
Verifying code manually
Once you’ve built your code, you need to verify that it’s working correctly. Testing takes countless shapes and forms, and there are more kinds of tests than we could describe in this book. But here are some of the most common forms that you may want to subject your code to:
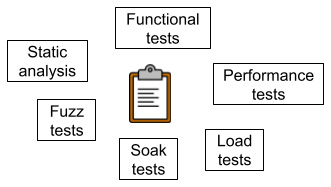
Figure 1.1 – Tests for verifying code
Functional tests
Does your program do what it’s supposed to? That’s the question that functional tests answer. Most programming projects begin with a specification that describes how the software should behave: given a certain input, what output should it provide? The developers are only done with their jobs when the code they write conforms to those specs. How do they know that their code conforms? That’s where functional tests come in.
Just like there are many forms of testing in general, there are many sub-categories of testing that, together, make up functional tests.
Happy path testing makes sure that the program works as expected when it’s fed common, valid input. For example, if you feed 2 + 2
into a calculator, it had better return 4
! Happy path tests seem like the most important kind of tests because they check behavior that users are most likely to run into when they use your software. But in fact, you can usually cover the most common use cases with just a few happy path tests. The tests that cover unusual or unexpected cases tend to be far more numerous.
Speaking of unusual cases, that’s where edge- case testing enters the scene. If you imagine a spectrum of input values, most values that users will input will fall in the middle of that spectrum. For example, calculator users are more likely to enter something such as 56 ÷ 209
(where these values are in the middle of the range of values the calculator will accept) than they are to enter 0 + 0
or 999,999 – 999,999
(since those values are at the edges of the range). Edge -case testing makes sure that input values at the far edges of the acceptable spectrum don’t break your software. Can you create a username that consists of a single letter? Can you order 9,999 copies of a book? Can you deposit 1 cent into a bank account? If the specifications say that your software should be able to handle these edge cases, you’d better make sure it really can!
If edge -case testing ensures that your software can handle an input value that’s right up against the edge of acceptable values, corner-case testing confirms that your software can handle two or more simultaneous edge cases. Think of it as turbocharged edge-case testing that challenges your software by placing it in even more uncomfortable (but still valid) situations. For example, does your banking app allow you to schedule a withdrawal for the smallest valid amount of currency at the farthest valid date in the future? There’s no need to limit corner-case testing to two input values: if your software accepts three or ten or 100 input values at a time, you’ll need to make sure it works when every input is pushed all the way to the extreme end of the range of values valid according to the specifications.
That handles cases where the software is given valid values. But do you also need to make sure it behaves correctly when it receives invalid values? Of course you do! This form of testing is sometimes called unhappy path testing and is usually a lot more fun for testers to perform since it’s more likely to reveal bugs. All software must gracefully handle unexpected, invalid, or malformed data, and you need tests to prove that it does so. To return to our earlier examples, you’ll need to make sure your calculator doesn’t crash when you ask it to divide a number by zero. You have to check that the banking app doesn’t accidentally give you a deposit when you ask to withdraw negative-6
dollars. And your currency conversion software should give a sensible error message when you ask about an exchange rate on February 31, 2020.
Since there are usually more ways to enter bad data than good data into an application, developers often concentrate on correctly processing expected data but fail to think through the types of unexpected, malformed, or out-of-range data that their users might enter. Programs need to anticipate and gracefully handle all sorts of data – both good and bad. Writing complete sets of both happy path and unhappy path tests is the best way to make sure that the developer has written code that behaves well no matter what data a user throws at it.
Those are some of the kinds of behavior that involve both valid and invalid data that tests can check for. But there’s another dimension that you can use to categorize tests: the size of the code chunk that a test targets.
In most cases, the smallest piece of code that a test can check is a single method or function. For example, you may want to test a function called alphabetize that takes any number of strings as input and returns those same strings, but now in alphabetical order. To test this function, you would probably use a kind of test called a unit test. It tests a single unit of code, where a unit in this case is a single function. You could have a collection of several unit tests that all cover that function, albeit in different ways:
- Some might cover happy paths. For example, they could pass the
dog
,cat
, andmouse
strings as input. - Some might cover edge or corner cases. For example, they could pass the function a single empty string, strings that consist only of digits, or strings that are already alphabetized.
