Chapter 1: Deep Learning Life Cycle and MLOps Challenges
The past few years have seen great success in Deep Learning (DL) for solving practical business, industrial, and scientific problems, particularly for tasks such as Natural Language Processing (NLP), image, video, speech recognition, and conversational understanding. While research in these areas has made giant leaps, bringing these DL models from offline experimentation to production and continuously improving the models to deliver sustainable values is still a challenge. For example, a recent article by VentureBeat (https://venturebeat.com/2019/07/19/why-do-87-of-data-science-projects-never-make-it-into-production/) found that 87% of data science projects never make it to production. While there might be business reasons for such a low production rate, a major contributing factor is the difficulty caused by the lack of experiment management and a mature model production and feedback platform.
This chapter will help us to understand the challenges and bridge these gaps by learning the concepts, steps, and components that are commonly used in the full life cycle of DL model development. Additionally, we will learn about the challenges of an emerging field known as Machine Learning Operations (MLOps), which aims to standardize and automate ML life cycle development, deployment, and operation. Having a solid understanding of these challenges will motivate us to learn the skills presented in the rest of this book using MLflow, an open source, ML full life cycle platform. The business values of adopting MLOps' best practices are numerous; they include faster time-to-market of model-derived product features, lower operating costs, agile A/B testing, and strategic decision making to ultimately improve customer experience. By the end of this chapter, we will have learned about the critical role that MLflow plays in the four pillars of MLOps (that is, data, model, code, and explainability), implemented our first working DL model, and grasped a clear picture of the challenges with data, models, code, and explainability in DL.
In this chapter, we're going to cover the following main topics:
- Understanding the DL life cycle and MLOps challenges
- Understanding DL data challenges
- Understanding DL model challenges
- Understanding DL code challenges
- Understanding DL explainability challenges
Technical requirements
All of the code examples for this book can be found at the following GitHub URL: https://github.com/PacktPublishing/Practical-Deep-Learning-at-Scale-with-MLFlow.
You need to have Miniconda (https://docs.conda.io/en/latest/miniconda.html) installed on your development environment. In this chapter, we will walk through the process of installing the PyTorch lightning-flash
library (https://github.com/PyTorchLightning/lightning-flash), which can be used to build our first DL model in the Implementing a basic DL sentiment classifier section. Alternatively, you can sign up for a free Databricks Community Edition account at https://community.cloud.databricks.com/login.html and use a GPU cluster and a notebook to carry out the model development described in this book.
In addition to this, if you are a Microsoft Windows user, we recommend that you install WSL2 (https://www.windowscentral.com/how-install-wsl2-windows-10) so that you have a Linux environment to run the command lines that are present in this book.
Understanding the DL life cycle and MLOps challenges
Nowadays, the most successful DL models that are deployed in production primarily observe the following two steps:
- Self-supervised learning: This refers to the pretraining of a model in a data-rich domain that does not require labeled data. This step produces a pretrained model, which is also called a foundation model, for example, BERT, GPT-3 for NLP, and VGG-NETS for computer vision.
- Transfer learning: This refers to the fine-tuning of the pretrained model in a specific prediction task such as text sentiment classification, which requires labeled training data.
One ground-breaking and successful example of a DL model in production is the Buyer Sentiment Analysis model, which is built on top of BERT for classifying sales engagement email messages, providing critical fine-grained insights into buyer emotions and signals beyond simple activity metrics such as reply, click, and open rates (https://www.prnewswire.com/news-releases/outreach-unveils-groundbreaking-ai-powered-buyer-sentiment-analysis-transforming-sales-engagement-301188622.html). There are different variants regarding how this works, but in this book, we will primarily focus on the Transfer Learning paradigm of developing and deploying DL models, as it exemplifies a practical DL life cycle.
