Introduction
In this chapter, we'll have a gentle introduction tonatural language processing (NLP) and how natural language processing concepts are used in real-life artificial intelligence applications. We will focus mainly on Python programming paradigms, which are used to develop NLP applications. Later on, the chapter has a tips section for readers. If you are really interested in finding out about the comparison of various programming paradigms for NLP and why Python is the best programming paradigm then, as a reader, you should go through the Preface of this book. As an industry professional, I have tried most of the programming paradigms for NLP. I have used Java, R, and Python for NLP applications. Trust me, guys, Python is quite easy and efficient for developing applications that use NLP concepts.
We will cover following topics in this chapter:
- Understanding NLP
- Understanding basic applications
- Understanding advance applications
- Advantages of the togetherness--NLP and Python
- Environment setup for NLTK
- Tips for readers
Understanding natural language processing
In the last few years, branches of artificial intelligence (AI) have created a lot of buzz, and those branches are data science, data analytics, predictive analysis, NLP, and so on.
As mentioned in the Preface of this book, we are focusing on Python and natural language processing. Let me ask you some questions--Do you really know what natural language is? What is natural language processing? What are the other branches involved in building expert systems using various concepts of natural language processing? How can we build intelligent systems using the concept of NLP?
Let's begin our roller coaster ride of understanding NLP.
What is natural language?
- As a human being, we express our thoughts or feelings via a language
- Whatever you speak, read, write, or listen to is mostly in the form of natural language, so it is commonly expressed as natural language
- For example:
- The content of this book is a source of natural language
- Whatever you speak, listen, and write in your daily life is also in the form of natural language
- Movie dialogues are also a source of natural language
