Data is raw information that can exist in any form, usable or not. We can easily get data everywhere in our lives; for example, the price of gold on the day of writing was $ 1.158 per ounce. This does not have any meaning, except describing the price of gold. This also shows that data is useful based on context.
In this chapter, we will cover the following topics:
Data is getting bigger and more diverse every day. Therefore, analyzing and processing data to advance human knowledge or to create value is a big challenge. To tackle these challenges, you will need domain knowledge and a variety of skills, drawing from areas such as computer science, artificial intelligence (AI) and machine learning (ML), statistics and mathematics, and knowledge domain, as shown in the following figure:
Let's go through data analysis and its domain knowledge:
- Computer science: We need this knowledge to provide abstractions for efficient data processing. Basic Python programming experience is required to follow the next chapters. We will introduce Python libraries used in data analysis.
- Artificial intelligence and machine learning: If computer science knowledge helps us to program data analysis tools, artificial intelligence and machine learning help us to model the data and learn from it in order to build smart products.
- Statistics and mathematics: We cannot extract useful information from raw data if we do not use statistical techniques or mathematical functions.
- Knowledge domain: Besides technology and general techniques, it is important to have an insight into the specific domain. What do the data fields mean? What data do we need to collect? Based on the expertise, we explore and analyze raw data by applying the above techniques, step by step.
Data analysis is a process composed of the following steps:
- Data requirements: We have to define what kind of data will be collected based on the requirements or problem analysis. For example, if we want to detect a user's behavior while reading news on the internet, we should be aware of visited article links, dates and times, article categories, and the time the user spends on different pages.
- Data collection: Data can be collected from a variety of sources: mobile, personal computer, camera, or recording devices. It may also be obtained in different ways: communication, events, and interactions between person and person, person and device, or device and device. Data appears whenever and wherever in the world. The problem is how we can find and gather it to solve our problem? This is the mission of this step.
- Data processing: Data that is initially obtained must be processed or organized for analysis. This process is performance-sensitive. How fast can we create, insert, update, or query data? When building a real product that has to process big data, we should consider this step carefully. What kind of database should we use to store data? What kind of data structure, such as analysis, statistics, or visualization, is suitable for our purposes?
- Data cleaning: After being processed and organized, the data may still contain duplicates or errors. Therefore, we need a cleaning step to reduce those situations and increase the quality of the results in the following steps. Common tasks include record matching, deduplication, and column segmentation. Depending on the type of data, we can apply several types of data cleaning. For example, a user's history of visits to a news website might contain a lot of duplicate rows, because the user might have refreshed certain pages many times. For our specific issue, these rows might not carry any meaning when we explore the user's behavior so we should remove them before saving it to our database. Another situation we may encounter is click fraud on news—someone just wants to improve their website ranking or sabotage a website. In this case, the data will not help us to explore a user's behavior. We can use thresholds to check whether a visit page event comes from a real person or from malicious software.
- Exploratory data analysis: Now, we can start to analyze data through a variety of techniques referred to as exploratory data analysis. We may detect additional problems in data cleaning or discover requests for further data. Therefore, these steps may be iterative and repeated throughout the whole data analysis process. Data visualization techniques are also used to examine the data in graphs or charts. Visualization often facilitates understanding of data sets, especially if they are large or high-dimensional.
- Modelling and algorithms: A lot of mathematical formulas and algorithms may be applied to detect or predict useful knowledge from the raw data. For example, we can use similarity measures to cluster users who have exhibited similar news-reading behavior and recommend articles of interest to them next time. Alternatively, we can detect users' genders based on their news reading behavior by applying classification models such as the Support Vector Machine (SVM) or linear regression. Depending on the problem, we may use different algorithms to get an acceptable result. It can take a lot of time to evaluate the accuracy of the algorithms and choose the best one to implement for a certain product.
- Data product: The goal of this step is to build data products that receive data input and generate output according to the problem requirements. We will apply computer science knowledge to implement our selected algorithms as well as manage the data storage.
There are numerous data analysis libraries that help us to process and analyze data. They use different programming languages, and have different advantages and disadvantages of solving various data analysis problems. Now, we will introduce some common libraries that may be useful for you. They should give you an overview of the libraries in the field. However, the rest of this book focuses on Python-based libraries.
Some of the libraries that use the Java language for data analysis are as follows:
- Weka: This is the library that I became familiar with the first time I learned about data analysis. It has a graphical user interface that allows you to run experiments on a small dataset. This is great if you want to get a feel for what is possible in the data processing space. However, if you build a complex product, I think it is not the best choice, because of its performance, sketchy API design, non-optimal algorithms, and little documentation (http://www.cs.waikato.ac.nz/ml/weka/).
- Mallet: This is another Java library that is used for statistical natural language processing, document classification, clustering, topic modeling, information extraction, and other machine-learning applications on text. There is an add-on package for Mallet, called GRMM, that contains support for inference in general, graphical models, and training of Conditional random fields (CRF) with arbitrary graphical structures. In my experience, the library performance and the algorithms are better than Weka. However, its only focus is on text-processing problems. The reference page is at http://mallet.cs.umass.edu/.
- Mahout: This is Apache's machine-learning framework built on top of Hadoop; its goal is to build a scalable machine-learning library. It looks promising, but comes with all the baggage and overheads of Hadoop. The homepage is at http://mahout.apache.org/.
- Spark: This is a relatively new Apache project, supposedly up to a hundred times faster than Hadoop. It is also a scalable library that consists of common machine-learning algorithms and utilities. Development can be done in Python as well as in any JVM language. The reference page is at https://spark.apache.org/docs/1.5.0/mllib-guide.html.
Here are a few libraries that are implemented in C++:
- Vowpal Wabbit: This library is a fast, out-of-core learning system sponsored by Microsoft Research and, previously, Yahoo! Research. It has been used to learn a tera-feature (1012) dataset on 1,000 nodes in one hour. More information can be found in the publication at http://arxiv.org/abs/1110.4198.
- MultiBoost: This package is a multiclass, multi label, and multitask classification boosting software implemented in C++. If you use this software, you should refer to the paper published in 2012 in the JournalMachine Learning Research, MultiBoost: A Multi-purpose Boosting Package, D.Benbouzid, R. Busa-Fekete, N. Casagrande, F.-D. Collin, and B. Kégl.
- MLpack: This is also a C++ machine-learning library, developed by the Fundamental Algorithmic and Statistical Tools Laboratory (FASTLab) at Georgia Tech. It focusses on scalability, speed, and ease-of-use, and was presented at the BigLearning workshop of NIPS 2011. Its homepage is at http://www.mlpack.org/about.html.
- Caffe: The last C++ library we want to mention is Caffe. This is a deep learning framework made with expression, speed, and modularity in mind. It is developed by the Berkeley Vision and Learning Center (BVLC) and community contributors. You can find more information about it at http://caffe.berkeleyvision.org/.
Other libraries for data processing and analysis are as follows:
- Statsmodels: This is a great Python library for statistical modeling and is mainly used for predictive and exploratory analysis.
- Modular toolkit for data processing (MDP): This is a collection of supervised and unsupervised learning algorithms and other data processing units that can be combined into data processing sequences and more complex feed-forward network architectures (http://mdp-toolkit.sourceforge.net/index.html).
- Orange: This is an open source data visualization and analysis for novices and experts. It is packed with features for data analysis and has add-ons for bioinformatics and text mining. It contains an implementation of self-organizing maps, which sets it apart from the other projects as well (http://orange.biolab.si/).
- Mirador: This is a tool for the visual exploration of complex datasets, supporting Mac and Windows. It enables users to discover correlation patterns and derive new hypotheses from data (http://orange.biolab.si/).
- RapidMiner: This is another GUI-based tool for data mining, machine learning, and predictive analysis (https://rapidminer.com/).
- Theano: This bridges the gap between Python and lower-level languages. Theano gives very significant performance gains, particularly for large matrix operations, and is, therefore, a good choice for deep learning models. However, it is not easy to debug because of the additional compilation layer.
- Natural language processing toolkit (NLTK): This is written in Python with very unique and salient features.
Python is a multi-platform, general-purpose programming language that can run on Windows, Linux/Unix, and Mac OS X, and has been ported to Java and .NET virtual machines as well. It has a powerful standard library. In addition, it has many libraries for data analysis: Pylearn2, Hebel, Pybrain, Pattern, MontePython, and MILK. In this book, we will cover some common Python data analysis libraries such as Numpy, Pandas, Matplotlib, PyMongo, and scikit-learn. Now, to help you get started, I will briefly present an overview of each library for those who are less familiar with the scientific Python stack.
One of the fundamental packages used for scientific computing in Python is Numpy. Among other things, it contains the following:
Pandas is a Python package that supports rich data structures and functions for analyzing data, and is developed by the PyData Development Team. It is focused on the improvement of Python's data libraries. Pandas consists of the following things:
- A set of labeled array data structures; the primary of which are Series, DataFrame, and Panel
- Index objects enabling both simple axis indexing and multilevel/hierarchical axis indexing
- An intergraded group by engine for aggregating and transforming datasets
- Date range generation and custom date offsets
- Input/output tools that load and save data from flat files or PyTables/HDF5 format
- Optimal memory versions of the standard data structures
- Moving window statistics and static and moving window linear/panel regression
Due to these features, Pandas is an ideal tool for systems that need complex data structures or high-performance time series functions such as financial data analysis applications.
Matplotlib is the single most used Python package for 2D-graphics. It provides both a very quick way to visualize data from Python and publication-quality figures in many formats: line plots, contour plots, scatter plots, and Basemap plots. It comes with a set of default settings, but allows customization of all kinds of properties. However, we can easily create our chart with the defaults of almost every property in Matplotlib.
MongoDB is a type of NoSQL database. It is highly scalable, robust, and perfect to work with JavaScript-based web applications, because we can store data as JSON documents and use flexible schemas.
The scikit-learn is an open source machine-learning library using the Python programming language. It supports various machine learning models, such as classification, regression, and clustering algorithms, interoperated with the Python numerical and scientific libraries NumPy and SciPy. The latest scikit-learn version is 0.16.1, published in April 2015.
the fundamental packages used for scientific computing in Python is Numpy. Among other things, it contains the following:
Pandas is a Python package that supports rich data structures and functions for analyzing data, and is developed by the PyData Development Team. It is focused on the improvement of Python's data libraries. Pandas consists of the following things:
- A set of labeled array data structures; the primary of which are Series, DataFrame, and Panel
- Index objects enabling both simple axis indexing and multilevel/hierarchical axis indexing
- An intergraded group by engine for aggregating and transforming datasets
- Date range generation and custom date offsets
- Input/output tools that load and save data from flat files or PyTables/HDF5 format
- Optimal memory versions of the standard data structures
- Moving window statistics and static and moving window linear/panel regression
Due to these features, Pandas is an ideal tool for systems that need complex data structures or high-performance time series functions such as financial data analysis applications.
Matplotlib is the single most used Python package for 2D-graphics. It provides both a very quick way to visualize data from Python and publication-quality figures in many formats: line plots, contour plots, scatter plots, and Basemap plots. It comes with a set of default settings, but allows customization of all kinds of properties. However, we can easily create our chart with the defaults of almost every property in Matplotlib.
MongoDB is a type of NoSQL database. It is highly scalable, robust, and perfect to work with JavaScript-based web applications, because we can store data as JSON documents and use flexible schemas.
The scikit-learn is an open source machine-learning library using the Python programming language. It supports various machine learning models, such as classification, regression, and clustering algorithms, interoperated with the Python numerical and scientific libraries NumPy and SciPy. The latest scikit-learn version is 0.16.1, published in April 2015.
Python package that supports rich data structures and functions for analyzing data, and is developed by the PyData Development Team. It is focused on the improvement of Python's data libraries. Pandas consists of the following things:
- A set of labeled array data structures; the primary of which are Series, DataFrame, and Panel
- Index objects enabling both simple axis indexing and multilevel/hierarchical axis indexing
- An intergraded group by engine for aggregating and transforming datasets
- Date range generation and custom date offsets
- Input/output tools that load and save data from flat files or PyTables/HDF5 format
- Optimal memory versions of the standard data structures
- Moving window statistics and static and moving window linear/panel regression
Due to these features, Pandas is an ideal tool for systems that need complex data structures or high-performance time series functions such as financial data analysis applications.
Matplotlib is the single most used Python package for 2D-graphics. It provides both a very quick way to visualize data from Python and publication-quality figures in many formats: line plots, contour plots, scatter plots, and Basemap plots. It comes with a set of default settings, but allows customization of all kinds of properties. However, we can easily create our chart with the defaults of almost every property in Matplotlib.