Let's walk through an example to understand a typical core DL development paradigm. For example, the popular BERT model released in late 2018 (a basic version of the BERT model can be found at https://huggingface.co/bert-base-uncased) was initially pretrained on raw texts (without human labeling) from over 11,000 books from BookCorpus and the entire English Wikipedia. This pretrained language model was then fine-tuned to many downstream NLP tasks, such as text classification and sentiment analysis, in different application domains such as movie review classifications by using labeled movie review data (https://huggingface.co/datasets/imdb). Note that sometimes, it might be necessary to further pretrain a foundation model (for example, BERT) within the application domain by using unlabeled data before fine-tuning to boost the final model performance in terms of accuracy. This core DL development paradigm is illustrated in Figure 1.1:
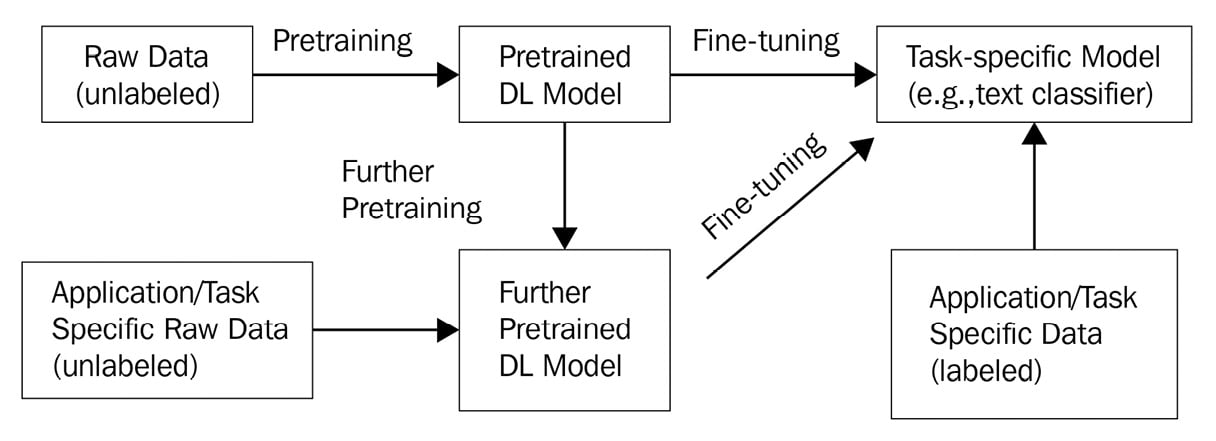
Figure 1.1 – A typical core DL development paradigm
Note that while Figure 1.1 represents a common development paradigm, not all of these steps are necessary for a specific application scenario. For example, you might only need to do fine-tuning using a publicly available pretrained DL model with your labeled application-specific data. Therefore, you don't need to do your own pretraining or carry out further pretraining using unlabeled data since other people or organizations have already done the pretraining step for you.
DL over Classical ML
Unlike classical ML model development, where, usually, a feature engineering step is required to extract and transform raw data into features to train an ML model such as decision tree or logistic regression, DL can learn the features automatically, which is especially attractive for modeling unstructured data such as texts, images, videos, audio, and speeches. DL is also called representational learning due to this characteristic. In addition to this, DL is usually data- and compute-intensive, requiring Graphics Process Units (GPUs), Tensor Process Units (TPU), or other types of computing hardware accelerators for at-scale training and inference. Explainability for DL models is also harder to implement, compared with traditional ML models, although recent progress has now made that possible.
Implementing a basic DL sentiment classifier
To set up the development of a basic DL sentiment classifier, you need to create a virtual environment in your local environment. Let's assume that you have miniconda installed. You can implement the following in your command-line prompt to create a new virtual environment called dl_model
and install the PyTorch lightning-flash
package so that the model can be built:
conda create -n dl_model python==3.8.10 conda activate dl_model pip install lightning-flash[all]==0.5.0 pip pytorch-lightning==1.4.4 datasets==1.9.0
Depending on your local machine's memory, the preceding commands might take about 10 minutes to finish. You can verify the success of your installation by running the following command:
conda list | grep lightning
If you see output similar to the following, your installation was successful:
lightning-bolts 0.3.4 pypi_0 pypi lightning-flash 0.5.0 pypi_0 pypi pytorch-lightning 1.4.4 pypi_0 pypi
Now you are ready to build your first DL model!