- Your WhatsApp conversations are also considered a form of natural language
What is natural language processing?
- Now you have an understanding of what natural language is. NLP is a sub-branch of AI. Let's consider an example and understand the concept of NLP. Let's say you want to build a machine that interacts with humans in the form of natural language. This kind of an intelligent system needs computational technologies and computational linguistics to build it, and the system processes natural language like humans.
- You can relate the aforementioned concept of NLP to the existing NLP products from the world's top tech companies, such as Google Assistant from Google, Siri speech assistance from Apple, and so on.
- Now you will able to understand the definitions of NLP, which are as follows:
- Natural language processing is the ability of computational technologies and/or computational linguistics to process human natural language
- Natural language processing is a field of computer science, artificial intelligence, and computational linguistics concerned with the interactions between computers and human (natural) languages
- Natural language processing can be defined as the automatic (or semi-automatic) processing of human natural language
What are the other branches involved in building expert systems using, various concepts of NLP? Figure 1.1 is the best way to know how many other branches are involved when you are building an expert system using NLP concepts:
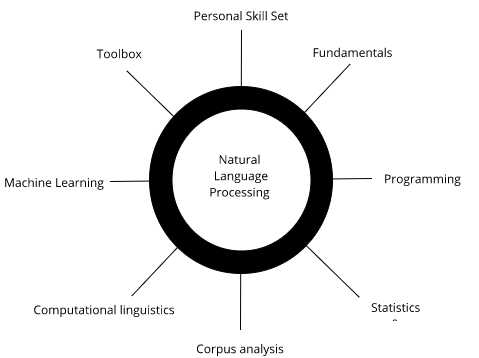
Figures 1.2 and 1.3 convey all the subtopics that are included in every branch given in Figure 1.1:
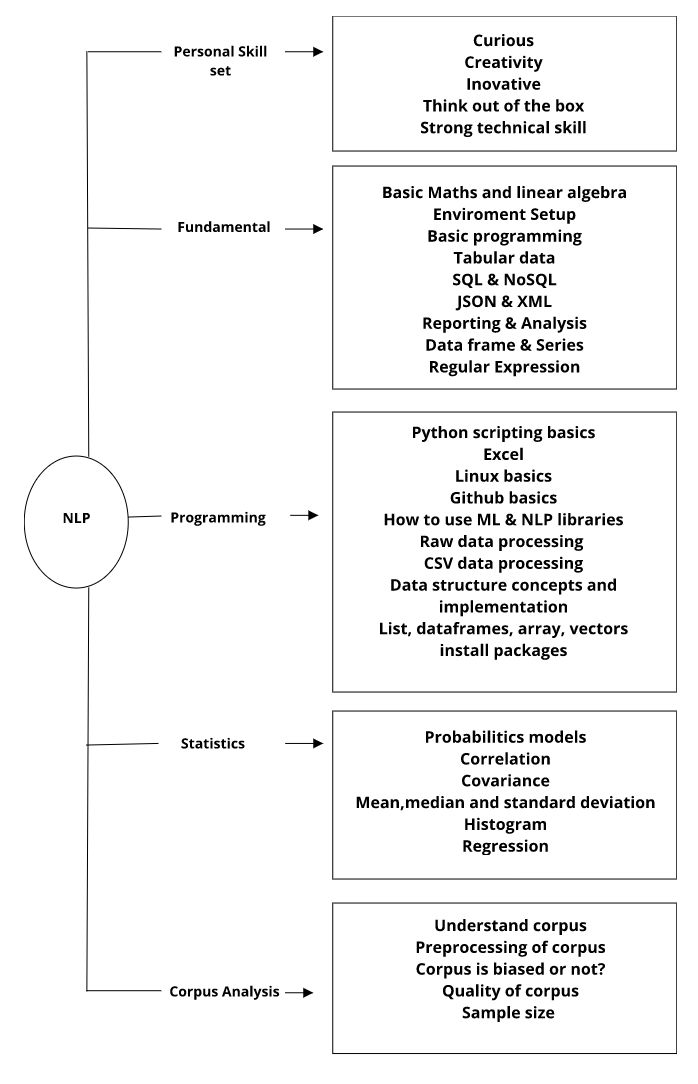
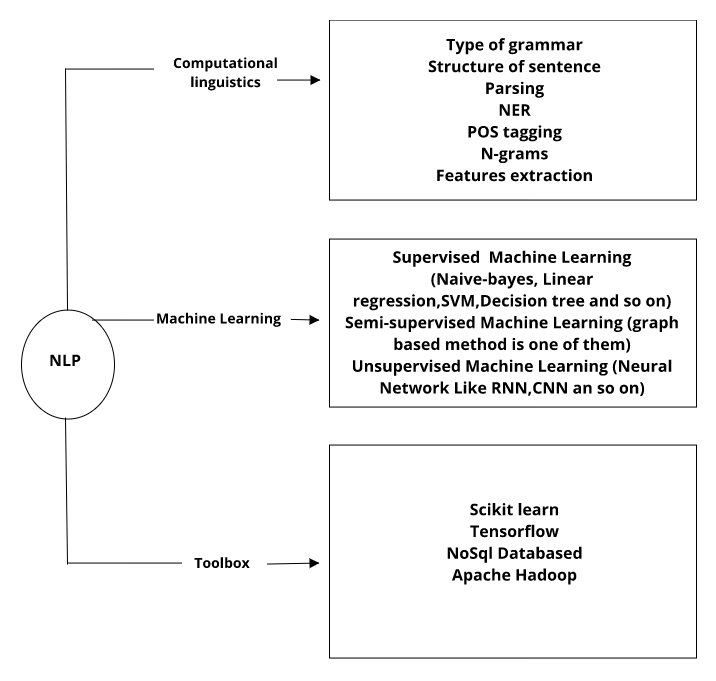
How can we build an intelligent system using concepts of NLP? Figure 1.4 is the basic model, which indicates how an expert system can be built for NLP applications. The development life cycle is defined in the following figure:
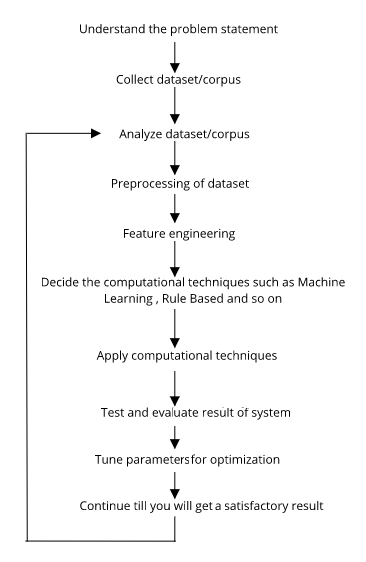
Let's see some of the details of the development life cycle of NLP-related problems:
- If you are solving an NLP problem, you first need to understand the problem statement.