MongoDB is a type of NoSQL database. It is highly scalable, robust, and perfect to work with JavaScript-based web applications, because we can store data as JSON documents and use flexible schemas.
The scikit-learn is an open source machine-learning library using the Python programming language. It supports various machine learning models, such as classification, regression, and clustering algorithms, interoperated with the Python numerical and scientific libraries NumPy and SciPy. The latest scikit-learn version is 0.16.1, published in April 2015.
is the single most used Python package for 2D-graphics. It provides both a very quick way to visualize data from Python and publication-quality figures in many formats: line plots, contour plots, scatter plots, and Basemap plots. It comes with a set of default settings, but allows customization of all kinds of properties. However, we can easily create our chart with the defaults of almost every property in Matplotlib.
MongoDB is a type of NoSQL database. It is highly scalable, robust, and perfect to work with JavaScript-based web applications, because we can store data as JSON documents and use flexible schemas.
The scikit-learn is an open source machine-learning library using the Python programming language. It supports various machine learning models, such as classification, regression, and clustering algorithms, interoperated with the Python numerical and scientific libraries NumPy and SciPy. The latest scikit-learn version is 0.16.1, published in April 2015.
is a type of NoSQL database. It is highly scalable, robust, and perfect to work with JavaScript-based web applications, because we can store data as JSON documents and use flexible schemas.
The scikit-learn is an open source machine-learning library using the Python programming language. It supports various machine learning models, such as classification, regression, and clustering algorithms, interoperated with the Python numerical and scientific libraries NumPy and SciPy. The latest scikit-learn version is 0.16.1, published in April 2015.
scikit-learn is an open source machine-learning library using the Python programming language. It supports various machine learning models, such as classification, regression, and clustering algorithms, interoperated with the Python numerical and scientific libraries NumPy and SciPy. The latest scikit-learn version is 0.16.1, published in April 2015.
The following table describes users' rankings on Snow White movies:
UserID |
Sex |
Location |
Ranking |
---|---|---|---|
A |
Male |
Philips |
4 |
B |
Male |
VN |
2 |
C |
Male |
Canada |
1 |
D |
Male |
Canada |
2 |
E |
Female |
VN |
5 |
F |
Female |
NY |
4 |
NumPy is the fundamental package supported for presenting and computing data with high performance in Python. It provides some interesting features as follows:
- Extension package to Python for multidimensional arrays (
ndarrays
), various derived objects (such as masked arrays), matrices providing vectorization operations, and broadcasting capabilities. Vectorization can significantly increase the performance of array computations by taking advantage of Single Instruction Multiple Data (SIMD) instruction sets in modern CPUs. - Fast and convenient operations on arrays of data, including mathematical manipulation, basic statistical operations, sorting, selecting, linear algebra, random number generation, discrete Fourier transforms, and so on.
- Efficiency tools that are closer to hardware because of integrating C/C++/Fortran code.
An array can be used to contain values of a data object in an experiment or simulation step, pixels of an image, or a signal recorded by a measurement device. For example, the latitude of the Eiffel Tower, Paris is 48.858598 and the longitude is 2.294495. It can be presented in a NumPy array object as p
:
This is a manual construction of an array using the np.array
function. The standard convention to import NumPy is as follows:
You can, of course, put from numpy import *
in your code to avoid having to write np
. However, you should be careful with this habit because of the potential code conflicts (further information on code conventions can be found in the Python Style Guide, also known as PEP8, at https://www.python.org/dev/peps/pep-0008/).
There are two requirements of a NumPy array: a fixed size at creation and a uniform, fixed data type, with a fixed size in memory. The following functions help you to get information on the p
matrix:
>>> p.ndim # getting dimension of array p 1 >>> p.shape # getting size of each array dimension (2,) >>> len(p) # getting dimension length of array p 2 >>> p.dtype # getting data type of array p dtype('float64')
There are five basic numerical types including Booleans (bool
), integers (int
), unsigned integers (uint
), floating point (float
), and complex. They indicate how many bits are needed to represent elements of an array in memory. Besides that, NumPy also has some types, such as intc
and intp
, that have different bit sizes depending on the platform.
See the following table for a listing of NumPy's supported data types:
Type |
Type code |
Description |
Range of value |
---|---|---|---|
|
Boolean stored as a byte |
True/False | |
|
Similar to C int (int32 or int 64) | ||
|
Integer used for indexing (same as C size_t) | ||
|
i1, u1 |
Signed and unsigned 8-bit integer types |
int8: (-128 to 127) uint8: (0 to 255) |
|
i2, u2 |
Signed and unsigned 16-bit integer types |
int16: (-32768 to 32767) uint16: (0 to 65535) |
|
I4, u4 |
Signed and unsigned 32-bit integer types |
int32: (-2147483648 to 2147483647 uint32: (0 to 4294967295) |
|
i8, u8 |
Signed and unsigned 64-bit integer types |
Int64: (-9223372036854775808 to 9223372036854775807) uint64: (0 to 18446744073709551615) |
|
f2 |
Half precision float: sign bit, 5 bits exponent, and 10b bits mantissa | |
|
f4 / f |
Single precision float: sign bit, 8 bits exponent, and 23 bits mantissa | |
|
f8 / d |
Double precision float: sign bit, 11 bits exponent, and 52 bits mantissa | |
|
c8, c16, c32 |
Complex numbers represented by two 32-bit, 64-bit, and 128-bit floats | |
|
0 |
Python object type | |
|
S |
Fixed-length string type |
Declare a string |
|
U |
Fixed-length Unicode type |
Similar to string_ example, we have 'U10' |
We can easily convert or cast an array from one dtype
to another using the astype
method:
There are various functions provided to create an array object. They are very useful for us to create and store data in a multidimensional array in different situations.
Function |
Description |
Example |
---|---|---|
|
Create a new array of the given shape and type, without initializing elements |
>>> np.empty([3,2], dtype=np.float64) array([[0., 0.], [0., 0.], [0., 0.]]) >>> a = np.array([[1, 2], [4, 3]]) >>> np.empty_like(a) array([[0, 0], [0, 0]])
|
|
Create a NxN identity matrix with ones on the diagonal and zero elsewhere |
>>> np.eye(2, dtype=np.int) array([[1, 0], [0, 1]])
|
|
Create a new array with the given shape and type, filled with 1s for all elements |
>>> np.ones(5) array([1., 1., 1., 1., 1.]) >>> np.ones(4, dtype=np.int) array([1, 1, 1, 1]) >>> x = np.array([[0,1,2], [3,4,5]]) >>> np.ones_like(x) array([[1, 1, 1],[1, 1, 1]])
|
|
This is similar to |
>>> np.zeros(5) array([0., 0., 0., 0-, 0.]) >>> np.zeros(4, dtype=np.int) array([0, 0, 0, 0]) >>> x = np.array([[0, 1, 2], [3, 4, 5]]) >>> np.zeros_like(x) array([[0, 0, 0],[0, 0, 0]])
|
|
Create an array with even spaced values in a given interval |
>>> np.arange(2, 5) array([2, 3, 4]) >>> np.arange(4, 12, 5) array([4, 9])
|
|
Create a new array with the given shape and type, filled with a selected value |
>>> np.full((2,2), 3, dtype=np.int) array([[3, 3], [3, 3]]) >>> x = np.ones(3) >>> np.full_like(x, 2) array([2., 2., 2.])
|
|
Create an array from the existing data |
>>> np.array([[1.1, 2.2, 3.3], [4.4, 5.5, 6.6]]) array([1.1, 2.2, 3.3], [4.4, 5.5, 6.6]])
|
|
Convert the input to an array |
>>> a = [3.14, 2.46] >>> np.asarray(a) array([3.14, 2.46])
|
|
Return an array copy of the given object |
>>> a = np.array([[1, 2], [3, 4]]) >>> np.copy(a) array([[1, 2], [3, 4]])
|
|
Create 1-D array from a string or text |
>>> np.fromstring('3.14 2.17', dtype=np.float, sep=' ') array([3.14, 2.17])
|
As with other Python sequence types, such as lists, it is very easy to access and assign a value of each array's element:
As another example, if our array is multidimensional, we need tuples of integers to index an item:
We call b
and c
as array slices, which are views on the original one. It means that the data is not copied to b
or c
, and whenever we modify their values, it will be reflected in the array a
as well:
Besides indexing with slices, NumPy also supports indexing with Boolean or integer arrays (masks). This method is called fancy indexing. It creates copies, not views.
First, we take a look at an example of indexing with a Boolean mask array:
The second example is an illustration of using integer masks on arrays:
Tip
You can download the example code files for all Packt books you have purchased from your account at http://www.packtpub.com. If you purchased this book elsewhere, you can visit http://www.packtpub.com/support and register to have the files e-mailed directly to you.
We are getting familiar with creating and accessing ndarrays
. Now, we continue to the next step, applying some mathematical operations to array data without writing any for loops, of course, with higher performance.
Scalar operations will propagate the value to each element of the array:
All arithmetic operations between arrays apply the operation element wise:
Also, here are some examples of comparisons and logical operations:
Many helpful array functions are supported in NumPy for analyzing data. We will list some part of them that are common in use. Firstly, the transposing function is another kind of reshaping form that returns a view on the original data array without copying anything:
See the following table for a listing of array functions:
Function |
Description |
Example |
---|---|---|
|
Trigonometric and hyperbolic functions |
>>> a = np.array([0.,30., 45.]) >>> np.sin(a * np.pi / 180) array([0., 0.5, 0.7071678])
|
|
Rounding elements of an array to the given or nearest number |
>>> a = np.array([0.34, 1.65]) >>> np.round(a) array([0., 2.])
|
|
Computing the exponents and logarithms of an array |
>>> np.exp(np.array([2.25, 3.16])) array([9.4877, 23.5705])
|
|
Set of arithmetic functions on arrays |
>>> a = np.arange(6) >>> x1 = a.reshape(2,3) >>> x2 = np.arange(3) >>> np.multiply(x1, x2) array([[0,1,4],[0,4,10]])
|
|
Perform elementwise comparison: >, >=, <, <=, ==, != |
>>> np.greater(x1, x2) array([[False, False, False], [True, True, True]], dtype = bool)
|
With the NumPy package, we can easily solve many kinds of data processing tasks without writing complex loops. It is very helpful for us to control our code as well as the performance of the program. In this part, we want to introduce some mathematical and statistical functions.
See the following table for a listing of mathematical and statistical functions:
Function |
Description |
Example |
---|---|---|
|
Calculate the sum of all the elements in an array or along the axis |
>>> a = np.array([[2,4], [3,5]]) >>> np.sum(a, axis=0) array([5, 9])
|
|
Compute the product of array elements over the given axis |
>>> np.prod(a, axis=1) array([8, 15])
|
|
Calculate the discrete difference along the given axis |
>>> np.diff(a, axis=0) array([[1,1]])
|
|
Return the gradient of an array |
>>> np.gradient(a) [array([[1., 1.], [1., 1.]]), array([[2., 2.], [2., 2.]])]
|
|
Return the cross product of two arrays |
>>> b = np.array([[1,2], [3,4]]) >>> np.cross(a,b) array([0, -3])
|
|
Return standard deviation and variance of arrays |
>>> np.std(a) 1.1180339 >>> np.var(a) 1.25
|
|
Calculate arithmetic mean of an array |
>>> np.mean(a) 3.5
|
|
Return elements, either from x or y, that satisfy a condition |
>>> np.where([[True, True], [False, True]], [[1,2],[3,4]], [[5,6],[7,8]]) array([[1,2], [7, 4]])
|
|
Return the sorted unique values in an array |
>>> id = np.array(['a', 'b', 'c', 'c', 'd']) >>> np.unique(id) array(['a', 'b', 'c', 'd'], dtype='|S1')
|
|
Compute the sorted and common elements in two arrays |
>>> a = np.array(['a', 'b', 'a', 'c', 'd', 'c']) >>> b = np.array(['a', 'xyz', 'klm', 'd']) >>> np.intersect1d(a,b) array(['a', 'd'], dtype='|S3')
|
Arrays are saved by default in an uncompressed raw binary format, with the file extension .npy
by the np.save
function:
Arrays are saved by default in an uncompressed raw binary format, with the file extension .npy
by the np.save
function:
Linear algebra is a branch of mathematics concerned with vector spaces and the mappings between those spaces. NumPy has a package called linalg that supports powerful linear algebra functions. We can use these functions to find eigenvalues and eigenvectors or to perform singular value decomposition:
The following table will summarise some commonly used functions in the numpy.linalg
package:
Function |
Description |
Example |
---|---|---|
|
Calculate the dot product of two arrays |
>>> a = np.array([[1, 0],[0, 1]]) >>> b = np.array( [[4, 1],[2, 2]]) >>> np.dot(a,b) array([[4, 1],[2, 2]])
|
|
Calculate the inner and outer product of two arrays |
>>> a = np.array([1, 1, 1]) >>> b = np.array([3, 5, 1]) >>> np.inner(a,b) 9
|
|
Find a matrix or vector norm |
>>> a = np.arange(3) >>> np.linalg.norm(a) 2.23606
|
|
Compute the determinant of an array |
>>> a = np.array([[1,2],[3,4]]) >>> np.linalg.det(a) -2.0
|
|
Compute the inverse of a matrix |
>>> a = np.array([[1,2],[3,4]]) >>> np.linalg.inv(a) array([[-2., 1.],[1.5, -0.5]])
|
|
Calculate the QR decomposition |
>>> a = np.array([[1,2],[3,4]]) >>> np.linalg.qr(a) (array([[0.316, 0.948], [0.948, 0.316]]), array([[ 3.162, 4.427], [ 0., 0.632]]))
|
|
Compute the condition number of a matrix |
>>> a = np.array([[1,3],[2,4]]) >>> np.linalg.cond(a) 14.933034
|
|
Compute the sum of the diagonal element |
>>> np.trace(np.arange(6)). reshape(2,3)) 4
|
An important part of any simulation is the ability to generate random numbers. For this purpose, NumPy provides various routines in the submodule random
. It uses a particular algorithm, called the Mersenne Twister, to generate pseudorandom numbers.
