Welcome! Let's get started. Python has become one of the de facto standard language and platform for data analysis and data science. The mind map that you will see shortly depicts some of the numerous libraries available in the Python ecosystem that are used by data analysts and data scientists. NumPy, SciPy, Pandas, and Matplotlib libraries lay the foundation of Python data analysis and are now part of SciPy Stack 1.0 (http://www.scipy.org/stackspec.html). We will learn how to install SciPy Stack 1.0 and Jupyter Notebook, and write some simple data analysis code as a warm-up exercise.
The following are the libraries available in the Python ecosystem that are used by data analysts and data scientists:
NumPy: This is a general-purpose library that provides numerical arrays, and functions to manipulate the arrays efficiently.
SciPy: This is a scientific computing library that provides science and engineering related functions. SciPy supplements and slightly overlaps NumPy. NumPy and SciPy historically shared their code base but were later separated.
Pandas: This is a data-manipulation library that provides data structures and operations for manipulating tables and time series data.
Matplotlib: This is a 2D plotting library that provides support for producing plots, graphs, and figures. Matplotlib is used by SciPy and supports NumPy.
IPython: This provides a powerful interactive shell for Python, kernel for Jupyter, and support for interactive data visualization. We will cover the IPython shell later in this chapter.
Jupyter Notebook: This provides a web-based interactive shell for creating and sharing documents with live code and visualizations. Jupyter Notebook supports multiple versions of Python through the kernel provided by IPython. We will cover the Jupyter Notebook later in this chapter.
Installation instructions for the other required software will be given throughout the book at the appropriate time. At the end of this chapter, you will find pointers on how to find additional information online if you get stuck or are uncertain about the best way of solving problems:
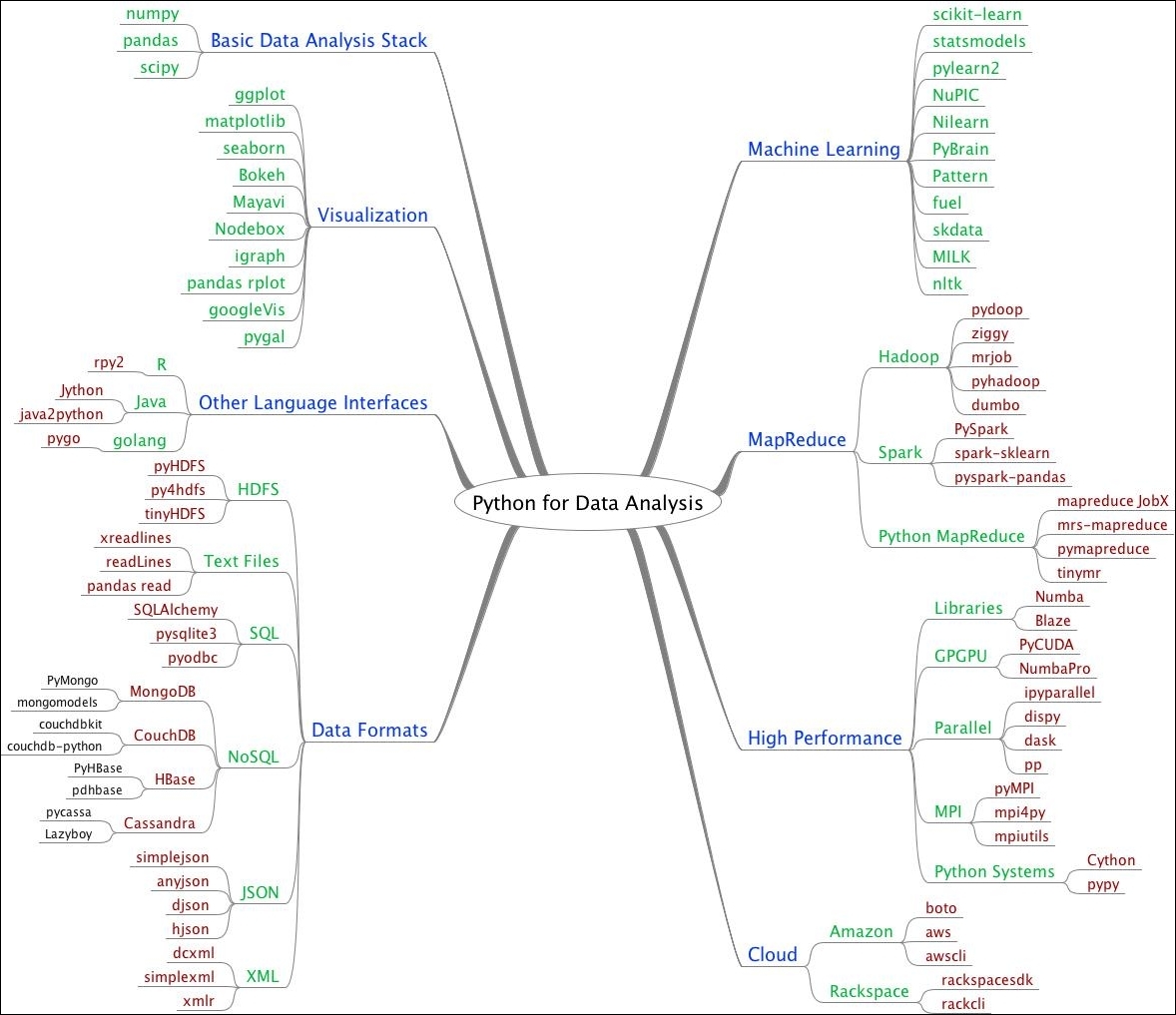
In this chapter, we will cover the following topics:
Installing Python 3
Using IPython as a shell
Reading manual pages
Jupyter Notebook
NumPy arrays
A simple application
Where to find help and references
Listing modules inside the Python libraries
Visualizing data using matplotlib
The software used in this book is based on Python 3, so you need to have Python 3 installed. On some operating systems, Python 3 is already installed. There are many implementations of Python, including commercial implementations and distributions. In this book, we will focus on the standard Python implementation, which is guaranteed to be compatible with NumPy.