To begin building a DL model, complete the following steps:
- Import the necessary
torch
andflash
libraries, and importdownload_data
,TextClassificationData
, andTextClassifier
from theflash
subpackages:import torch import flash from flash.core.data.utils import download_data from flash.text import TextClassificationData, TextClassifier
- To get the dataset for fine-tuning, use
download_data
to download theimdb.zip
file, which is the public domain binary sentiment classification (positive/negative) dataset from Internet Movie Database (IMDb) to a local data folder. The IMDb ZIP file contains three CSV files:train.csv
valid.csv
test.csv
Each file contains two columns: review
and sentiment
. We then use TextClassificationData.from_csv
to declare a datamodule
variable that assigns the "review" to input_fields
, and the "sentiment" to target_fields
. Additionally, it assigns the train.csv
file to train_file
, the valid.csv
file to val_file
, and the test.csv
file to the test_file
properties of datamodule
, respectively:
download_data("https://pl-flash-data.s3.amazonaws.com/imdb.zip", "./data/") datamodule = TextClassificationData.from_csv( input_fields="review", target_fields="sentiment", train_file="data/imdb/train.csv", val_file="data/imdb/valid.csv", test_file="data/imdb/test.csv" )
- Once we have the data, we can now perform fine-tuning using a foundation model. First, we declare
classifier_model
by callingTextClassifier
with a backbone assigned toprajjwal1/bert-tiny
(which is a much smaller BERT-like pretrained model located in the Hugging Face model repository: https://huggingface.co/prajjwal1/bert-tiny). This means our model will be based on thebert-tiny
model. - The next step is to set up the trainer by defining how many epochs we want to run and how many GPUs we want to use to run them. Here,
torch.cuda.device_count()
will return either 0 (no GPU) or 1 to N, where N is the maximum number of GPUs you can have in your running environment. Now we are ready to calltrainer.finetune
to train a binary sentiment classifier for the IMDb dataset:classifier_model = TextClassifier(backbone="prajjwal1/bert-tiny", num_classes=datamodule.num_classes) trainer = flash.Trainer(max_epochs=3, gpus=torch.cuda.device_count()) trainer.finetune(classifier_model, datamodule=datamodule, strategy="freeze")
DL Fine-Tuning Time
Depending on your running environment, the fine-tuning step might take a couple of minutes on a GPU or around 10 minutes (if you're only using a CPU). You can reduce
max_epochs=1
if you simply want to get a basic version of the sentiment classifier quickly. - Once the fine-tuning step is complete, we will test the accuracy of the model by running
trainer.test()
:trainer.test()
The output of the test should look similar to the following screenshot, whichindicates that the final model accuracy is about 52%:
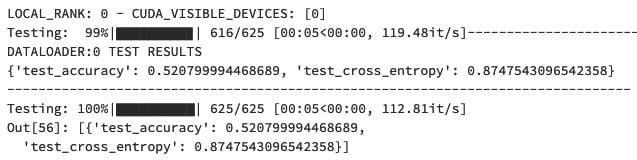
Figure 1.2 – The test results of our first DL model
The test result shown in the preceding diagram indicates that we have a basic version of the model, as we only fine-tuned the foundation model for three epochs and haven't used any advanced techniques such as hyperparameter tuning or better fine-tuning strategies. However, this is a great accomplishment since you now have a working knowledge of how the core DL model paradigm works! We will explore more advanced model training techniques in later chapters of this book.
Understanding DL's full life cycle development
By now, you should have your first DL model ready and should feel proud of it. Now, let's explore the full DL life cycle together to fully understand its concepts, components, and challenges.
You might have gathered that the core DL development paradigm revolves around three key artifacts: Data, Model, and Code. In addition to this, Explainability is another major artifact that is required in many mission-critical application scenarios such as medical diagnoses, the financial industry, and decision making for criminal justice. As DL is usually considered a black box, providing explainability for DL increasingly becomes a key requirement before and after shipping to production.