An array of random numbers in the [0.0, 1.0]
interval can be generated as follows:
NumPy also provides for many other distributions, including the Beta
, bionomial
, chi
-square
, Dirichlet
, exponential
, F
, Gamma
, geometric
, or Gumbel
.
Function |
Description |
Example |
---|---|---|
|
Draw samples from a binomial distribution (n: number of trials, p: probability) |
>>> n, p = 100, 0.2 >>> np.random.binomial(n, p, 3) array([17, 14, 23])
|
|
Draw samples using a Dirichlet distribution |
>>> np.random.dirichlet(alpha=(2,3), size=3) array([[0.519, 0.480], [0.639, 0.36], [0.838, 0.161]])
|
|
Draw samples from a Poisson distribution |
>>> np.random.poisson(lam=2, size= 2) array([4,1])
|
|
Draw samples using a normal Gaussian distribution |
>>> np.random.normal (loc=2.5, scale=0.3, size=3) array([2.4436, 2.849, 2.741)
|
|
Draw samples using a uniform distribution |
>>> np.random.uniform( low=0.5, high=2.5, size=3) array([1.38, 1.04, 2.19[)
|
The following figure shows two distributions, binomial
and poisson
, side by side with various parameters (the visualization was created with matplotlib
, which will be covered in Chapter 4, Data Visualization):
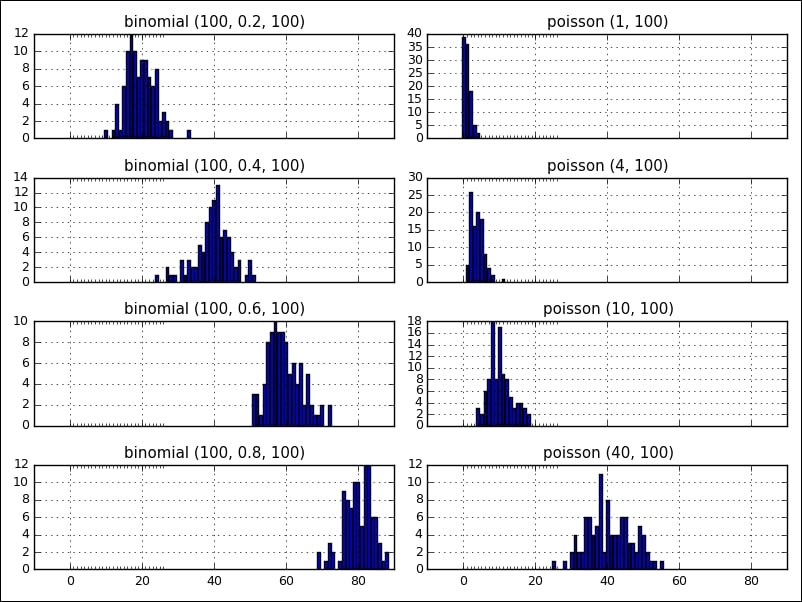
Then, we are getting familiar with some common functions and operations on ndarray
.
Exercise 2: What is the difference between np.dot(a, b)
and (a*b)
?
Pandas is a Python package that supports fast, flexible, and expressive data structures, as well as computing functions for data analysis. The following are some prominent features that Pandas supports:
- Data structure with labeled axes. This makes the program clean and clear and avoids common errors from misaligned data.
- Flexible handling of missing data.
- Intelligent label-based slicing, fancy indexing, and subset creation of large datasets.
- Powerful arithmetic operations and statistical computations on a custom axis via axis label.
- Robust input and output support for loading or saving data from and to files, databases, or HDF5 format.
Related to Pandas installation, we recommend an easy way, that is to install it as a part of Anaconda, a cross-platform distribution for data analysis and scientific computing. You can refer to the reference at http://docs.continuum.io/anaconda/ to download and install the library.
After installation, we can use it like other Python packages. Firstly, we have to import the following packages at the beginning of the program:
>>> import pandas as pd >>> import numpy as np
Let's first get acquainted with two of Pandas' primary data structures: the Series and the DataFrame. They can handle the majority of use cases in finance, statistic, social science, and many areas of engineering.
A Series is a one-dimensional object similar to an array, list, or column in table. Each item in a Series is assigned to an entry in an index:
We can access the value of a Series by using the index:
Sometimes, we want to filter or rename the index of a Series created from a Python dictionary. At such times, we can pass the selected index list directly to the initial function, similarly to the process in the above example. Only elements that exist in the index list will be in the Series object. Conversely, indexes that are missing in the dictionary are initialized to default NaN
values by Pandas:
The library also supports functions that detect missing data:
Similarly, we can also initialize a Series from a scalar value:
The DataFrame is a tabular data structure comprising a set of ordered columns and rows. It can be thought of as a group of Series objects that share an index (the column names). There are a number of ways to initialize a DataFrame object. Firstly, let's take a look at the common example of creating DataFrame from a dictionary of lists:
We can provide the index labels of a DataFrame similar to a Series:
We can construct a DataFrame out of nested lists as well:
Columns can be accessed by column name as a Series can, either by dictionary-like notation or as an attribute, if the column name is a syntactically valid attribute name:
Using a couple of methods, rows can be retrieved by position or name:
Another common case is to provide a DataFrame with data from a location such as a text file. In this situation, we use the read_csv
function that expects the column separator to be a comma, by default. However, we can change that by using the sep
parameter:
sep
: This is a delimiter between columns. The default is comma symbol.dtype
: This is a data type for data or columns.header
: This sets row numbers to use as the column names.skiprows
: This skips line numbers to skip at the start of the file.error_bad_lines
: This shows invalid lines (too many fields) that will, by default, cause an exception, such that no DataFrame will be returned. If we set the value of this parameter asfalse
, the bad lines will be skipped.
Series is a one-dimensional object similar to an array, list, or column in table. Each item in a Series is assigned to an entry in an index:
We can access the value of a Series by using the index:
Sometimes, we want to filter or rename the index of a Series created from a Python dictionary. At such times, we can pass the selected index list directly to the initial function, similarly to the process in the above example. Only elements that exist in the index list will be in the Series object. Conversely, indexes that are missing in the dictionary are initialized to default NaN
values by Pandas:
The library also supports functions that detect missing data:
Similarly, we can also initialize a Series from a scalar value:
The DataFrame is a tabular data structure comprising a set of ordered columns and rows. It can be thought of as a group of Series objects that share an index (the column names). There are a number of ways to initialize a DataFrame object. Firstly, let's take a look at the common example of creating DataFrame from a dictionary of lists:
We can provide the index labels of a DataFrame similar to a Series:
We can construct a DataFrame out of nested lists as well:
Columns can be accessed by column name as a Series can, either by dictionary-like notation or as an attribute, if the column name is a syntactically valid attribute name:
Using a couple of methods, rows can be retrieved by position or name:
Another common case is to provide a DataFrame with data from a location such as a text file. In this situation, we use the read_csv
function that expects the column separator to be a comma, by default. However, we can change that by using the sep
parameter:
sep
: This is a delimiter between columns. The default is comma symbol.dtype
: This is a data type for data or columns.header
: This sets row numbers to use as the column names.skiprows
: This skips line numbers to skip at the start of the file.error_bad_lines
: This shows invalid lines (too many fields) that will, by default, cause an exception, such that no DataFrame will be returned. If we set the value of this parameter asfalse
, the bad lines will be skipped.
We can provide the index labels of a DataFrame similar to a Series:
We can construct a DataFrame out of nested lists as well:
Columns can be accessed by column name as a Series can, either by dictionary-like notation or as an attribute, if the column name is a syntactically valid attribute name:
Using a couple of methods, rows can be retrieved by position or name:
Another common case is to provide a DataFrame with data from a location such as a text file. In this situation, we use the read_csv
function that expects the column separator to be a comma, by default. However, we can change that by using the sep
parameter:
sep
: This is a delimiter between columns. The default is comma symbol.dtype
: This is a data type for data or columns.header
: This sets row numbers to use as the column names.skiprows
: This skips line numbers to skip at the start of the file.error_bad_lines
: This shows invalid lines (too many fields) that will, by default, cause an exception, such that no DataFrame will be returned. If we set the value of this parameter asfalse
, the bad lines will be skipped.
Pandas supports many essential functionalities that are useful to manipulate Pandas data structures. In this book, we will focus on the most important features regarding exploration and analysis.
Reindex is a critical method in the Pandas data structures. It confirms whether the new or modified data satisfies a given set of labels along a particular axis of Pandas object.
First, let's view a reindex
example on a Series object:
Argument |
Description |
---|---|
|
This is the new labels/index to conform to. |
|
This is the method to use for filling holes in a
|
|
This return a new object. The default setting is |
|
The matches index values on the passed multiple index level. |
|
This is the value to use for missing values. The default setting is |
|
This is the maximum size gap to fill in |
In common data analysis situations, our data structure objects contain many columns and a large number of rows. Therefore, we cannot view or load all information of the objects. Pandas supports functions that allow us to inspect a small sample. By default, the functions return five elements, but we can set a custom number as well. The following example shows how to display the first five and the last three rows of a longer Series:
We can also use these functions for DataFrame objects in the same way.
Firstly, we will consider arithmetic operations between objects. In different indexes objects case, the expected result will be the union of the index pairs. We will not explain this again because we had an example about it in the above section (s5 + s6
). This time, we will show another example with a DataFrame:
The mechanisms for returning the result between two kinds of data structure are similar. A problem that we need to consider is the missing data between objects. In this case, if we want to fill with a fixed value, such as 0
, we can use the arithmetic functions such as add
, sub
, div
, and mul
, and the function's supported parameters such as fill_value
:
Next, we will discuss comparison
operations between data objects. We have some supported functions such as
equal (eq), not equal (ne), greater than (gt), less than (lt), less equal (le), and greater equal (ge). Here is an example:
The supported statistics method of a library is really important in data analysis. To get inside a big data object, we need to know some summarized information such as mean, sum, or quantile. Pandas supports a large number of methods to compute them. Let's consider a simple example of calculating the sum
information of df5
, which is a DataFrame object:
When we do not specify which axis we want to calculate sum
information, by default, the function will calculate on index axis, which is axis 0
:
Here, we have a summary table for common supported statistics functions in Pandas:
Function |
Description |
---|---|
|
This compute the index labels with the minimum or maximum corresponding values. |
|
This compute the frequency of unique values. |
|
This return the number of non-null values in a data object. |
|
This return mean, median, minimum, and maximum values of an axis in a data object. |
|
These return the standard deviation, variance, and standard error of mean. |
|
This gets the absolute value of a data object. |
Pandas supports function application that allows us to apply some functions supported in other packages such as NumPy or our own functions on data structure objects. Here, we illustrate two examples of these cases, firstly, using apply
to execute the std()
function, which is the standard deviation calculating function of the NumPy package:
- Define the function or formula that you want to apply on a data object.