- Some might cover unhappy paths. For example, they could pass the function an unexpected data type, such as booleans, instead of the expected data type of strings.
To verify the behavior of bigger pieces of code, you can use integration tests. These don’t look at single functions, but instead at how groups of functions interact with each other. For example, imagine that your currency conversion application has four functions:
-
get_input
, which takes input from the user in the form of a source currency, a source amount, and a target currency. -
convert
, which converts that amount of source currency into the correct amount of the target currency. -
print_output
, which tells the user how much target currency the conversion produces. main
, which is the main entry point to your app. This is the function that is called when your app is used. It calls the three other functions and passes the output of each function as input to the next.
To make sure these functions play nicely together – that is, to check if they integrate well – you need integration tests that call main
, as opposed to unit tests that call get_input
, convert
, and print_output
. This lets you test at a higher level of abstraction, which is to say a level that gets closer to how a real user would use your application. After all, a user isn’t going to call get_input
in isolation. Instead, they will call main
, which, in turn, will call the other three functions and coordinate passing values between them. It’s easy to write a function that works as expected on its own, but it’s harder to make a collection of functions cooperate to build a larger piece of logic. Integration tests spot this type of problem in a way that pure unit tests can’t.
Testers often think of various sorts of tests as forming a pyramid. According to this model, unit tests occupy the wide, low base of the pyramid: they are low-level in the sense that they test fundamental pieces of code, and there are many of them. Integration tests occupy the middle of the pyramid: they operate at a higher level of abstraction than unit tests, and there are fewer of them. At the top of the pyramid is a third category of tests, which we’ll talk about next – user tests:
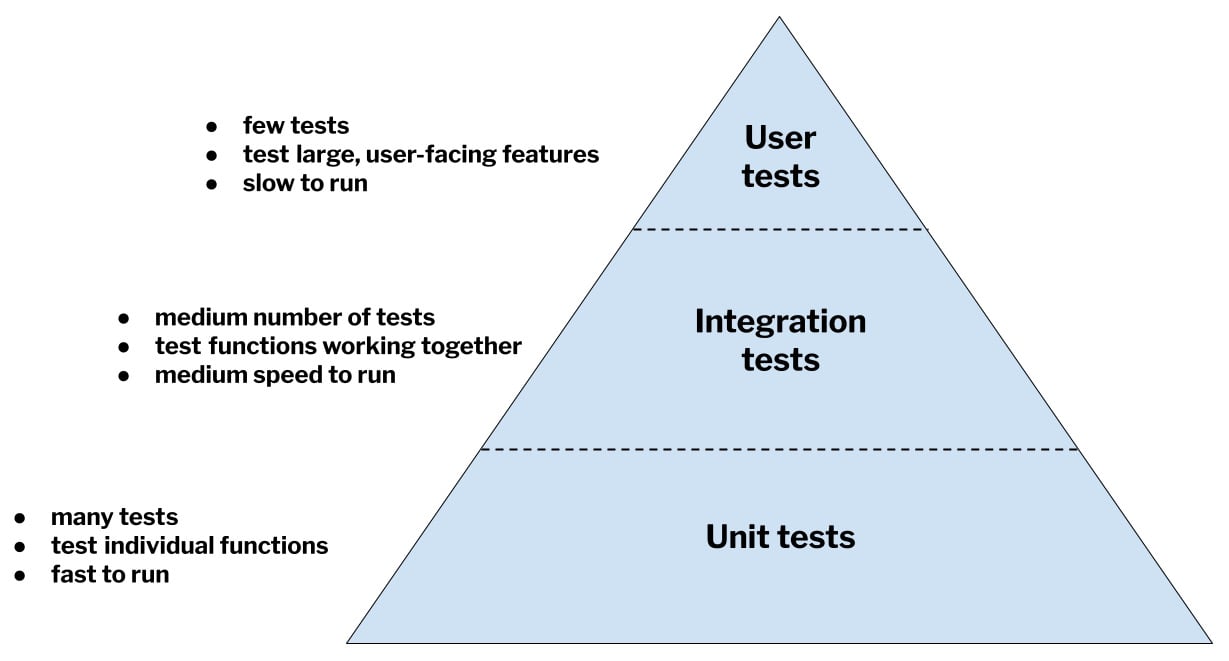
Figure 1.2 – The test pyramid
The final type of test, user tests, simulate a user’s behavior and exercise the software the same way a user would. For example, if users interact with a foreign exchange app by entering a source currency, an amount of that source currency, and a target currency, and then expect to see the output in the form of an amount of the target currency, then that’s exactly what a user test will do. This might mean that it uses the app’s GUI by clicking on buttons and entering values in fields. Or it might mean that it calls the app’s REST API endpoints, passing in input values and inspecting the result for the output value. However, it interacts with the application and does so in a fashion that’s as similar to a real user as possible. As with unit and integration tests, user tests can include happy path tests, edge- and corner-case tests, and unhappy path tests to cover all the scenarios that the software’s specifications describe, as well as any other scenarios that the test designer can concoct.