Note that Figure 1.1 is still considered offline experimentation if we are still trying to figure out which model works using a dataset in a lab-like environment. Even in such an offline experimentation environment, things will quickly become complicated. Additionally, we would like to know and track which experiments we have or have not performed so that we don't waste time repeating the same experiments, whatever parameters and datasets we have used, and whatever kind of metrics we have for a specific model. Once we have a model that's good enough for the use cases and customer scenarios, the complexity increases as we need a way to continuously deploy and update the model in production, monitor the model and data drift, and then retrain the model when necessary. This complexity further increases when at-scale training, deployment, monitoring, and explainability are needed.
Let's examine what a DL life cycle looks like (see Figure 1.3). There are five stages:
- Data collection, cleaning, and annotation/labeling.
- Model development (which is also known as offline experimentation). The core DL development paradigm in Figure 1.1 is considered part of the model development stage, which itself can be an iterative process.
- Model deployment and serving in production.
- Model validation and A/B testing (which is also known as online experimentation; this is usually in a production environment).
- Monitoring and feedback data collection during production.
Figure 1.3 provides a diagram to show that it is a continuous development cycle for a DL model:
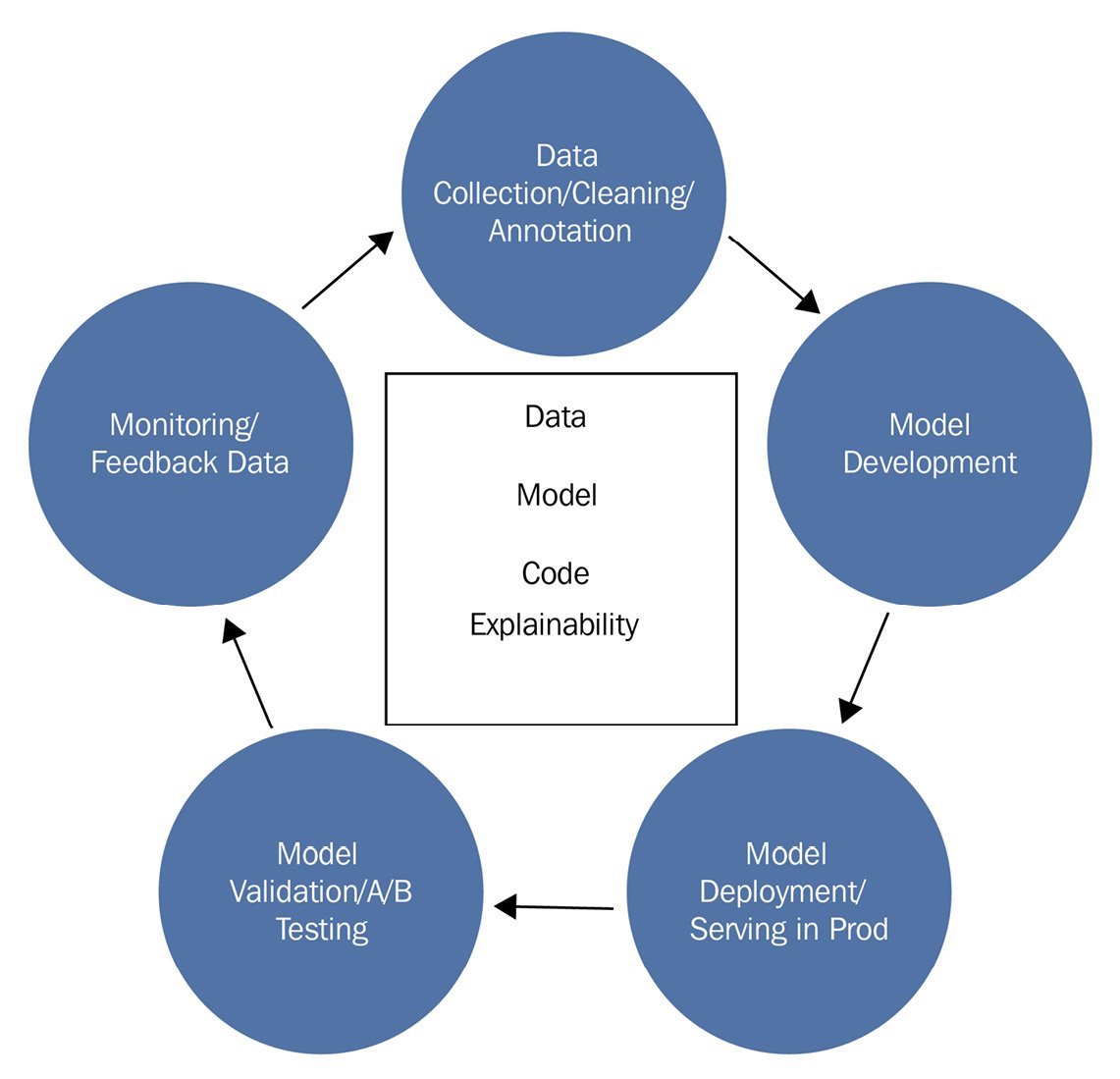
Figure 1.3 – The full DL development life cycle
In addition to this, we want to point out that the backbone of these five stages, as shown in Figure 1.3, essentially revolves around the four artifacts: data, model, code, and explainability. We will examine the challenges related to these four artifacts in the life cycle in the following sections. However, first, let's explore and understand MLOps, which is an evolving platform concept and framework that supports the full life cycle of ML. This will help us understand these challenges in a big-picture context.
Understanding MLOps challenges
MLOps has some connections to DevOps, where a set of technology stacks and standard operational procedures are used for software development and deployment combined with IT operations. Unlike traditional software development, ML and especially DL represent a new era of software development paradigms called Software 2.0 (https://karpathy.medium.com/software-2-0-a64152b37c35). The key differentiator of Software 2.0 is that the behavior of the software does not just depend on well-understood programming language code (which is the