- Call the defined function or formula via
apply
. In this step, we also need to figure out the axis that we want to apply the calculation to:>>> f = lambda x: x.max() – x.min() # step 1 >>> df5.apply(f, axis=1) # step 2 0 2 1 2 2 2 dtype: int64 >>> def sigmoid(x): return 1/(1 + np.exp(x)) >>> df5.apply(sigmoid) a b c 0 0.500000 0.268941 0.119203 1 0.047426 0.017986 0.006693 2 0.002473 0.000911 0.000335
is a critical method in the Pandas data structures. It confirms whether the new or modified data satisfies a given set of labels along a particular axis of Pandas object.
First, let's view a reindex
example on a Series object:
Argument |
Description |
---|---|
|
This is the new labels/index to conform to. |
|
This is the method to use for filling holes in a
|
|
This return a new object. The default setting is |
|
The matches index values on the passed multiple index level. |
|
This is the value to use for missing values. The default setting is |
|
This is the maximum size gap to fill in |
In common data analysis situations, our data structure objects contain many columns and a large number of rows. Therefore, we cannot view or load all information of the objects. Pandas supports functions that allow us to inspect a small sample. By default, the functions return five elements, but we can set a custom number as well. The following example shows how to display the first five and the last three rows of a longer Series:
We can also use these functions for DataFrame objects in the same way.
Firstly, we will consider arithmetic operations between objects. In different indexes objects case, the expected result will be the union of the index pairs. We will not explain this again because we had an example about it in the above section (s5 + s6
). This time, we will show another example with a DataFrame:
The mechanisms for returning the result between two kinds of data structure are similar. A problem that we need to consider is the missing data between objects. In this case, if we want to fill with a fixed value, such as 0
, we can use the arithmetic functions such as add
, sub
, div
, and mul
, and the function's supported parameters such as fill_value
:
Next, we will discuss comparison
operations between data objects. We have some supported functions such as
equal (eq), not equal (ne), greater than (gt), less than (lt), less equal (le), and greater equal (ge). Here is an example:
The supported statistics method of a library is really important in data analysis. To get inside a big data object, we need to know some summarized information such as mean, sum, or quantile. Pandas supports a large number of methods to compute them. Let's consider a simple example of calculating the sum
information of df5
, which is a DataFrame object:
When we do not specify which axis we want to calculate sum
information, by default, the function will calculate on index axis, which is axis 0
:
Here, we have a summary table for common supported statistics functions in Pandas:
Function |
Description |
---|---|
|
This compute the index labels with the minimum or maximum corresponding values. |
|
This compute the frequency of unique values. |
|
This return the number of non-null values in a data object. |
|
This return mean, median, minimum, and maximum values of an axis in a data object. |
|
These return the standard deviation, variance, and standard error of mean. |
|
This gets the absolute value of a data object. |
Pandas supports function application that allows us to apply some functions supported in other packages such as NumPy or our own functions on data structure objects. Here, we illustrate two examples of these cases, firstly, using apply
to execute the std()
function, which is the standard deviation calculating function of the NumPy package:
- Define the function or formula that you want to apply on a data object.
- Call the defined function or formula via
apply
. In this step, we also need to figure out the axis that we want to apply the calculation to:>>> f = lambda x: x.max() – x.min() # step 1 >>> df5.apply(f, axis=1) # step 2 0 2 1 2 2 2 dtype: int64 >>> def sigmoid(x): return 1/(1 + np.exp(x)) >>> df5.apply(sigmoid) a b c 0 0.500000 0.268941 0.119203 1 0.047426 0.017986 0.006693 2 0.002473 0.000911 0.000335
Firstly, we will consider arithmetic operations between objects. In different indexes objects case, the expected result will be the union of the index pairs. We will not explain this again because we had an example about it in the above section (s5 + s6
). This time, we will show another example with a DataFrame:
The mechanisms for returning the result between two kinds of data structure are similar. A problem that we need to consider is the missing data between objects. In this case, if we want to fill with a fixed value, such as 0
, we can use the arithmetic functions such as add
, sub
, div
, and mul
, and the function's supported parameters such as fill_value
:
Next, we will discuss comparison
operations between data objects. We have some supported functions such as
equal (eq), not equal (ne), greater than (gt), less than (lt), less equal (le), and greater equal (ge). Here is an example:
The supported statistics method of a library is really important in data analysis. To get inside a big data object, we need to know some summarized information such as mean, sum, or quantile. Pandas supports a large number of methods to compute them. Let's consider a simple example of calculating the sum
information of df5
, which is a DataFrame object:
When we do not specify which axis we want to calculate sum
information, by default, the function will calculate on index axis, which is axis 0
:
Here, we have a summary table for common supported statistics functions in Pandas:
Function |
Description |
---|---|
|
This compute the index labels with the minimum or maximum corresponding values. |
|
This compute the frequency of unique values. |
|
This return the number of non-null values in a data object. |
|
This return mean, median, minimum, and maximum values of an axis in a data object. |
|
These return the standard deviation, variance, and standard error of mean. |
|
This gets the absolute value of a data object. |
Pandas supports function application that allows us to apply some functions supported in other packages such as NumPy or our own functions on data structure objects. Here, we illustrate two examples of these cases, firstly, using apply
to execute the std()
function, which is the standard deviation calculating function of the NumPy package:
- Define the function or formula that you want to apply on a data object.
- Call the defined function or formula via
apply
. In this step, we also need to figure out the axis that we want to apply the calculation to:>>> f = lambda x: x.max() – x.min() # step 1 >>> df5.apply(f, axis=1) # step 2 0 2 1 2 2 2 dtype: int64 >>> def sigmoid(x): return 1/(1 + np.exp(x)) >>> df5.apply(sigmoid) a b c 0 0.500000 0.268941 0.119203 1 0.047426 0.017986 0.006693 2 0.002473 0.000911 0.000335
The mechanisms for returning the result between two kinds of data structure are similar. A problem that we need to consider is the missing data between objects. In this case, if we want to fill with a fixed value, such as 0
, we can use the arithmetic functions such as add
, sub
, div
, and mul
, and the function's supported parameters such as fill_value
:
Next, we will discuss comparison
operations between data objects. We have some supported functions such as
equal (eq), not equal (ne), greater than (gt), less than (lt), less equal (le), and greater equal (ge). Here is an example:
The supported statistics method of a library is really important in data analysis. To get inside a big data object, we need to know some summarized information such as mean, sum, or quantile. Pandas supports a large number of methods to compute them. Let's consider a simple example of calculating the sum
information of df5
, which is a DataFrame object:
When we do not specify which axis we want to calculate sum
information, by default, the function will calculate on index axis, which is axis 0
:
Here, we have a summary table for common supported statistics functions in Pandas:
Function |
Description |
---|---|
|
This compute the index labels with the minimum or maximum corresponding values. |
|
This compute the frequency of unique values. |
|
This return the number of non-null values in a data object. |
|
This return mean, median, minimum, and maximum values of an axis in a data object. |
|
These return the standard deviation, variance, and standard error of mean. |
|
This gets the absolute value of a data object. |
Pandas supports function application that allows us to apply some functions supported in other packages such as NumPy or our own functions on data structure objects. Here, we illustrate two examples of these cases, firstly, using apply
to execute the std()
function, which is the standard deviation calculating function of the NumPy package:
- Define the function or formula that you want to apply on a data object.
- Call the defined function or formula via
apply
. In this step, we also need to figure out the axis that we want to apply the calculation to:>>> f = lambda x: x.max() – x.min() # step 1 >>> df5.apply(f, axis=1) # step 2 0 2 1 2 2 2 dtype: int64 >>> def sigmoid(x): return 1/(1 + np.exp(x)) >>> df5.apply(sigmoid) a b c 0 0.500000 0.268941 0.119203 1 0.047426 0.017986 0.006693 2 0.002473 0.000911 0.000335
supported statistics method of a library is really important in data analysis. To get inside a big data object, we need to know some summarized information such as mean, sum, or quantile. Pandas supports a large number of methods to compute them. Let's consider a simple example of calculating the sum
information of df5
, which is a DataFrame object:
When we do not specify which axis we want to calculate sum
information, by default, the function will calculate on index axis, which is axis 0
:
Here, we have a summary table for common supported statistics functions in Pandas:
Function |
Description |
---|---|
|
This compute the index labels with the minimum or maximum corresponding values. |
|
This compute the frequency of unique values. |
|
This return the number of non-null values in a data object. |
|
This return mean, median, minimum, and maximum values of an axis in a data object. |
|
These return the standard deviation, variance, and standard error of mean. |
|
This gets the absolute value of a data object. |
Pandas supports function application that allows us to apply some functions supported in other packages such as NumPy or our own functions on data structure objects. Here, we illustrate two examples of these cases, firstly, using apply
to execute the std()
function, which is the standard deviation calculating function of the NumPy package:
- Define the function or formula that you want to apply on a data object.
- Call the defined function or formula via
apply
. In this step, we also need to figure out the axis that we want to apply the calculation to:>>> f = lambda x: x.max() – x.min() # step 1 >>> df5.apply(f, axis=1) # step 2 0 2 1 2 2 2 dtype: int64 >>> def sigmoid(x): return 1/(1 + np.exp(x)) >>> df5.apply(sigmoid) a b c 0 0.500000 0.268941 0.119203 1 0.047426 0.017986 0.006693 2 0.002473 0.000911 0.000335
- Define the function or formula that you want to apply on a data object.
- Call the defined function or formula via
apply
. In this step, we also need to figure out the axis that we want to apply the calculation to:>>> f = lambda x: x.max() – x.min() # step 1 >>> df5.apply(f, axis=1) # step 2 0 2 1 2 2 2 dtype: int64 >>> def sigmoid(x): return 1/(1 + np.exp(x)) >>> df5.apply(sigmoid) a b c 0 0.500000 0.268941 0.119203 1 0.047426 0.017986 0.006693 2 0.002473 0.000911 0.000335
In this section, we will focus on how to get, set, or slice subsets of Pandas data structure objects. As we learned in previous sections, Series or DataFrame objects have axis labeling information. This information can be used to identify items that we want to select or assign a new value to in the object:
If the data object is a DataFrame structure, we can also proceed in a similar way:
For label indexing on the rows of DataFrame, we use the ix
function that enables us to select a set of rows and columns in the object. There are two parameters that we need to specify: the row
and column
labels that we want to get. By default, if we do not specify the selected column names, the function will return selected rows with all columns in the object:
Method |
Description |
---|---|
|
This selects a single row or column by integer location. |
|
This selects or sets a single value of a data object by row or column label. |
|
This selects a single column or row as a Series by label. |
Let's start with correlation and covariance computation between two data objects. Both the Series and DataFrame have a cov
method. On a DataFrame object, this method will compute the covariance between the Series inside the object:
We also have the corrwith
function that supports calculating correlations between Series that have the same label contained in different DataFrame objects:
In this section, we will discuss missing, NaN
, or null
values, in Pandas data structures. It is a very common situation to arrive with missing data in an object. One such case that creates missing data is reindexing:
>>> df10.isnull() a b c 3 False False False 2 False False False a True True True 0 False False False
On a Series, we can drop all null
data and index values by using the dropna
function:
Another way to control missing values is to use the supported parameters of functions that we introduced in the previous section. They are also very useful to solve this problem. In our experience, we should assign a fixed value in missing cases when we create data objects. This will make our objects cleaner in later processing steps. For example, consider the following:
We can alse use the fillna
function to fill a custom value in missing values:
In this section we will consider some advanced Pandas use cases.