Note
You can download Python 3.5.x from https://www.python.org/downloads/. On this web page, you can find installers for Windows and Mac OS X, as well as source archives for Linux, Unix, and Mac OS X. You can find instructions for installing and using Python for various operating systems at https://docs.python.org/3/using/index.html.
The software we will install in this chapter has binary installers for Windows, various Linux distributions, and Mac OS X. There are also source distributions, if you prefer. You need to have Python 3.5.x or above installed on your system. The sunset date for Python 2.7 was moved from 2015 to 2020, thus Python 2.7 will be supported and maintained until 2020. For these reasons, we have updated this book for Python 3.
We will learn how to install and set up NumPy, SciPy, Pandas, Matplotlib, IPython, and Jupyter Notebook on Windows, Linux, and Mac OS X. Let's look at the process in detail. We shall use pip3
to install the libraries. From version 3.4 onwards, pip3
has been included by default with the Python installation.
To install the foundational libraries, run the following command line instruction:
$ pip3 install numpy scipy pandas matplotlib jupyter notebook
It may be necessary to prepend sudo
to this command if your current user doesn't have sufficient rights on your system.
At the time of writing this book, we had the following software installed as a prerequisite on our Windows 10 virtual machine:
Python 3.6 from https://www.python.org/ftp/python/3.6.0/python-3.6.0-amd64.exe
Microsoft Visual C++ Build Tools 2015 from http://landinghub.visualstudio.com/visual-cpp-build-tools
Download and install the appropriate prebuilt NumPy and Scipy binaries for your Windows platform from http://www.lfd.uci.edu/~gohlke/pythonlibs/:
We downloaded numpy-1.12.0+mkl-cp36-cp36m-win_amd64.whl and scipy-0.18.1-cp36-cp36m-win_amd64.whl
After downloading, we executed the
pip3 install Downloads\numpy-1.12.0+mkl-cp36-cp36m-win_amd64.whl
andpip3 install Downloads\scipy-0.18.1-cp36-cp36m-win_amd64.whl
commands
After these prerequisites are installed, to install the rest of the foundational libraries, run the following command line instruction:
$ pip3 install pandas matplotlib jupyter
Data analysts, data scientists, and engineers are used to experimenting. IPython was created by scientists with experimentation in mind. The interactive environment that IPython provides is comparable to an interactive computing environment provided by Matlab, Mathematica, and Maple.