- Once you understand your problem statement, think about what kind of data or corpus you need to solve the problem. So, data collection is the basic activity toward solving the problem.
- After you have collected a sufficient amount of data, you can start analyzing your data. What is the quality and quantity of our corpus? According to the quality of the data and your problem statement, you need to do preprocessing.
- Once you are done with preprocessing, you need to start with the process of feature engineering. Feature engineering is the most important aspect of NLP and data science related applications. We will be covering feature engineering related aspects in much more detail in Chapter 5, Feature Engineering and NLP Algorithms and Chapter 6, Advance Feature Engineering and NLP Algorithms.
- Having decided on and extracted features from the raw preprocessed data, you are to decide which computational technique is useful to solve your problem statement, for example, do you want to apply machine learning techniques or rule-based techniques?.
- Now, depending on what techniques you are going to use, you should ready the feature files that you are going to provide as an input to your decided algorithm.
- Run your logic, then generate the output.
- Test and evaluate your system's output.
- Tune the parameters for optimization, and continue till you get satisfactory results.
We will be covering a lot of information very quickly in this chapter, so if you see something that doesn't immediately make sense, please do not feel lost and bear with me. We will explore all the details and examples from the next chapter onward, and that will definitely help you connect the dots.
Understanding basic applications
NLP is a sub-branch of AI. Concepts from NLP are used in the following expert systems:
- Speech recognition system
- Question answering system
- Translation from one specific language to another specific language
- Text summarization
- Sentiment analysis
- Template-based chatbots
- Text classification
- Topic segmentation
We will learn about most of the NLP concepts that are used in the preceding applications in the further chapters.
Understanding advanced applications
Advanced applications include the following:
- Human robots who understand natural language commands and interact with humans in natural language.
- Building a universal machine translation system is the long-term goal in the NLP domain because you could easily build a machine translation system which can convert one specific language to another specific language, but that system may not help you to translate other languages. With the help of deep learning, we can develop a universal machine translation system and Google recently announced that they are very close to achieving this goal. We will build our own machine translation system using deep learning in Chapter 9, Deep Learning for NLP and NLG Problems.
- The NLP system, which generates the logical title for the given document is one of the advance applications. Also, with the help of deep learning, you can generate the title of document and perform summarization on top of that. This kind of application, you will see in Chapter 9, Deep Learning for NLP and NLG Problems.
- The NLP system, which generates text for specific topics or for an image is also considered an advanced NLP application.
- Advanced chatbots, which generate personalized text for humans and ignore mistakes in human writing is also a goal we are trying to achieve.