Hierarchical indexing provides us with a way to work with higher dimensional data in a lower dimension by structuring the data object into multiple index levels on an axis:
In the preceding example, we have a Series object that has two index levels. The object can be rearranged into a DataFrame using the unstack
function. In an inverse situation, the stack
function can be used:
>>> s8.unstack() 0 1 a 0.549211 0.420874 b 0.051516 0.715021 c 0.503072 0.720772 d 0.373037 0.207026
We can also create a DataFrame to have a hierarchical index in both axes:
After grouping data into multiple index levels, we can also use most of the descriptive and statistics functions that have a level option, which can be used to specify the level we want to process:
The Panel is another data structure for three-dimensional data in Pandas. However, it is less frequently used than the Series or the DataFrame. You can think of a Panel as a table of DataFrame objects. We can create a Panel object from a 3D ndarray
or a dictionary of DataFrame objects:
Each item in a Panel is a DataFrame. We can select an item, by item name:
Alternatively, if we want to select data via an axis or data position, we can use the ix
method, like on Series or DataFrame:
In the preceding example, we have a Series object that has two index levels. The object can be rearranged into a DataFrame using the unstack
function. In an inverse situation, the stack
function can be used:
>>> s8.unstack() 0 1 a 0.549211 0.420874 b 0.051516 0.715021 c 0.503072 0.720772 d 0.373037 0.207026
We can also create a DataFrame to have a hierarchical index in both axes:
After grouping data into multiple index levels, we can also use most of the descriptive and statistics functions that have a level option, which can be used to specify the level we want to process:
The Panel is another data structure for three-dimensional data in Pandas. However, it is less frequently used than the Series or the DataFrame. You can think of a Panel as a table of DataFrame objects. We can create a Panel object from a 3D ndarray
or a dictionary of DataFrame objects:
Each item in a Panel is a DataFrame. We can select an item, by item name:
Alternatively, if we want to select data via an axis or data position, we can use the ix
method, like on Series or DataFrame:
The link https://www.census.gov/2010census/csv/pop_change.csv contains an US census dataset. It has 23 columns and one row for each US state, as well as a few rows for macro regions such as North, South, and West.
- Get this dataset into a Pandas DataFrame. Hint: just skip those rows that do not seem helpful, such as comments or description.
- While the dataset contains change metrics for each decade, we are interested in the population change during the second half of the twentieth century, that is between, 1950 and 2000. Which region has seen the biggest and the smallest population growth in this time span? Also, which US state?
Advanced open-ended exercise:
- Find more census data on the internet; not just on the US but on the world's countries. Try to find GDP data for the same time as well. Try to align this data to explore patterns. How are GDP and population growth related? Are there any special cases. such as countries with high GDP but low population growth or countries with the opposite history?
The easiest way to get started with plotting using matplotlib is often by using the MATLAB API that is supported by the package:
The output for the preceding command is as follows:
The preceding example could then be written as follows:
The output for the preceding command is as follows:
The output for the preceding command is as follows:
The default line format when we plot data in matplotlib is a solid blue line, which is abbreviated as b-
. To change this setting, we only need to add the symbol code, which includes letters as color string and symbols as line style string, to the plot
function. Let us consider a plot of several lines with different format styles:
The output for the preceding command is as follows:
The output for the preceding command is as follows:
The following table lists some common properties of the line2d
plotting:
Property |
Value type |
Description |
---|---|---|
|
Any matplotlib color |
This sets the color of the line in the figure |
|
On/off |
This sets the sequence of ink in the points |
|
|
This sets the data used for visualization |
|
[ |
This sets the line style in the figure |
|
Float value in points |
This sets the width of line in the figure |
|
Any symbol |
This sets the style at data points in the figure |
By default, all plotting commands apply to the current figure and axes. In some situations, we want to visualize data in multiple figures and axes to compare different plots or to use the space on a page more efficiently. There are two steps required before we can plot the data. Firstly, we have to define which figure we want to plot. Secondly, we need to figure out the position of our subplot in the figure:
The output for the preceding command is as follows:
The output for the preceding command is as follows:
There is a convenience method, plt.subplots()
, to creating a figure that contains a given number of subplots. As inthe previous example, we can use the plt.subplots(2,2)
command to create a 2x2
figure that consists of four subplots.
We have looked at how to create simple line plots so far. The matplotlib library supports many more plot types that are useful for data visualization. However, our goal is to provide the basic knowledge that will help you to understand and use the library for visualizing data in the most common situations. Therefore, we will only focus on four kinds of plot types: scatter plots, bar plots, contour plots, and histograms.
A scatter plot is used to visualize the relationship between variables measured in the same dataset. It is easy to plot a simple scatter plot, using the plt.scatter()
function, that requires numeric columns for both the x
and y
axis:
A bar plot is used to present grouped data with rectangular bars, which can be either vertical or horizontal, with the lengths of the bars corresponding to their values. We use the plt.bar()
command to visualize a vertical bar, and the plt.barh()
command for the other:
We use contour plots to present the relationship between three numeric variables in two dimensions. Two variables are drawn along the x
and y
axes, and the third variable, z
, is used for contour levels that are plotted as curves in different colors:
Let's take a look at the contour plot in the following image:
scatter plot is used to visualize the relationship between variables measured in the same dataset. It is easy to plot a simple scatter plot, using the plt.scatter()
function, that requires numeric columns for both the x
and y
axis:
A bar plot is used to present grouped data with rectangular bars, which can be either vertical or horizontal, with the lengths of the bars corresponding to their values. We use the plt.bar()
command to visualize a vertical bar, and the plt.barh()
command for the other:
We use contour plots to present the relationship between three numeric variables in two dimensions. Two variables are drawn along the x
and y
axes, and the third variable, z
, is used for contour levels that are plotted as curves in different colors:
Let's take a look at the contour plot in the following image:
bar plot is used to present grouped data with rectangular bars, which can be either vertical or horizontal, with the lengths of the bars corresponding to their values. We use the plt.bar()
command to visualize a vertical bar, and the plt.barh()
command for the other:
We use contour plots to present the relationship between three numeric variables in two dimensions. Two variables are drawn along the x
and y
axes, and the third variable, z
, is used for contour levels that are plotted as curves in different colors:
Let's take a look at the contour plot in the following image:
contour plots to present the relationship between three numeric variables in two dimensions. Two variables are drawn along the x
and y
axes, and the third variable, z
, is used for contour levels that are plotted as curves in different colors:
Let's take a look at the contour plot in the following image:
Legends are an important element that is used to identify the plot
elements in a figure. The easiest way to show a legend inside a figure is to use the label
argument of the plot
function, and show the labels by calling the plt.legend()
method:
The output for the preceding command as follows:
The loc
argument in the legend command is used to figure out the position of the label box. There are several valid location options: lower left
, right
, upper left
, lower center
, upper right
, center
, lower right
, upper right
, center right
, best
, upper center
, and center left
. The default position setting is upper right
. However, when we set an invalid location option that does not exist in the above list, the function automatically falls back to the best option.
The output for the preceding command is as follows:
The other element in a figure that we want to introduce is the annotations which can consist of text, arrows, or other shapes to explain parts of the figure in detail, or to emphasize some special data points. There are different methods for showing annotations, such as text
, arrow
, and
annotation
.
- The
text
method draws text at the given coordinates(x, y)
on the plot; optionally with custom properties. There are some common arguments in the function:x
,y
, label text, and font-related properties that can be passed in viafontdict
, such asfamily
,fontsize
, andstyle
. - The
annotate
method can draw both text and arrows arranged appropriately. Arguments of this function ares
(label text),xy
(the position of element to annotation),xytext
(the position of the labels
),xycoords
(the string that indicates what type of coordinatexy
is), andarrowprops
(the dictionary of line properties for the arrow that connects the annotation).
Here is a simple example to illustrate the annotate
and text
functions:
We have covered most of the important components in a plot figure using matplotlib. In this section, we will introduce another powerful plotting method for directly creating standard visualization from Pandas data objects that are often used to manipulate data.
The output for the preceding command is as follows:
Another example will visualize the data of a DataFrame object consisting of multiple columns:
The output for the preceding command is as follows:
The plot method of the DataFrame has a number of options that allow us to handle the plotting of the columns. For example, in the above DataFrame visualization, we chose to plot the columns in separate subplots. The following table lists more options:
Argument |
Value |
Description |
---|---|---|
|
|
The plots each data column in a separate subplot |
|
|
The gets a log-scale |
|
|
The plots data on a secondary |
|
|
The shares the same |
Besides matplotlib, there are other powerful data visualization toolkits based on Python. While we cannot dive deeper into these libraries, we would like to at least briefly introduce them in this session.
Bokeh is a project by Peter Wang, Hugo Shi, and others at Continuum Analytics. It aims to provide elegant and engaging visualizations in the style of D3.js
. The library can quickly and easily create interactive plots, dashboards, and data applications. Here are a few differences between matplotlib and Bokeh:
- Bokeh achieves cross-platform ubiquity through IPython's new model of in-browser client-side rendering
- Bokeh uses a syntax familiar to R and ggplot users, while matplotlib is more familiar to Matlab users
- Bokeh has a coherent vision to build a ggplot-inspired in-browser interactive visualization tool, while Matplotlib has a coherent vision of focusing on 2D cross-platform graphics.
The basic steps for creating plots with Bokeh are as follows:
- Prepare some data in a list, series, and Dataframe
- Tell Bokeh where you want to generate the output
- Call
figure()
to create a plot with some overall options, similar to the matplotlib options discussed earlier - Add renderers for your data, with visual customizations such as colors, legends, and width
- Ask Bokeh to
show()
orsave()
the results
MayaVi is a library for interactive scientific data visualization and 3D plotting, built on top of the award-winning visualization toolkit (VTK), which is a traits-based wrapper for the open-source visualization library. It offers the following:
- The possibility to interact with the data and object in the visualization through dialogs.
- An interface in Python for scripting. MayaVi can work with Numpy and scipy for 3D plotting out of the box and can be used within IPython notebooks, which is similar to matplotlib.
- An abstraction over VTK that offers a simpler programming model.
Let's view an illustration made entirely using MayaVi based on VTK examples and their provided data:
a project by Peter Wang, Hugo Shi, and others at Continuum Analytics. It aims to provide elegant and engaging visualizations in the style of D3.js
. The library can quickly and easily create interactive plots, dashboards, and data applications. Here are a few differences between matplotlib and Bokeh:
- Bokeh achieves cross-platform ubiquity through IPython's new model of in-browser client-side rendering
- Bokeh uses a syntax familiar to R and ggplot users, while matplotlib is more familiar to Matlab users
- Bokeh has a coherent vision to build a ggplot-inspired in-browser interactive visualization tool, while Matplotlib has a coherent vision of focusing on 2D cross-platform graphics.
The basic steps for creating plots with Bokeh are as follows:
- Prepare some data in a list, series, and Dataframe
- Tell Bokeh where you want to generate the output
- Call
figure()
to create a plot with some overall options, similar to the matplotlib options discussed earlier - Add renderers for your data, with visual customizations such as colors, legends, and width
- Ask Bokeh to
show()
orsave()
the results
MayaVi is a library for interactive scientific data visualization and 3D plotting, built on top of the award-winning visualization toolkit (VTK), which is a traits-based wrapper for the open-source visualization library. It offers the following:
- The possibility to interact with the data and object in the visualization through dialogs.
- An interface in Python for scripting. MayaVi can work with Numpy and scipy for 3D plotting out of the box and can be used within IPython notebooks, which is similar to matplotlib.
- An abstraction over VTK that offers a simpler programming model.
Let's view an illustration made entirely using MayaVi based on VTK examples and their provided data:
is a library for interactive scientific data visualization and 3D plotting, built on top of the award-winning visualization toolkit (VTK), which is a traits-based wrapper for the open-source visualization library. It offers the following:
- The possibility to interact with the data and object in the visualization through dialogs.
- An interface in Python for scripting. MayaVi can work with Numpy and scipy for 3D plotting out of the box and can be used within IPython notebooks, which is similar to matplotlib.
- An abstraction over VTK that offers a simpler programming model.
Let's view an illustration made entirely using MayaVi based on VTK examples and their provided data:
- Name two real or fictional datasets and explain which kind of plot would best fit the data: line plots, bar charts, scatter plots, contour plots, or histograms. Name one or two applications, where each of the plot type is common (for example, histograms are often used in image editing applications).
- We only focused on the most common plot types of matplotlib. After a bit of research, can you name a few more plot types that are available in matplotlib?
- Take one Pandas data structure from Chapter 3, Data Analysis with Pandas and plot the data in a suitable way. Then, save it as a PNG image to the disk.
In general, time series serve two purposes. First, they help us to learn about the underlying process that generated the data. On the other hand, we would like to be able to forecast future values of the same or related series using existing data. When we measure temperature, precipitation or wind, we would like to learn more about more complex things, such as weather or the climate of a region and how various factors interact. At the same time, we might be interested in weather forecasting.
Python supports date and time handling in the date time and time modules from the standard library:
Real-world data usually comes in all kinds of shapes and it would be great if we did not need to remember the exact date format specifies for parsing. Thankfully, Pandas abstracts away a lot of the friction, when dealing with strings representing dates or time. One of these helper functions is to_datetime
:
Which means they can be used interchangeably in many cases:
There are a few things to note here: We create a list of timestamp objects and pass it to the series constructor as index. This list of timestamps gets converted into a DatetimeIndex
on the fly. If we had passed only the date strings, we would not get a DatetimeIndex
, just an index
:
Pandas offer another great utility function for this task: date_range
.