The following is a list of features of the IPython shell:
Tab completion, which helps you find a command
History mechanism
Inline editing
Ability to call external Python scripts with
%run
Access to system commands
Access to the Python debugger and profiler
The following list describes how to use the IPython shell:
Starting a session: To start a session with IPython,enter the following instruction on the command line:
$ ipython3 Python 3.5.2 (default, Sep 28 2016, 18:08:09) Type "copyright", "credits" or "license" for more information. IPython 5.1.0 -- An enhanced Interactive Python. ? -> Introduction and overview of IPython's features. %quickref -> Quick reference. help -> Python's own help system. object? -> Details about 'object', use 'object??' for extra details. In [1]: quit()
Saving a session: We might want to be able to go back to our experiments. In IPython, it is easy to save a session for later use with the following command:
In [1]: %logstart Activating auto-logging. Current session state plus future input saved: Filename : ipython_log.py Mode : rotate Output logging : False Raw input log : False Timestamping : False State : active
Logging can be switched off as follows:
In [9]: %logoff Switching logging OFF
Executing a system shell command: Execute a system shell command in the default IPython profile by prefixing the command with the
!
symbol. For instance, the following input will get the current date:In [1]: !date
In fact, any line prefixed with
!
is sent to the system shell. We can also store the command output, as shown here:In [2]: thedate = !date In [3]: thedate
Displaying history: We can show the history of our commands with the
%hist
command. For example:In [1]: a = 2 + 2 In [2]: a Out[2]: 4 In [3]: %hist a = 2 + 2 a %hist
This is a common feature in command line interface (CLI) environments. We can also search through the history with the
-g
switch as follows:In [5]: %hist -g a = 2 1: a = 2 + 2
We saw a number of so-called magic functions in action. These functions start with the %
character. If the magic function is used on a line by itself, the %
prefix is optional.
When the libraries are imported in IPython, we can open manual pages for library functions with the help
command. It is not necessary to know the name of a function. We can type a few characters and then let the tab completion do its work. Let's, for instance, browse the available information for the arange()
function.
We can browse the available information in either of the following two ways:
Calling the help function: Type in
help(
followed by a few characters of the function and press the Tab key. A list of functions will appear. Select the function from the list using the arrow keys and press the Enter key. Close the help function call with)
and press the Enter key.Querying with a question mark: Another option is to append a question mark to the function name. You will then, of course, need to know the function name, but you don't have to type
help
, for example:In [3]: numpy.arange?
Tab completion is dependent on
readline
, so you need to make sure that it is installed. It can be installed withpip
by typing the following command:$ pip3 install readline
The question mark gives you information from docstrings.
Jupyter Notebook, previously known as IPython Notebooks, provides a tool to create and share web pages with text, charts, and Python code in a special format. Have a look at these notebook collections at the following links:
Often, the notebooks are used as an educational tool, or to demonstrate Python software. We can import or export notebooks either from plain Python code or from the special notebook format. The notebooks can be run locally, or we can make them available online by running a dedicated notebook server. Certain cloud computing solutions, such as Wakari and PiCloud, allow you to run notebooks in the cloud. Cloud computing is one of the topics of Chapter 11, Environments Outside the Python Ecosystem and Cloud Computing.
To start a session with Jupyter Notebook,enter the following instruction on the command line:
$ jupyter-notebook
This will start the notebook server and open a web page showing the contents of the folder from which the command will execute. You can then select New | Python 3 to start a new notebook in Python 3.
You can also open ch-01.ipynb
, provided in the code package for this book. The ch-01
notebook file has the code for the simple applications that we will describe shortly.
After going through the installation of NumPy, it's time to have a look at NumPy arrays. NumPy arrays are more efficient than Python lists when it comes to numerical operations. NumPy arrays are, in fact, specialized objects with extensive optimizations. NumPy code requires less explicit loops than equivalent Python code. This is based on vectorization.