characteristic of Software 1.0) but depends on the learned weights in a neural network that's difficult to write as code. In other words, there exists an inseparable integration of the code, data, and model that must be managed together. Therefore, MLOps is being developed and is still evolving to accommodate this new Software 2.0 paradigm. In this book, MLOps is defined as an operational automation platform that consists of three foundation layers and four pillars. They are listed as follows:
- Here are the three foundation layers:
- Here are the four pillars:
- Data observability and management
- Model observability and life cycle management
- Explainability and Artificial Intelligence (AI) observability
- Code reproducibility and observability
Additionally, we will explain MLflow's roles in these MLOps layers and pillars so that we have a clear picture regarding what MLflow can do to build up the MLOps layers in their entirety:
- Infrastructure management and automation: This includes, but is not limited to, Kubernetes (also known as k8s) for automated container orchestration and Terraform (commonly used for managing hundreds of cloud services and access control). These tools are adapted to manage ML and DL applications that have deployed models as service endpoints. These infrastructure layers are not the focus of this book; instead, we will focus on how to deploy a trained DL model using MLflow's provided capabilities.
- Application life cycle management and CI/CD: This includes, but is not limited to, Docker containers for virtualization, container life cycle management tools such as Kubernetes, and CircleCI or Concourse for CI and CD. Usually, CI means that whenever there are code or model changes in a GitHub repository, a series of automatic tests will be triggered to make sure no breaking changes are introduced. Once these tests have been passed, new changes will be automatically released as part of a new package. This will then trigger a new deployment process (CD) to deploy the new package to the production environment (often, this will include human approval as a safety gate). Note that these tools are not unique to ML applications but have been adapted to ML and DL applications, especially when we require GPU and distributed clusters for the training and testing of DL models. In this book, we will not focus on these tools but will mention the integration points or examples when needed.
- Service system observability: This is mostly for monitoring the hardware/clusters/CPU/memory/storage, operating system, service availability, latency, and throughput. This includes tools such as Grafana, Datadog, and more. Again, these are not unique to ML and DL applications and are not the focus of this book.