The freq
attribute allows us to specify a multitude of options. Pandas has been used successfully in finance and economics, not least because it is really simple to work with business dates as well. As an example, to get an index with the first three business days of the millennium, the B
offset alias can be used:
The following table shows the available offset aliases and can be also be looked up in the Pandas documentation on time series under http://pandas.pydata.org/pandas-docs/stable/timeseries.html#offset-aliases:
Alias |
Description |
---|---|
B |
Business day frequency |
C |
Custom business day frequency |
D |
Calendar day frequency |
W |
Weekly frequency |
M |
Month end frequency |
BM |
Business month end frequency |
CBM |
Custom business month end frequency |
MS |
Month start frequency |
BMS |
Business month start frequency |
CBMS |
Custom business month start frequency |
Q |
Quarter end frequency |
BQ |
Business quarter frequency |
QS |
Quarter start frequency |
BQS |
Business quarter start frequency |
A |
Year end frequency |
BA |
Business year end frequency |
AS |
Year start frequency |
BAS |
Business year start frequency |
BH |
Business hour frequency |
H |
Hourly frequency |
T |
Minutely frequency |
S |
Secondly frequency |
L |
Milliseconds |
U |
Microseconds |
N |
Nanoseconds |
Moreover, the offset aliases can be used in combination as well. Here, we are generating a datetime
index with five elements, each one day, one hour, one minute and one second apart:
A custom definition of what a business hour means is also possible:
We can use this custom business hour to build indexes as well:
Finally, we can merge various indexes of different frequencies. The possibilities are endless. We only show one example, where we combine two indexes – each over a decade – one pointing to every first business day of a year and one to the last day of February:
A possible output of this plot is show in the following figure:
Just as with usual series objects, you can select parts and slice the index:
We can use date strings as keys, even though our series has a DatetimeIndex
:
Access is similar to lookup in dictionaries or lists, but more powerful. We can, for example, slice with strings or even mixed objects:
To see all entries from March until May, including:
Time series can be shifted forward or backward in time. The index stays in place, the values move:
Resampling describes the process of frequency conversion over time series data. It is a helpful technique in various circumstances as it fosters understanding by grouping together and aggregating data. It is possible to create a new time series from daily temperature data that shows the average temperature per week or month. On the other hand, real-world data may not be taken in uniform intervals and it is required to map observations into uniform intervals or to fill in missing values for certain points in time. These are two of the main use directions of resampling: binning and aggregation, and filling in missing data. Downsampling and upsampling occur in other fields as well, such as digital signal processing. There, the process of downsampling is often called decimation and performs a reduction of the sample rate. The inverse process is called interpolation, where the sample rate is increased. We will look at both directions from a data analysis angle.
Downsampling reduces the number of samples in the data. During this reduction, we are able to apply aggregations over data points. Let's imagine a busy airport with thousands of people passing through every hour. The airport administration has installed a visitor counter in the main area, to get an impression of exactly how busy their airport is.
Or we can reduce the sampling interval even more by resampling to an hourly interval:
We can ask for other things as well. For example, what was the maximum number of people that passed through our airport within one hour:
If you specify a function by string, Pandas uses highly optimized versions.
While in our airport this metric might not be that valuable, we can compute it nonetheless:
In upsampling, the frequency of the time series is increased. As a result, we have more sample points than data points. One of the main questions is how to account for the entries in the series where we have no measurement.
Let's start with hourly data for a single day:
If we upsample to data points taken every 15 minutes, our time series will be extended with NaN
values:
With the limit
parameter, it is possible to control the number of missing values to be filled:
If you want to adjust the labels during resampling, you can use the loffset
keyword argument:
There is another way to fill in missing values. We could employ an algorithm to construct new data points that would somehow fit the existing points, for some definition of somehow. This process is called interpolation.
While, by default, Pandas objects are time zone unaware, many real-world applications will make use of time zones. As with working with time in general, time zones are no trivial matter: do you know which countries have daylight saving time and do you know when the time zone is switched in those countries? Thankfully, Pandas builds on the time zone capabilities of two popular and proven utility libraries for time and date handling: pytz
and dateutil
:
To supply time zone information, you can use the tz
keyword argument:
This works for ranges
as well:
Time zone objects can also be constructed beforehand:
To move a time zone aware object to other time zones, you can use the tz_convert
method:
Along with the powerful timestamp object, which acts as a building block for the DatetimeIndex
, there is another useful data structure, which has been introduced in Pandas 0.15 – the Timedelta. The Timedelta can serve as a basis for indices as well, in this case a TimedeltaIndex
.
Pandas comes with great support for plotting, and this holds true for time series data as well.
As a first example, let's take some monthly data and plot it:
The following figure shows an example time series plot:
We can overlay an aggregate plot over 2 and 5 years:
The following figure shows the resampled 2-year plot:
The following figure shows the resample 5-year plot:
We can pass the kind of chart to the plot
method as well. The return value of the plot
method is an AxesSubplot
, which allows us to customize many aspects of the plot. Here we are setting the label values on the X
axis to the year values from our time series:
Let's imagine we have four time series that we would like to plot simultaneously. We generate a matrix of 1000 × 4 random values and treat each column as a separated time series:
Text is a great medium and it's a simple way to exchange information. The following statement is taken from a quote attributed to Doug McIlroy: Write programs to handle text streams, because that is the universal interface.
In this section we will start reading and writing data from and to text files.
Normally, the raw data logs of a system are stored in multiple text files, which can accumulate a large amount of information over time. Thankfully, it is simple to interact with these kinds of files in Python.
Pandas supports a number of functions for reading data from a text file into a DataFrame object. The most simple one is the read_csv()
function. Let's start with a small example file:
We can also set a specific row as the caption row by using the header
that's equal to the index of the selected row. Similarly, when we want to use any column in the data file as the column index of DataFrame, we set index_col
to the name or index of the column. We again use the second data file example_data/ex_06-02.txt
to illustrate this:
Apart from those parameters, we still have a lot of useful ones that can help us load data files into Pandas objects more effectively. The following table shows some common parameters:
Parameter |
Value |
Description |
---|---|---|
|
Type name or dictionary of type of columns |
Sets the data type for data or columns. By default it will try to infer the most appropriate data type. |
|
List-like or integer |
The number of lines to skip (starting from 0). |
|
List-like or dict, default None |
Values to recognize as |
|
List |
A list of values to be converted to Boolean True as well. |
|
List |
A list of values to be converted to Boolean False as well. |
|
|
If the |
|
|
The thousands separator |
|
|
Limits the number of rows to read from the file. |
|
|
If set to True, a DataFrame is returned, even if an error occurred during parsing. |
Besides the read_csv()
function, we also have some other parsing functions in Pandas:
Function |
Description |
---|---|
|
Read the general delimited file into DataFrame |
|
Read a table of fixed-width formatted lines into DataFrame |
|
Read text from the clipboard and pass to |
In some situations, we cannot automatically parse data files from the disk using these functions. In that case, we can also open files and iterate through the reader, supported by the CSV module in the standard library:
We saw how to load data from a text file into a Pandas data structure. Now, we will learn how to export data from the data object of a program to a text file. Corresponding to the read_csv()
function, we also have the to_csv()
function, supported by Pandas. Let's see an example below:
The result will look like this:
If we want to skip the header line or index column when writing out data into a disk file, we can set a False
value to the header and index parameters:
We can read and write binary serialization of Python objects with the pickle module, which can be found in the standard library. Object serialization can be useful, if you work with objects that take a long time to create, like some machine learning models. By pickling such objects, subsequent access to this model can be made faster. It also allows you to distribute Python objects in a standardized way.
Pandas includes support for pickling out of the box. The relevant methods are the read_pickle()
and to_pickle()
functions to read and write data from and to files easily. Those methods will write data to disk in the pickle format, which is a convenient short-term storage format:
HDF5 is not a database, but a data model and file format. It is suited for write-one, read-many datasets. An HDF5 file includes two kinds of objects: data sets, which are array-like collections of data, and groups, which are folder-like containers what hold data sets and other groups. There are some interfaces for interacting with HDF5 format in Python, such as h5py
which uses familiar NumPy and Python constructs, such as dictionaries and NumPy array syntax. With h5py
, we have high-level interface to the HDF5 API which helps us to get started. However, in this book, we will introduce another library for this kind of format called PyTables, which works well with Pandas objects:
Objects stored in the HDF5 file can be retrieved by specifying the object keys:
Once we have finished interacting with the HDF5 file, we close it to release the file handle:
Many applications require more robust storage systems then text files, which is why many applications use databases to store data. There are many kinds of databases, but there are two broad categories: relational databases, which support a standard declarative language called SQL, and so called NoSQL databases, which are often able to work without a predefined schema and where a data instance is more properly described as a document, rather as a row.
The above snippet says that our MongoDB instance only has one database, named 'local'. If the databases and collections we point to do not exist, MongoDB will create them as necessary:
The df_ex2
is transposed and converted to a JSON string before loading into a dictionary. The insert()
function receives our created dictionary from df_ex2
and saves it to the collection.
If we want to list all data inside the collection, we can execute the following commands:
Sometimes, we want to delete data in MongdoDB. All we need to do is to pass a query to the remove()
method on the collection:
The following table shows methods that provide shortcuts to manipulate documents in MongoDB:
Update Method |
Description |
---|---|
|
Increment a numeric field |
|
Set certain fields to new values |
|
Remove a field from the document |
|
Append a value onto an array in the document |
|
Append several values onto an array in the document |
|
Add a value to an array, only if it does not exist |
|
Remove the last value of an array |
|
Remove all occurrences of a value from an array |
|
Remove all occurrences of any set of values from an array |
|
Rename a field |
|
Update a value by bitwise operation |
Redis is an advanced kind of key-value store where the values can be of different types: string, list, set, sorted set or hash. Redis stores data in memory like memcached but it can be persisted on disk, unlike memcached, which has no such option. Redis supports fast reads and writes, in the order of 100,000 set or get operations per second.
As a first step to storing data in Redis, we need to define which kind of data structure is suitable for our requirements. In this section, we will introduce four commonly used data structures in Redis: simple value, list, set and ordered set. Though data is stored into Redis in many different data structures, each value must be associated with a key.
This is the most basic kind of value in Redis. For every key in Redis, we also have a value that can have a data type, such as string, integer or double. Let's start with an example for setting and getting data to and from Redis:
In the second example, we show you another kind of value type, an integer:
We have a few methods for interacting with list values in Redis. The following example uses rpush()
and lrange()
functions to put and get list data to and from the DB:
Function |
Description |
---|---|
|
Push value onto the tail of the list name if name exists |
|
Remove and return the last item of the list name |
|
Set item at the index position of the list name to input value |
|
Push value on the head of the list name if name exists |
|
Remove and return the first item of the list name |
This data structure is also similar to the list type. However, in contrast to a list, we cannot store duplicate values in our set:
Corresponding to the list data structure, we also have a number of functions to get, set, update or delete items in the set. They are listed in the supported functions for set data structure, in the following table:
Function |
Description |
---|---|
|
Add value(s) to the set with key name |
|
Return the number of element in the set with key name |
|
Return all members of the set with key name |
|
Remove value(s) from the set with key name |
The ordered set data structure takes an extra attribute when we add data to a set called score. An ordered set will use the score to determine the order of the elements in the set:
By using the zrange(name, start, end)
function, we can get a range of values from the sorted set between the start and end score sorted in ascending order by default. If we want to change the way
method of sorting, we can set the desc
parameter to True
. The withscore
parameter is used in case we want to get the scores along with the return values. The return type is a list of (value, score) pairs as you can see in the above example.