So far, we’ve explained the different purposes of unit, integration, and user tests, but we haven’t described another fundamental difference. Unit and integration tests are almost always automated. That is, they are computer programs that test other computer programs. While user tests are automated whenever possible, there are enough difficulties with writing reliable, reproducible tests that interact with an application’s GUI that many user tests must be run manually instead. Web applications are notoriously hard to test because of unpredictable behavior around load times, incomplete page rendering, missing or incompletely loaded CSS files, and network congestion. This means that while software development teams often attempt to automate user tests of web applications, more often than not, they end up with a hybrid of automated and manual user tests. As you may have guessed, manual user tests are extraordinarily expensive to run, both in terms of time and tester morale.
Performance tests
After that high-level tour of functional testing, you may be thinking that we’ve covered all the testing bases. But we’re just getting started. Another aspect of your application that should probably be tested is its performance: does it do what it’s supposed to do quickly enough to keep users from getting frustrated? Does it meet performance specifications the developers may have been given before they started coding? Is its performance significantly better or worse than the performance of its competitors? These are some of the questions that performance tests are designed to answer.
Performance testing is notoriously difficult to design and carry out. There are so many variables to consider when gauging how quickly your application runs:
- What environment should it be running in during the tests? Creating an environment identical to the production environment is often prohibitively expensive, but what corners can you cut in the test environment that won’t skew the results of performance tests too badly?
- What input values should your performance tests use? Depending on the application, some input values may take significantly longer to process than others.
- If your application is configurable, what configuration settings should you use? This is especially important if there is no standard configuration that most users settle on.
Even if you can figure out how to design useful performance tests, they often take a long time to run and, in some cases, produce inconsistent results. This leads teams to rerun performance tests frequently, which causes them to take even more time. So, performance tests are among the most critical, and also more expensive, of all the test types.
Load tests
Performance tests have a close cousin called load tests. Whereas performance tests determine how quickly your software can perform one operation (a single currency conversion, a single bank deposit, or a single arithmetic problem, for example), load tests determine how well your application handles many users interacting with it at the same time. Load tests suffer from many of the same design difficulties as performance tests and can produce similarly inconsistent results. They can be even more expensive to set up since they need a way to simulate hundreds or thousands of users.
Soak tests
As your application runs for hours or days, does it allocate memory that it never reclaims? Does it consume huge amounts of disk space with overzealous logging? Does it launch background processes that it never shuts down? If it suffers from any of these resource “leaks,” it could lose performance or even crash as it runs low on memory, disk space, or dedicated CPU cycles. These problems can be found with soak tests, which simply exercise your software over an extended period while monitoring its stability and performance. It’s probably obvious that soak tests are extraordinarily expensive in terms of time and hardware resources to run and monitor.
Fuzz tests
An underutilized but powerful form of testing is called fuzz testing. This approach sends valid but strange input data into your software to expose bugs that traditional functional tests may have missed. Think of it as happy path testing while drunk. So, instead of trying to create an account with the username “Sam,” try a username that consists of 1,000 letters. Or try to create a username that is entirely spaces. Or include Klingon alphabet Unicode characters in a shipping address.
Fuzz testing introduces a strong element of randomness: the input values it sends to your software are either completely randomly generated or are random permutations of input values that are known to be unproblematic for your code. For example, if your code translates PDF files into HTML files, a fuzz test may start by sending slightly tweaked versions of valid, easily handled PDF files, and then progress to asking your software to convert purely random strings that bear no resemblance to PDF files at all. Because fuzz testing can send many thousands of random input values before it stumbles on an input value that causes a crash or other bug, fuzz tests must be automated. They are simply too cumbersome to run manually.