- There are many other NLP applications, which you can see in Figure 1.5:
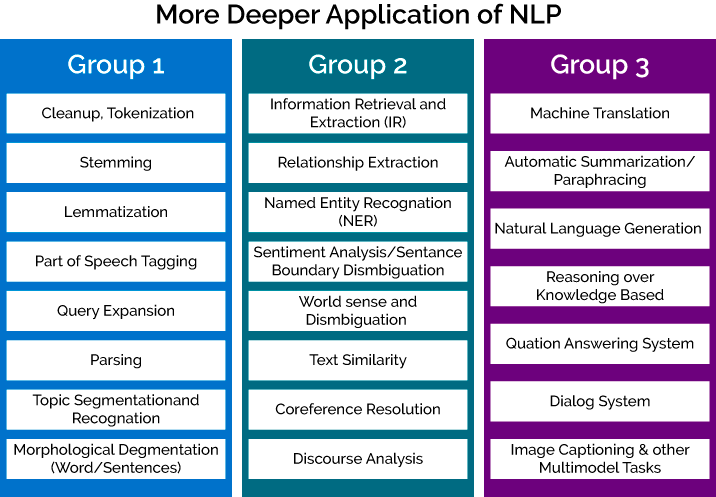
Advantages of togetherness - NLP and Python
The following points illustrate why Python is one of the best options to build an NLP-based expert system:
- Developing prototypes for the NLP-based expert system using Python is very easy and efficient
- A large variety of open source NLP libraries are available for Python programmers
- Community support is very strong
- Easy to use and less complex for beginners
- Rapid development: testing, and evaluation are easy and less complex
- Many of the new frameworks, such as Apache Spark, Apache Flink, TensorFlow, and so on, provide API for Python
- Optimization of the NLP-based system is less complex compared to other programming paradigms
Environment setup for NLTK
I would like to suggest to all my readers that they pull the NLPython repository on GitHub. The repository URL is https://github.com/jalajthanaki/NLPython
I'm using Linux (Ubuntu) as the operating system, so if you are not familiar with Linux, it's better for you to make yourself comfortable with it, because most of the advanced frameworks, such as Apache Hadoop, Apache Spark, Apache Flink, Google TensorFlow, and so on, require a Linux operating system.
The GitHub repository contains instructions on how to install Linux, as well as basic Linux commands which we will use throughout this book. On GitHub, you can also find basic commands for GitHub if you are new to Git as well. The URL is https://github.com/jalajthanaki/NLPython/tree/master/ch1/documentation
I'm providing an installation guide for readers to set up the environment for these chapters. The URL is https://github.com/jalajthanaki/NLPython/tree/master/ch1/installation_guide
Steps for installing nltk are as follows (or you can follow the URL: https://github.com/jalajthanaki/NLPython/blob/master/ch1/installation_guide/NLTK%2BSetup.md):
- Install Python 2.7.x manually, but on Linux Ubuntu 14.04, it has already been installed; otherwise, you can check your Python version using the python -V command.
- Configure pip for installing Python libraries (https://github.com/jalajthanaki/NLPython/blob/master/ch1/installation_guide/NLTK%2BSetup.md).
- Open the terminal, and execute the following command:
pip install nltk or sudo pip install nltk
- Open the terminal, and execute the python command.
- Inside the Python shell, execute the import nltk command.
If your nltk module is successfully installed on your system, the system will not throw any messages.
- Inside the Python shell, execute the nltk.download() command.