- Data observability and management: This is traditionally under-represented in the DevOps world but becomes very important in MLOps as data is critical within the full life cycle of ML/DL models. This includes data quality monitoring, outlier detection, data drift and concept drift detection, bias detection, secured and compliant data sharing, data provenance tracking and versioning, and more. The tool stacks in this area that are suitable for ML and DL applications are still emerging. A few examples include DataFold (https://www.datafold.com/) and Databand (https://databand.ai/open-source/). A recent development in data management is a unified lakehouse architecture and implementation called Delta Lake (http://delta.io) that can be used for ML data management. MLflow has native integration points with Delta Lake, and we will cover that integration in this book.
- Model observability and life cycle management: This is unique to ML/DL models, and it only became widely available recently due to the rise of MLflow. This includes tools for model training, testing, versioning, registration, deployment, serialization, model drift monitoring, and more. We will learn about the exciting capabilities that MLflow provides in this area. Note that once we combine CI/CD tools with MLflow training/monitoring, user feedback loops, and human annotations, we can achieve Continuous Training, Continuous Testing, and Continuous Labeling. MLflow provides the foundational capabilities so that further automation in MLOps becomes possible, although such complete automation will not be the focus of this book. Interested readers can find relevant references at the end of this chapter to explore this area further.
- Explainability and AI observability: This is unique to ML/DL models and is especially important for DL models, as traditionally, DL models are treated as black boxes. Understanding why the model provides certain predictions is critical for societally important applications. For example, in medical, financial, juridical, and many human-in-the-loop decision support applications, such as civilian and military emergency response, the demand for explainability is increasingly higher. MLflow provides native integration with a popular explainability framework called SHAP, which we will cover in this book.
- Code reproducibility and observability: This is not entirely unique to ML/DL applications. However, DL models face some special challenges as the number of DL code frameworks are diverse and the need to reproduce a model is not entirely up to the code alone (we also need data and execution environments such as GPU clusters). In addition to this, notebooks are commonly used in model development and production. How to manage the notebooks along with the model run is important. Usually, GitHub is used to manage the code repository; however, we need to structure the ML project code in a way that's reproducible either locally (such as on a local laptop) or remotely (for example, in a Databricks' GPU cluster). MLflow provides this capability to allow DL projects that have been written once to run anywhere, whether this is in an offline experimentation environment or an online production environment. We will cover MLflow's MLproject capability in this book.