See the below table for more functions available on ordered sets:
Function |
Description |
---|---|
|
Return the number of elements in the sorted set with key name |
|
Increment the score of value in the sorted set with key name by amount |
|
Return a range of values from the sorted set with key name with a score between min and max. If If start and |
|
Return a 0-based value indicating the rank of value in the sorted set with key name |
|
Remove member value(s) from the sorted set with key name |
We have a few methods for interacting with list values in Redis. The following example uses rpush()
and lrange()
functions to put and get list data to and from the DB:
Function |
Description |
---|---|
|
Push value onto the tail of the list name if name exists |
|
Remove and return the last item of the list name |
|
Set item at the index position of the list name to input value |
|
Push value on the head of the list name if name exists |
|
Remove and return the first item of the list name |
This data structure is also similar to the list type. However, in contrast to a list, we cannot store duplicate values in our set:
Corresponding to the list data structure, we also have a number of functions to get, set, update or delete items in the set. They are listed in the supported functions for set data structure, in the following table:
Function |
Description |
---|---|
|
Add value(s) to the set with key name |
|
Return the number of element in the set with key name |
|
Return all members of the set with key name |
|
Remove value(s) from the set with key name |
The ordered set data structure takes an extra attribute when we add data to a set called score. An ordered set will use the score to determine the order of the elements in the set:
By using the zrange(name, start, end)
function, we can get a range of values from the sorted set between the start and end score sorted in ascending order by default. If we want to change the way
method of sorting, we can set the desc
parameter to True
. The withscore
parameter is used in case we want to get the scores along with the return values. The return type is a list of (value, score) pairs as you can see in the above example.
See the below table for more functions available on ordered sets:
Function |
Description |
---|---|
|
Return the number of elements in the sorted set with key name |
|
Increment the score of value in the sorted set with key name by amount |
|
Return a range of values from the sorted set with key name with a score between min and max. If If start and |
|
Return a 0-based value indicating the rank of value in the sorted set with key name |
|
Remove member value(s) from the sorted set with key name |
Function |
Description |
---|---|
|
Push value onto the tail of the list name if name exists |
|
Remove and return the last item of the list name |
|
Set item at the index position of the list name to input value |
|
Push value on the head of the list name if name exists |
|
Remove and return the first item of the list name |
This data structure is also similar to the list type. However, in contrast to a list, we cannot store duplicate values in our set:
Corresponding to the list data structure, we also have a number of functions to get, set, update or delete items in the set. They are listed in the supported functions for set data structure, in the following table:
Function |
Description |
---|---|
|
Add value(s) to the set with key name |
|
Return the number of element in the set with key name |
|
Return all members of the set with key name |
|
Remove value(s) from the set with key name |
The ordered set data structure takes an extra attribute when we add data to a set called score. An ordered set will use the score to determine the order of the elements in the set:
By using the zrange(name, start, end)
function, we can get a range of values from the sorted set between the start and end score sorted in ascending order by default. If we want to change the way
method of sorting, we can set the desc
parameter to True
. The withscore
parameter is used in case we want to get the scores along with the return values. The return type is a list of (value, score) pairs as you can see in the above example.
See the below table for more functions available on ordered sets:
Function |
Description |
---|---|
|
Return the number of elements in the sorted set with key name |
|
Increment the score of value in the sorted set with key name by amount |
|
Return a range of values from the sorted set with key name with a score between min and max. If If start and |
|
Return a 0-based value indicating the rank of value in the sorted set with key name |
|
Remove member value(s) from the sorted set with key name |
Corresponding to the list data structure, we also have a number of functions to get, set, update or delete items in the set. They are listed in the supported functions for set data structure, in the following table:
Function |
Description |
---|---|
|
Add value(s) to the set with key name |
|
Return the number of element in the set with key name |
|
Return all members of the set with key name |
|
Remove value(s) from the set with key name |
The ordered set data structure takes an extra attribute when we add data to a set called score. An ordered set will use the score to determine the order of the elements in the set:
By using the zrange(name, start, end)
function, we can get a range of values from the sorted set between the start and end score sorted in ascending order by default. If we want to change the way
method of sorting, we can set the desc
parameter to True
. The withscore
parameter is used in case we want to get the scores along with the return values. The return type is a list of (value, score) pairs as you can see in the above example.
See the below table for more functions available on ordered sets:
Function |
Description |
---|---|
|
Return the number of elements in the sorted set with key name |
|
Increment the score of value in the sorted set with key name by amount |
|
Return a range of values from the sorted set with key name with a score between min and max. If If start and |
|
Return a 0-based value indicating the rank of value in the sorted set with key name |
|
Remove member value(s) from the sorted set with key name |
By using the zrange(name, start, end)
function, we can get a range of values from the sorted set between the start and end score sorted in ascending order by default. If we want to change the way
method of sorting, we can set the desc
parameter to True
. The withscore
parameter is used in case we want to get the scores along with the return values. The return type is a list of (value, score) pairs as you can see in the above example.
See the below table for more functions available on ordered sets:
Function |
Description |
---|---|
|
Return the number of elements in the sorted set with key name |
|
Increment the score of value in the sorted set with key name by amount |
|
Return a range of values from the sorted set with key name with a score between min and max. If If start and |
|
Return a 0-based value indicating the rank of value in the sorted set with key name |
|
Remove member value(s) from the sorted set with key name |
- Take a data set of your choice and design storage options for it. Consider text files, HDF5, a document database, and a data structure store as possible persistent options. Also evaluate how difficult (by some metric, for examples, how many lines of code) it would be to update or delete a specific item. Which storage type is the easiest to set up? Which storage type supports the most flexible queries?
- In Chapter 3, Data Analysis with Pandas we saw that it is possible to create hierarchical indices with Pandas. As an example, assume that you have data on each city with more than 1 million inhabitants and that we have a two level index, so we can address individual cities, but also whole countries. How would you represent this hierarchical relationship with the various storage options presented in this chapter: text files, HDF5, MongoDB, and Redis? What do you believe would be most convenient to work with in the long run?
Here are a few data preparation scenarios:
- A client hands you three files, each containing time series data about a single geological phenomenon, but the observed data is recorded on different intervals and uses different separators
- A machine learning algorithm can only work with numeric data, but your input only contains text labels
- You are handed the raw logs of a web server of an up and coming service and your task is to make suggestions on a growth strategy, based on existing visitor behavior
The arsenal of tools for data munging is huge, and while we will focus on Python we want to mention some useful tools as well. If they are available on your system and you expect to work a lot with data, they are worth learning.
We want to demonstrate the power of these tools with a single example only.
Each line of the log file contains an entry in the common log server format (you can download this data set from http://ita.ee.lbl.gov/html/contrib/EPA-HTTP.html):
$ cat epa-html.txt wpbfl2-45.gate.net [29:23:56:12] "GET /Access/ HTTP/1.0" 200 2376ebaca.icsi.net [30:00:22:20] "GET /Info.html HTTP/1.0" 200 884
For instance, we want to know how often certain users have visited our site.
We are interested in the first column only, since this is where the IP address or hostname can be found. After that, we need to count the number of occurrences of each host and finally display the results in a friendly way.
The sort
| uniq -c
stanza is our workhorse here: it sorts the data first and uniq -c
will save the number of occurrences along with the value. The sort -nr | head -15
is our formatting part; we sort numerically (-n
) and in reverse (-r
), and keep only the top 15 entries.
Putting it all together with pipes:
$ cut -d ' ' -f 1 epa-http.txt | sort | uniq -c | sort -nr | head -15 294 sandy.rtptok1.epa.gov 292 e659229.boeing.com 266 wicdgserv.wic.epa.gov 263 keyhole.es.dupont.com 248 dwilson.pr.mcs.net 176 oea4.r8stw56.epa.gov 174 macip26.nacion.co.cr 172 dcimsd23.dcimsd.epa.gov 167 www-b1.proxy.aol.com 158 piweba3y.prodigy.com 152 wictrn13.dcwictrn.epa.gov 151 nntp1.reach.com 151 inetg1.arco.com 149 canto04.nmsu.edu 146 weisman.metrokc.gov
With one command, we get to convert a sequential server log into an ordered list of the most common hosts that visited our site. We also see that we do not seem to have large differences in the number of visits among our top users.
There are more little helpful tools of which the following are just a tiny selection:
Most real-world data will have some defects and therefore will need to go through a cleaning step first. We start with a small file. Although this file contains only four rows, it will allow us to demonstrate the process up to a cleaned data set:
Pandas used the first row as header
, but this is not what we want:
This is better, but instead of numeric values, we would like to supply our own column names:
If we try to coerce the height
column into float values, Pandas will report an exception:
Now we can finally make the height column a bit more useful. We can assign it the updated version, which has the favored type:
We could use a default height, maybe a fixed value:
On the other hand, we could also use the average of the existing values:
Even if we have clean and probably correct data, we might want to use only parts of it or we might want to check for outliers. An outlier is an observation point that is distant from other observations because of variability or measurement errors. In both cases, we want to reduce the number of elements in our data set to make it more relevant for further processing.
In this example, we will try to find potential outliers. We will use the Europe Brent Crude Oil Spot Price as recorded by the U.S. Energy Information Administration. The raw Excel data is available from http://www.eia.gov/dnav/pet/hist_xls/rbrted.xls (it can be found in the second worksheet). We cleaned the data slightly (the cleaning process is part of an exercise at the end of this chapter) and will work with the following data frame, containing 7160 entries, ranging from 1987 to 2015:
>>> df.head() date price 0 1987-05-20 18.63 1 1987-05-21 18.45 2 1987-05-22 18.55 3 1987-05-25 18.60 4 1987-05-26 18.63 >>> df.tail() date price 7155 2015-08-04 49.08 7156 2015-08-05 49.04 7157 2015-08-06 47.80 7158 2015-08-07 47.54 7159 2015-08-10 48.30
While many people know about oil prices – be it from the news or the filling station – let us forget anything we know about it for a minute. We could first ask for the extremes:
>>> df[df.price==df.price.min()] date price 2937 1998-12-10 9.1 >>> df[df.price==df.price.max()] date price 5373 2008-07-03 143.95
Another way to find potential outliers would be to ask for values that deviate most from the mean. We can use the np.abs
function to calculate the deviation from the mean first:
>>> np.abs(df.price - df.price.mean()) 0 26.17137 1 26.35137 ... 7157 2.99863 7158 2.73863 7159 3.49863
We can now compare this deviation from a multiple – we choose 2.5 – of the standard deviation:
>>> import numpy as np >>> df[np.abs(df.price - df.price.mean()) > 2.5 * df.price.std()] date price 5354 2008-06-06 132.81 5355 2008-06-09 134.43 5356 2008-06-10 135.24 5357 2008-06-11 134.52 5358 2008-06-12 132.11 5359 2008-06-13 134.29 5360 2008-06-16 133.90 5361 2008-06-17 131.27 5363 2008-06-19 131.84 5364 2008-06-20 134.28 5365 2008-06-23 134.54 5366 2008-06-24 135.37 5367 2008-06-25 131.59 5368 2008-06-26 136.82 5369 2008-06-27 139.38 5370 2008-06-30 138.40 5371 2008-07-01 140.67 5372 2008-07-02 141.24 5373 2008-07-03 143.95 5374 2008-07-07 139.62 5375 2008-07-08 134.15 5376 2008-07-09 133.91 5377 2008-07-10 135.81 5378 2008-07-11 143.68 5379 2008-07-14 142.43 5380 2008-07-15 136.02 5381 2008-07-16 133.31 5382 2008-07-17 134.16
We see that those few days in summer 2008 must have been special. Sure enough, it is not difficult to find articles and essays with titles like Causes and Consequences of the Oil Shock of 2007–08. We have discovered a trace to these events solely by looking at the data.
We could ask the above question for each decade separately. We first make our data frame look more like a time series:
We could filter out the eighties:
We observe that within the data available (1987–1989), the fall of 1988 exhibits a slight spike in the oil prices. Similarly, during the nineties, we see that we have a larger deviation, in the fall of 1990:
The situation is common: you have multiple data sources, but in order to make statements about the content, you would rather combine them. Fortunately, Pandas' concatenation and merge functions abstract away most of the pain, when combining, joining, or aligning data. It does so in a highly optimized manner as well.
Sometimes, we won't care about the indices of the originating data frames:
We can add keys to create a hierarchical index.
Data frames resemble database tables. It is therefore not surprising that Pandas implements SQL-like join operations on them. What is positively surprising is that these operations are highly optimized and extremely fast:
A left, right and full join can be specified by the how
parameter:
The merge methods can be specified with the how parameter. The following table shows the methods in comparison with SQL:
Merge Method |
SQL Join Name |
Description |
---|---|---|
|
LEFT OUTER JOIN |
Use keys from the left frame only. |
|
RIGHT OUTER JOIN |
Use keys from the right frame only. |
|
FULL OUTER JOIN |
Use a union of keys from both frames. |
|
INNER JOIN |
Use an intersection of keys from both frames. |
We saw how to combine data frames but sometimes we have all the right data in a single data structure, but the format is impractical for certain tasks. We start again with some artificial weather data:
However, if we have to bring our data into form every time, we could be a little more effective, by creating a reshaped data frame first, having the dates as an index and the cities as columns.