- This will open an additional dialog window, where you can choose specific libraries, but in our case, click on All packages, and you can choose the path where the packages reside. Wait till all the packages are downloaded. It may take a long time to download. After completion of the download, you can find the folder named nltk_data at the path specified by you earlier. Take a look at the NLTK Downloader in the following screenshot:
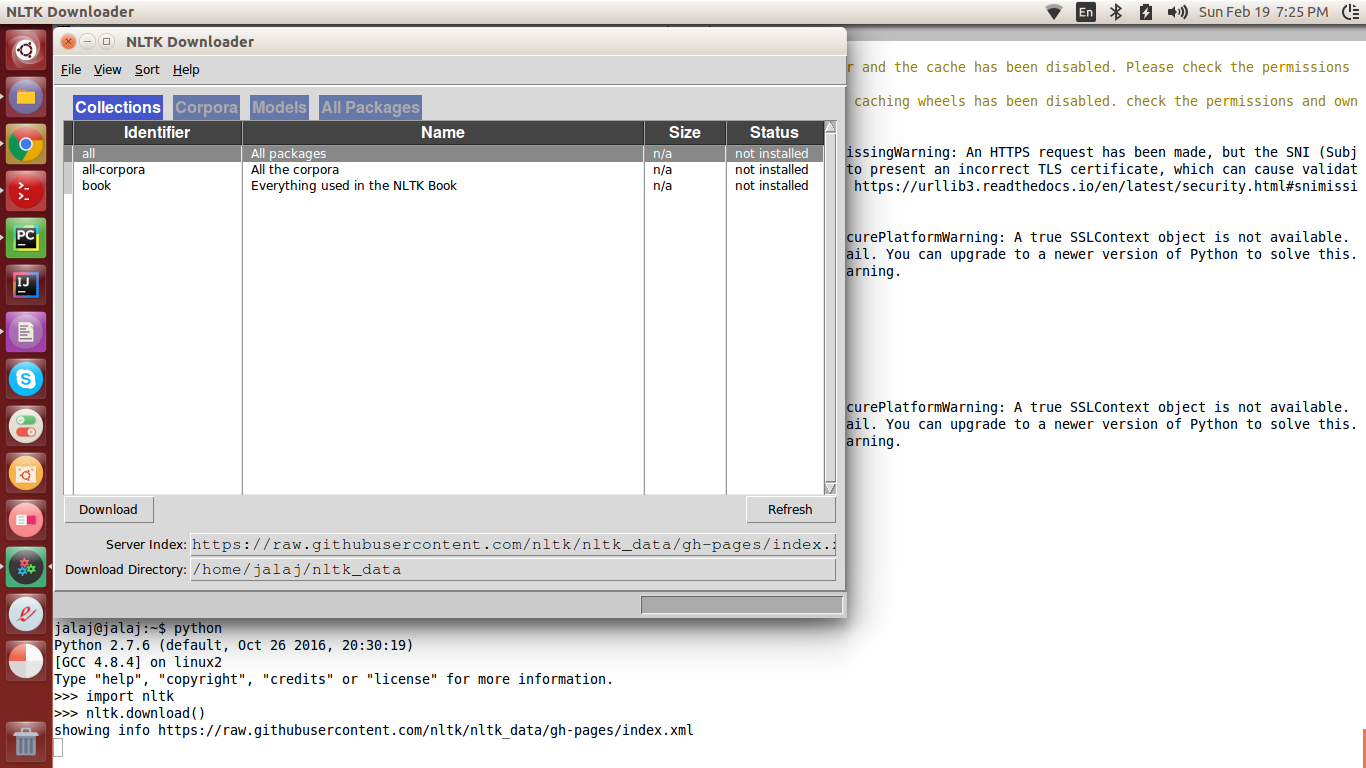
This repository contains an installation guide, codes, wiki page, and so on. If readers have questions and queries, they can post their queries on the Gitter group. The Gitter group URL is https://gitter.im/NLPython/Lobby?utm_source=share-link&utm_medium=link&utm_campaign=share-link
Tips for readers
This book is a practical guide. As an industry professional, I strongly recommend all my readers replicate the code that is already available on GitHub and perform the exercises given in the book. This will improve your understanding of NLP concepts. Without performing the practicals, it will be nearly impossible for you to get all the NLP concepts thoroughly. By the way, I promise that it will be fun to implement them.
The flow of upcoming chapters is as follows:
- Explanation of the concepts
- Application of the concepts
- Needs of the concepts
- Possible ways to implement the concepts (code is on GitHub)
- Challenges of the concepts
- Tips to overcome challenges
- Exercises
Summary
This chapter gave you an introduction to NLP. You now have a brief idea about what kind of branches are involved in NLP and the various stages for building an expert system using NLP concepts. Lastly, we set up the environment for NLTK. All the installation guidelines and codes are available on GitHub.
In the next chapter, we will see what kind of corpus is used on NLP-related applications and what all the critical points we should keep in mind are when we analyze a corpus. We will deal with the different types of file formats and datasets. Let's explore this together!