In summary, MLflow plays a critical and foundational role in MLOps. It fills in the gaps that DevOps traditionally does not cover and, thus, is the focus of this book. The following diagram (Figure 1.4) shows the central roles of MLflow in the still-evolving MLOps world:
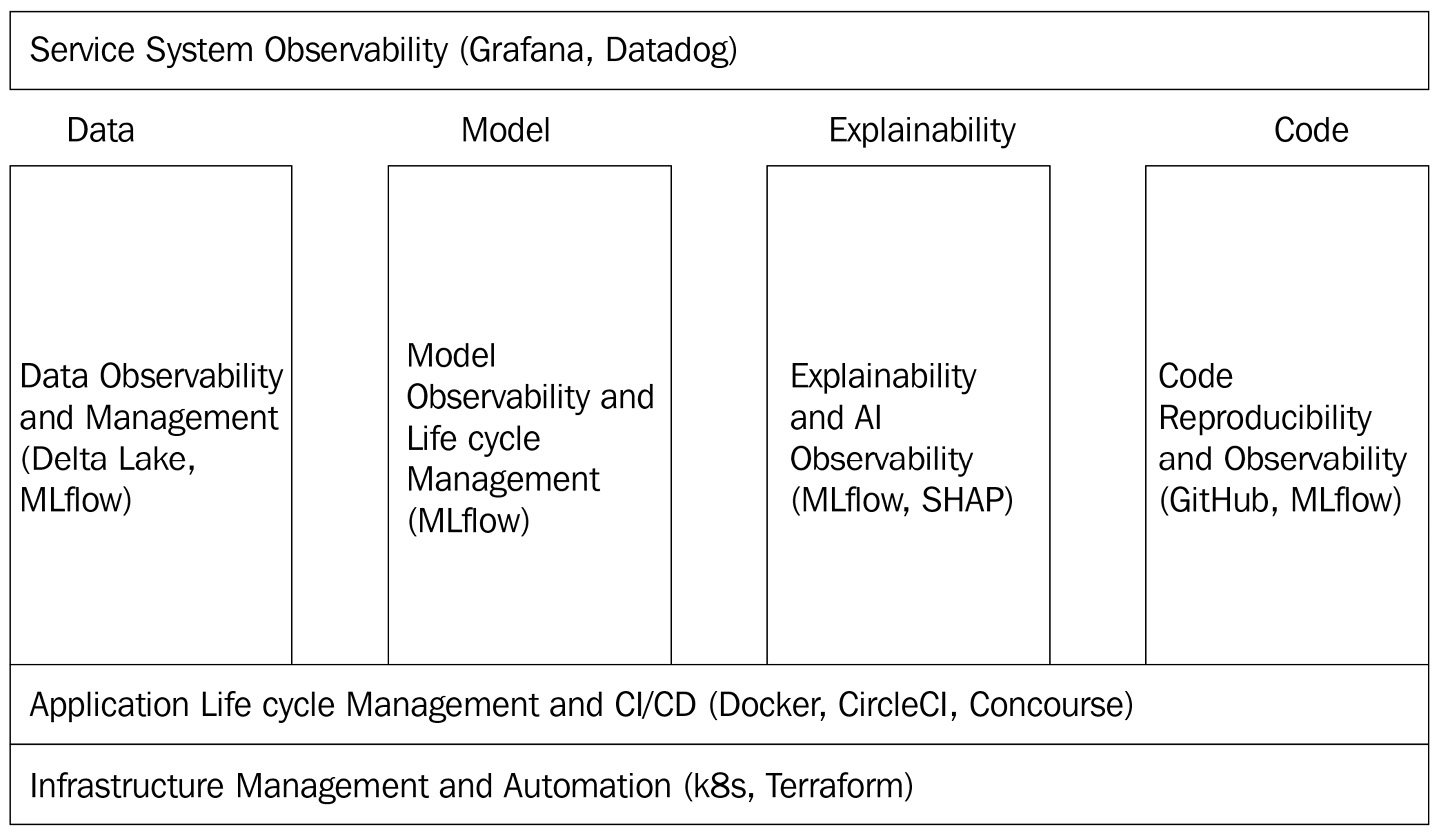
Figure 1.4 – The three layers and four pillars of MLOps and MLflow's roles
While the bottom two layers and the topmost layer are common within many software development and deployment processes, the middle four pillars are either entirely unique to ML/DL applications or partially unique to ML/DL applications. MLflow plays a critical role in all four of these pillars in MLOps. This book will help you to confidently apply MLflow to solve the issues of these four pillars while also equipping you to further integrate with other tools in the MLOps layers depicted in Figure 1.4 for full automation depending on your scenario requirements.
Understanding DL data challenges
In this section, we will discuss the data challenges at each stage of the DL life cycle, as illustrated in Figure 1.3. Essentially, DL is a data-centric AI, unlike symbolic AI where human knowledge can be used without lots of data. The challenges for data in DL are pervasive in all stages of the full life cycle:
- Data collection/cleaning/annotation: One of DL's first successes began with ImageNet (https://www.image-net.org/), where millions of images are collected and annotated according to the English nouns in the WordNet database (https://wordnet.princeton.edu/). This led to the successful development of pretrained DL models for computer vision such as VGG-NETS (https://pytorch.org/hub/pytorch_vision_vgg/), which can perform state-of-the-art image classification and is widely used for industrial and business applications. The main challenge of this kind of large-scale data collection and annotation is the unknown bias, which is hard to measure in this process (https://venturebeat.com/2020/11/03/researchers-show-that-computer-vision-algorithms-pretrained-on-imagenet-exhibit-multiple-distressing-biases/). Another example is the sales engagement platform Outreach (https://www.outreach.io/), where we can classify a potential buyer's sentiment. For instance, we might start by collecting email messages of 100 paid organizations to train a DL model. Following this, we would need to collect email messages from more organizations, either due to an accuracy requirement or expanded language coverage (such as from English only to other languages such as German and French). These many iterations of data collection and annotation will generate quite a lot of datasets. There is a tendency to just name the version of the dataset with hardcoded version numbers as part of a dataset filename such as the following:
MyCoolAnnotatedData-v1.0.csv MyCoolAnnotatedData-v2.0.csv MyCoolAnnotatedData-v3.0.csv MyCoolAnnotatedData-v4.0.csv
This seems to work until some changes are required in any one of the vX.0 datasets due to the need to correct annotation errors or remove email messages because of customer churn. Also, what happens if we need to combine several datasets together or perform some data cleaning and transformation to train a new DL model? What if we need to implement data augmentation to artificially generate some datasets? Evidently, simply changing the names of the files is neither scalable nor sustainable.