As a final topic, we will look at ways to get a condensed view of data with aggregations. Pandas comes with a lot of aggregation functions built-in. We already saw the describe
function in Chapter 3, Data Analysis with Pandas. This works on parts of the data as well. We start with some artificial data again, containing measurements about the number of sunshine hours per city and date:
>>> df.head() country city date hours 0 Germany Hamburg 2015-06-01 8 1 Germany Hamburg 2015-06-02 10 2 Germany Hamburg 2015-06-03 9 3 Germany Hamburg 2015-06-04 7 4 Germany Hamburg 2015-06-05 3
To view a summary per city
, we use the describe
function on the grouped data set:
>>> df.groupby("city").describe() hours city Berlin count 10.000000 mean 6.000000 std 3.741657 min 0.000000 25% 4.000000 50% 6.000000 75% 9.750000 max 10.000000 Birmingham count 10.000000 mean 5.100000 std 2.078995 min 2.000000 25% 4.000000 50% 5.500000 75% 6.750000 max 8.000000
On certain data sets
, it can be useful to group by more than one attribute. We can get an overview about the sunny hours per country and date by passing in two column names:
>>> df.groupby(["country", "date"]).describe() hours country date France 2015-06-01 count 5.000000 mean 6.200000 std 1.095445 min 5.000000 25% 5.000000 50% 7.000000 75% 7.000000 max 7.000000 2015-06-02 count 5.000000 mean 3.600000 std 3.577709 min 0.000000 25% 0.000000 50% 4.000000 75% 6.000000 max 8.000000 UK 2015-06-07 std 3.872983 min 0.000000 25% 2.000000 50% 6.000000 75% 8.000000 max 9.000000
One typical workflow during data exploration looks as follows:
- You find a criterion that you want to use to group your data. Maybe you have GDP data for every country along with the continent and you would like to ask questions about the continents. These questions usually lead to some function applications- you might want to compute the mean GDP per continent. Finally, you want to store this data for further processing in a new data structure.
- We use a simpler example here. Imagine some fictional weather data about the number of sunny hours per day and city:
>>> df date city value 0 2000-01-03 London 6 1 2000-01-04 London 3 2 2000-01-05 London 4 3 2000-01-03 Mexico 3 4 2000-01-04 Mexico 9 5 2000-01-05 Mexico 8 6 2000-01-03 Mumbai 12 7 2000-01-04 Mumbai 9 8 2000-01-05 Mumbai 8 9 2000-01-03 Tokyo 5 10 2000-01-04 Tokyo 5 11 2000-01-05 Tokyo 6
- The
groups
attributes return a dictionary containing the unique groups and the corresponding values as axis labels:>>> df.groupby("city").groups {'London': [0, 1, 2], 'Mexico': [3, 4, 5], 'Mumbai': [6, 7, 8], 'Tokyo': [9, 10, 11]}
- Although the result of a
groupby
is a GroupBy object, not a DataFrame, we can use the usual indexing notation to refer to columns:>>> grouped = df.groupby(["city", "value"]) >>> grouped["value"].max() city London 6 Mexico 9 Mumbai 12 Tokyo 6 Name: value, dtype: int64 >>> grouped["value"].sum() city London 13 Mexico 20 Mumbai 29 Tokyo 16 Name: value, dtype: int64
- We see that, according to our data set, Mumbai seems to be a sunny city. An alternative – and more verbose – way to achieve the above would be:
>>> df['value'].groupby(df['city']).sum() city London 13 Mexico 20 Mumbai 29 Tokyo 16 Name: value, dtype: int64
Machine learning is a subfield of artificial intelligence that explores how machines can learn from data to analyze structures, help with decisions, and make predictions. In 1959, Arthur Samuel defined machine learning as the, "Field of study that gives computers the ability to learn without being explicitly programmed."
Each sample might consist of single or multiple values. In the context of machine learning, the properties of data are called features.
Machine learning can be arranged by the nature of the input data:
In supervised learning, the input data (typically denoted with x) is associated with a target label (y), whereas in unsupervised learning, we only have unlabeled input data.
Supervised learning can be further broken down into the following problems:
Classification problems have a fixed set of target labels, classes, or categories, while regression problems have one or more continuous output variables. Classifying e-mail messages as spam or not spam is a classification task with two target labels. Predicting house prices—given the data about houses, such as size, age, and nitric oxides concentration—is a regression task, since the price is continuous.
The scikit-learn library is organized into submodules. Each submodule contains algorithms and helper methods for a certain class of machine learning models and approaches.
Here is a sample of those submodules, including some example models:
Submodule |
Description |
Example models |
---|---|---|
cluster |
This is the unsupervised clustering |
KMeans and Ward |
decomposition |
This is the dimensionality reduction |
PCA and NMF |
ensemble |
This involves ensemble-based methods |
AdaBoostClassifier, AdaBoostRegressor, RandomForestClassifier, RandomForestRegressor |
lda |
This stands for latent discriminant analysis |
LDA |
linear_model |
This is the generalized linear model |
LinearRegression, LogisticRegression, Lasso and Perceptron |
mixture |
This is the mixture model |
GMM and VBGMM |
naive_bayes |
This involves supervised learning based on Bayes' theorem |
BaseNB and BernoulliNB, GaussianNB |
neighbors |
These are k-nearest neighbors |
KNeighborsClassifier, KNeighborsRegressor, LSHForest |
neural_network |
This involves models based on neural networks |
BernoulliRBM |
tree |
decision trees |
DecisionTreeClassifier, DecisionTreeRegressor |
While these approaches are diverse, a scikit-learn library abstracts away a lot of differences by exposing a regular interface to most of these algorithms. All of the example algorithms listed in the table implement a fit
method, and most of them implement predict as well. These methods represent two phases in machine learning. First, the model is trained on the existing data with the fit
method. Once trained, it is possible to use the model to predict the class or value of unseen data with predict. We will see both the methods at work in the next sections.
In contrast to the heterogeneous domains and applications of machine learning, the data representation in scikit-learn is less diverse, and the basic format that many algorithms expect is straightforward—a matrix of samples and features.
There is something like Hello World
in the world of machine learning datasets as well; for example, the Iris dataset whose origins date back to 1936. With the standard installation of scikit-learn, you already have access to a couple of datasets, including Iris that consists of 150 samples, each consisting of four measurements taken from three different Iris flower species:
The dataset is packaged as a bunch, which is only a thin wrapper around a dictionary:
Under the data
key, we can find the matrix of samples and features, and can confirm its shape:
The target names are encoded. We can look up the corresponding names in the target_names
attribute:
This is the basic anatomy of many datasets, such as example data, target values, and target names.
What are the features of a single entry in this dataset?:
The four features are the measurements taken of real flowers: their sepal length and width, and petal length and width. Three different species have been examined: the Iris-Setosa, Iris-Versicolour, and Iris-Virginica.
Machine learning tries to answer the following question: can we predict the species of the flower, given only the measurements of its sepal and petal length?
In the next section, we will see how to answer this question with scikit-learn.
If you work with your own datasets, you will have to bring them in a shape that scikit-learn expects, which can be a task of its own. Tools such as Pandas make this task much easier, and Pandas DataFrames can be exported to numpy.ndarray
easily with the as_matrix()
method on DataFrame.
In this section, we will show short examples for both classification and regression.
Classification problems are pervasive: document categorization, fraud detection, market segmentation in business intelligence, and protein function prediction in bioinformatics.
We see that the petal length (the third feature) exhibits the biggest variance, which could indicate the importance of this feature during classification. It is also insightful to plot the data points in two dimensions, using one feature for each axis. Also, indeed, our previous observation reinforced that the petal length might be a good indicator to tell apart the various species. The Iris setosa also seems to be more easily separable than the other two species:
From the visualizations, we get an intuition of the solution to our problem. We will use a supervised method called a Support Vector Machine (SVM) to learn about a classifier for the Iris data. The API separates models and data, therefore, the first step is to instantiate the model. In this case, we pass an optional keyword parameter to be able to query the model for probabilities later:
The next step is to fit the model according to our training data:
With this one line, we have trained our first machine learning model on a dataset. This model can now be used to predict the species of unknown data. If given some measurement that we have never seen before, we can use the predict method on the model:
In fact, the classifier is relatively sure about this label, which we can inquire into by using the predict_proba
method on the classifier:
Our example consisted of four features, but many problems deal with higher-dimensional datasets and many algorithms work fine on these datasets as well.
We first create a sample dataset as follows:
>>> from sklearn.linear_model import LinearRegression >>> clf = LinearRegression() >>> clf.fit(X, y)
We can plot the prediction over our data as well:
The output of the plot is as follows:
The above graph is a simple example with artificial data, but linear regression has a wide range of applications. If given the characteristics of real estate objects, we can learn to predict prices. If given the features of the galaxies, such as size, color, or brightness, it is possible to predict their distance. If given the data about household income and education level of parents, we can say something about the grades of their children.
A lot of existing data is not labeled. It is still possible to learn from data without labels with unsupervised models. A typical task during exploratory data analysis is to find related items or clusters. We can imagine the Iris dataset, but without the labels:
While the task seems much harder without labels, one group of measurements (in the lower-left) seems to stand apart. The goal of clustering algorithms is to identify these groups.
For example, we instantiate the KMeans
model with n_clusters
equal to 3
:
We can already compare the result of these algorithms with our known target labels:
We quickly relabel
the result to simplify the prediction error calculation:
As another example for an unsupervised algorithm, we will take a look at Principal Component Analysis (PCA). The PCA aims to find the directions of the maximum variance in high-dimensional data. One goal is to reduce the number of dimensions by projecting a higher-dimensional space onto a lower-dimensional subspace while keeping most of the information.
The problem appears in various fields. You have collected many samples and each sample consists of hundreds or thousands of features. Not all the properties of the phenomenon at hand will be equally important. In our Iris dataset, we saw that the petal length alone seemed to be a good discriminator of the various species. PCA aims to find principal components that explain most of the variation in the data. If we sort our components accordingly (technically, we sort the eigenvectors of the covariance matrix by eigenvalue), we can keep the ones that explain most of the data and ignore the remaining ones, thereby reducing the dimensionality of the data.
The process is similar to the ones we implemented so far. First, we instantiate our model; this time, the PCA from the decomposition submodule. We also import a standardization method, called StandardScaler
, that will remove the mean from our data and scale to the unit variance. This step is a common requirement for many machine learning algorithms:
Dimensionality reduction is just one way to deal with high-dimensional datasets, which are sometimes effected by the so called curse of dimensionality.
We have already seen that the machine learning process consists of the following steps:
- Model selection: We first select a suitable model for our data. Do we have labels? How many samples are available? Is the data separable? How many dimensions do we have? As this step is nontrivial, the choice will depend on the actual problem. As of Fall 2015, the scikit-learn documentation contains a much appreciated flowchart called choosing the right estimator. It is short, but very informative and worth taking a closer look at.
- Training: We have to bring the model and data together, and this usually happens in the fit methods of the models in scikit-learn.
- Application: Once we have trained our model, we are able to make predictions about the unseen data.
Whether or not a model generalizes well can also be tested. However, it is important that the training and the test input are separate. The situation where a model performs well on a training input but fails on an unseen test input is called overfitting, and this is not uncommon.
Cross-validation (CV) is a technique that does not need a validation set, but still counteracts overfitting. The dataset is split into k
parts (called folds). For each fold, the model is trained on k-1
folds and tested on the remaining folds. The accuracy is taken as the average over the folds.
We will show a five-fold cross-validation on the Iris dataset, using SVC again:
There are various strategies implemented by different classes to split the dataset for cross-validation: KFold
, StratifiedKFold
, LeaveOneOut
, LeavePOut
, LeaveOneLabelOut
, LeavePLableOut
, ShuffleSplit
, StratifiedShuffleSplit
, and PredefinedSplit
.
The most interesting applications will be found in your own field. However, if you would like to get some inspiration, we recommend that you look at the www.kaggle.com website that runs predictive modeling and analytics competitions, which are both fun and insightful.
Practice exercises
Are the following problems supervised or unsupervised? Regression or classification problems?:
- Recognizing coins inside a vending machine
- Recognizing handwritten digits
- If given a number of facts about people and economy, we want to estimate consumer spending
- If given the data about geography, politics, and historical events, we want to predict when and where a human right violation will eventually take place
- If given the sounds of whales and their species, we want to label yet unlabeled whale sound recordings
Look up one of the first machine learning models and algorithms: the perceptron. Try the perceptron on the Iris dataset and estimate the accuracy of the model. How does the perceptron compare to the SVC from this chapter?