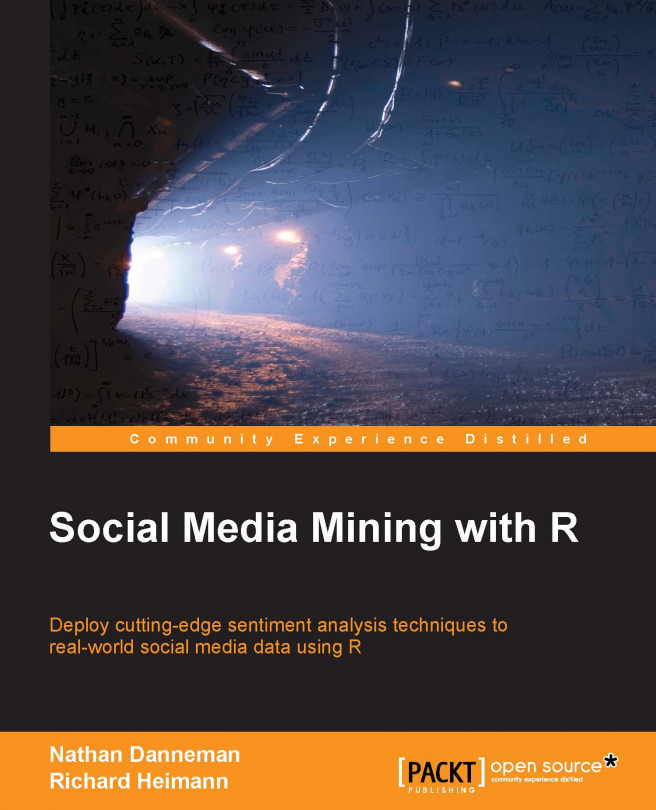
Social Media Mining with R
What do you get with a Packt Subscription?
What do you get with a Packt Subscription?
What do you get with eBook + Subscription?
What do you get with a Packt Subscription?
What do you get with eBook?
What do I get with Print?
What do I get with Print?
What do you get with video?
What do you get with video?
What do you get with Audiobook?
What do you get with Exam Trainer?
What do you get with a Packt Subscription?
What do you get with a Packt Subscription?
What do you get with eBook + Subscription?
What do you get with a Packt Subscription?
What do you get with eBook?
What do I get with Print?
What do I get with Print?
What do you get with video?
What do you get with video?
What do you get with Audiobook?
What do you get with Exam Trainer?