If we go back to high school mathematics, then we should remember the concepts of scalars and vectors. The number 2, for instance, is a scalar. When we add 2 to 2, we are performing scalar addition. We can form a vector out of a group of scalars. In Python programming terms, we will then have a one-dimensional array. This concept can, of course, be extended to higher dimensions. Performing an operation on two arrays, such as addition, can be reduced to a group of scalar operations. In straight Python, we will do that with loops going through each element in the first array and adding it to the corresponding element in the second array. However, this is more verbose than the way it is done in mathematics. In mathematics, we treat the addition of two vectors as a single operation. That's the way NumPy arrays do it too, and there are certain optimizations using low-level C routines that make these basic operations more efficient. We will cover NumPy arrays in more detail in the Chapter 2, NumPy Arrays.
Imagine that we want to add two vectors called a
and b
. The word vector is used here in the mathematical sense, which means a one-dimensional array. We will learn about specialized NumPy arrays that represent matrices in Chapter 4, Statistics and Linear Algebra. The vector a
holds the squares of integers 0
to n; for instance, if n
is equal to 3
, a
contains 0
, 1
, or 4
. The vector b
holds the cubes of integers 0
to n, so if n
is equal to 3
, then the vector b
is equal to 0
, 1
, or 8
. How would you do that using plain Python? After we come up with a solution, we will compare it to the NumPy equivalent.
The following function solves the vector addition problem using pure Python without NumPy:
def pythonsum(n): a = list(range(n)) b = list(range(n)) c = [] for i in range(len(a)): a[i] = i ** 2 b[i] = i ** 3 c.append(a[i] + b[i]) return c
The following is a function that solves the vector addition problem with NumPy:
def numpysum(n): a = numpy.arange(n) ** 2 b = numpy.arange(n) ** 3 c = a + b return c
Note that numpysum()
does not need a for
loop. We also used the arange()
function from NumPy, which creates a NumPy array for us with integers from 0
to n. The arange()
function was imported; that is why it is prefixed with numpy
.
Now comes the fun part. We mentioned earlier that NumPy is faster when it comes to array operations. How much faster is Numpy, though? The following program will show us by measuring the elapsed time in microseconds for the numpysum()
and pythonsum()
functions. It also prints the last two elements of the vector sum. Let's check that we get the same answers using Python and NumPy:
#!/usr/bin/env/python import sys from datetime import datetime import numpy as np """ This program demonstrates vector addition the Python way. Run the following from the command line: python vectorsum.py n Here, n is an integer that specifies the size of the vectors. The first vector to be added contains the squares of 0 up to n. The second vector contains the cubes of 0 up to n. The program prints the last 2 elements of the sum and the elapsed time: """ def numpysum(n): a = np.arange(n) ** 2 b = np.arange(n) ** 3 c = a + b return c def pythonsum(n): a = list(range(n)) b = list(range(n)) c = [] for i in range(len(a)): a[i] = i ** 2 b[i] = i ** 3 c.append(a[i] + b[i]) return c size = int(sys.argv[1]) start = datetime.now() c = pythonsum(size) delta = datetime.now() - start print("The last 2 elements of the sum", c[-2:]) print("PythonSum elapsed time in microseconds", delta.microseconds) start = datetime.now() c = numpysum(size) delta = datetime.now() - start print("The last 2 elements of the sum", c[-2:]) print("NumPySum elapsed time in microseconds", delta.microseconds)
The output of the program for 1000
, 2000
, and 3000
vector elements is as follows:
$ python3 vectorsum.py 1000 The last 2 elements of the sum [995007996, 998001000] PythonSum elapsed time in microseconds 976 The last 2 elements of the sum [995007996 998001000] NumPySum elapsed time in microseconds 87 $ python3 vectorsum.py 2000 The last 2 elements of the sum [7980015996, 7992002000] PythonSum elapsed time in microseconds 1623 The last 2 elements of the sum [7980015996 7992002000] NumPySum elapsed time in microseconds 143 $ python3 vectorsum.py 4000 The last 2 elements of the sum [63920031996, 63968004000] PythonSum elapsed time in microseconds 3417 The last 2 elements of the sum [63920031996 63968004000] NumPySum elapsed time in microseconds 237
Clearly, NumPy is much faster than the equivalent normal Python code. One thing is certain; we get the same results whether we are using NumPy or not. However, the result that is printed differs in representation. Note that the result from the numpysum()
function does not have any commas. How come? Obviously, we are not dealing with a Python list, but with a NumPy array. We will learn more about NumPy arrays in the Chapter 2, NumPy Arrays.