Static code analysis
Another strictly automated form of testing is static code analysis. Whereas the other tests we’ve discussed try to find problems in your code as it runs, static code analysis inspects your source code without executing it. It can look for a variety of different problems, but in general, it checks to make sure you’re conforming to recognized coding best practices and language idioms. These could be established by your team, by the developers of the language itself, or by other programming authorities.
For example, static code analysis could notice that you declare a variable without ever assigning a value to it. Or it could point out that you’ve assigned a value to a variable but then never refer to that variable. It can identify unreachable code, code that uses coding patterns known to be slower than alternative but functionally equivalent patterns, or code that uses whitespace in unorthodox ways. These are all practices that may not cause your code to break exactly but could keep your code from being as readable, maintainable, or speedy as it could have been.
More challenges of verifying code
So far, we’ve described just some of the ways that you may want to verify the behavior, performance, and quality of your code. But once you’ve finished running all these different types of tests, you face the potentially difficult question of how to parse, process, and report the results. If you’re lucky, your test tools will generate reports in a standard format that you can integrate into an automatically updated dashboard. But you’ll likely find yourself using at least one test tool or framework that can’t be shoehorned into your normal reporting structure, and which needs to be manually scanned, cleaned, and massaged into a format that’s easy to read and disseminate.
We’ve already mentioned how performance tests in particular often need to be run repeatedly. But in fact, all of these types of tests need to be run repeatedly to catch regressions or smooth out so-called “flickering” tests, which are tests that sometimes pass and sometimes fail, depending on network conditions, server loads, or countless other unpredictable factors. This means that the burden of either manually running tests, or managing and triggering automated tests, is far greater than it appears at first. If you’re going to run tests repeatedly, you need to figure out when and how often to do so, you need to make sure the right hardware or test environments are available at the right times, and you need to be flexible enough to change your testing cadence when conditions change, or management asks for more up-to-date results. The point is that testing is tough, time-consuming, and error-prone, and all these difficulties are exaggerated every time humans need to get involved with making sure the tests happen in the right way at the right time.
Even though we’ve just said that tests should typically be run and then repeatedly rerun, there’s another countervailing force at play. Because executing tests is expensive and difficult, there’s a tendency to want to run them as infrequently as possible. This tendency is encouraged by a common development model that has developers building a feature (or sometimes an entire product) and then throwing the code over the wall to the Quality Assurance (QA) team for validation. This strict division between building the code and testing the code means that on many teams, tests are only run at the end of the development cycle – whether that’s at the end of a two-week sprint, the end of a year-long project, or somewhere in-between.
The practice of infrequent or delayed testing leads to an enormous problem: when the developers turn over a huge batch of code for testing – thousands or tens of thousands of lines of code that had been developed by different people using different coding styles and idioms over weeks or months – it can be extremely hard to diagnose the root cause of any bugs that the tests unearth. This, in turn, means that it’s hard to fix those bugs. Just like big haystacks hide needles more effectively than small haystacks, large batches of code make it hard to find, understand, and correct any bugs that they contain. The longer a development team waits before passing code on to the QA team, the bigger this problem becomes.
This concludes our lightning-fast survey of functional tests, load tests, soak tests, fuzz tests, and static code analysis. In addition, we explained some of the hidden difficulties involved with running all of these different sorts of tests. You might be wondering why we’ve discussed testing at all. The reason is that understanding the challenges of testing – getting a feel for how many ways there are to verify your code, how important the different forms of tests are, how time-consuming it is to set up test environments, how much of a hassle it is to manually run non-automatable user tests, how tricky it can be to process and report the results, and how tough it can be to find and fix bugs that are lurking within a huge bundle of code – is a huge part of understanding how difficult software development was before the advent of DevOps. Later in this book, when you see how GitLab CI/CD pipelines simplify the process of running different kinds of tests and viewing their results, and when you understand how tests that run early and often make problems easier to detect and cheaper to fix, you can look back at these cumbersome test procedures and feel sympathy for the poor developers who had to wade through this part of the SDLC before GitLab existed. Life is much better in the GitLab era!