The following table lists documentation websites for the Python data analysis libraries we discussed in this chapter.
Packages |
Description |
NumPy and SciPy |
The main documentation website for NumPy and SciPy is at http://docs.scipy.org/doc/. Through this web page, you can browse NumPy and SciPy user guides and reference guides, as well as several tutorials. |
Pandas | |
Matplotlib | |
IPython | |
Jupyter Notebook |
The popular Stack Overflow software development forum has hundreds of questions tagged NumPy, SciPy, Pandas, Matplotlib, IPython, and Jupyter Notebook. To view them, go to http://stackoverflow.com/questions/tagged/<your-tag-word-here>
.
If you are really stuck with a problem, or you want to be kept informed of the development of these libraries, you can subscribe to their respective discussion mailing list(s). The number of e-mails per day varies from list to list. Developers actively involved with the development of these libraries answer some of the questions asked on the mailing lists.
For IRC users, there is an IRC channel on irc://irc.freenode.net
. The channel is called #scipy
, but you can also ask NumPy questions since SciPy users also have knowledge of NumPy, as SciPy is based on NumPy. There are at least 50 members on the SciPy channel at all times.
The ch-01.ipynb
file contains the code for looking at the modules inside the NumPy, SciPy, Pandas, and Matplotlib libraries. Don't worry about understanding the code just trying to run it for now. You can modify this code to look at the modules inside other libraries as well.
We shall learn about visualizing the data in a later chapter. For now, let's try loading two sample datasets and building a basic plot. First, install the sklearn library from which we shall load the data using the following command:
$ pip3 install scikit-learn
Import the datasets using the following command:
from sklearn.datasets import load_iris
from sklearn.datasets import load_boston
Import the Matplotlib plotting module:
from matplotlib import pyplot as plt
%matplotlib inline
Load the iris
dataset, print the description of the dataset, and plot column 1 (sepal length) as x
and column 2 (sepal width) as y
:
iris = load_iris() print(iris.DESCR) data=iris.data plt.plot(data[:,0],data[:,1],".")
The resulting plot will look like the following image:
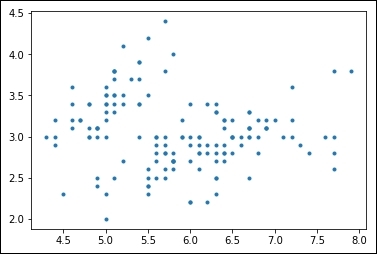
Load the boston dataset, print the description of the dataset and plot column 3 (proportion of non-retail business) as x
and column 5 (nitric oxide concentration) as y
, each point on the plot marked with a + sign:
boston = load_boston() print(boston.DESCR) data=boston.data plt.plot(data[:,2],data[:,4],"+")
The resulting plot will look like the following image:
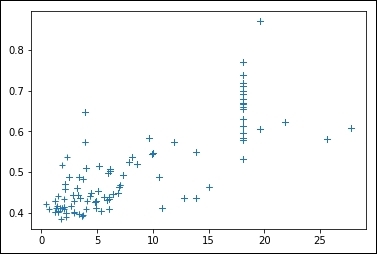
In this chapter, we installed NumPy, SciPy, Pandas, Matplotlib, IPython, and Jupyter Notebook, all of which we will be using in this book. We got a vector addition program working, and learned how NumPy offers superior performance. In addition, we explored the available documentation and online resources. We executed code to find the modules inside the libraries and loaded some sample datasets to draw some basic plots using Matplotlib.
In the next chapter, Chapter 2, NumPy Arrays, we will take a look under the hood of NumPy and explore some fundamental concepts, including arrays and data types.