- Model development: We need to understand that the bias in the data we use to train/pretrain a DL model will reflect in the prediction when applying the model. While we do not focus on de-biasing data in this book, we must implement data versioning and data provenance as first-class artifacts when training and serving a DL model so that we can track all model experiments. When fine-tuning a pretrained model for our use cases, as we did earlier, we also need to track the versioning of the fine-tuning dataset we use. In our previous example, we use a variant of the BERT model to fine-tune the IMDb review data. While, in our first example, we did not care about the versioning or source of the data, this is important for a practical and real application. In summary, DL models need to link to a particular version of datasets using a scalable approach. We will provide solutions to this topic in this book.
- Model deployment and serving in production: This is for deploying into the production environment to serve online traffic. DL model serving latency is of particular importance and is interesting to collect at this stage. This might allow you to adjust the hardware environment used for inference.
- Model validation and A/B testing: The data we collect at this stage is mostly for user behavior metrics in the online experimentation environment (https://www.slideshare.net/pavel/ab-testing-ai-global-artificial-intelligence-conference-2019). Online data traffic also needs to be characterized in order to understand whether there is a statistical difference in the input to the model between offline experimentation and online experimentation. Only if we pass the A/B testing and validate that the model indeed works better than its previous version in terms of user behavior metrics do we roll out to production for all users.
- Monitoring and feedback loops: In this stage, note that the data will need to be continuously collected to detect data drift and concept drift. For example, in the buyer sentiment classification example discussed earlier, if buyers start to use terminology that is not encountered in the training data, the performance of the model could suffer.
In summary, data tracking and observability are major challenges in all stages of the DL life cycle.
Understanding DL model challenges
In this section, we will discuss DL model challenges. Let's look at the challenges at each stage of the DL life cycle, as depicted in Figure 1.3:
- Data collection/cleaning/annotation: While the data challenge has already been stated, the challenge of linking data to the model of interest still exists. MLflow has native integration with Delta Lake so that any trained model can be traced back to a particular version within Delta Lake.
- Model development: This is the time for trying lots of model frameworks, packages, and model selections. We need to track all the packages we use, along with the model parameters, hyperparameters, and model metrics in all experiments we run. Without a scalable and standardized way to track all experiments, this becomes a very tangled space. This not only causes trouble in terms of not knowing which experiments have been done so that we don't waste time doing them again, but it also creates problems when tracking which model is ready to be deployed or has already been deployed. Model serialization is another major challenge as different DL frameworks tend to use different ways to serialize the model. For example,
pickle
. (https://github.com/cloudpipe/cloudpickle) is usually used in serializing the model written in Python. However, TorchScript (https://pytorch.org/docs/stable/jit.html) is now highly performant for PyTorch models. In addition, Open Neural Network Exchange or ONNX (https://onnx.ai/) tries to provide more framework-agnostic DL serialization. Finally, we need to log the serialized model and register the model so that we can track model versioning. MLflow is one of the first open source tools to overcome these challenges. - Model deployment and serving in production: An easy-to-use model deployment tool that can tie into the model registry is a challenge. MLflow can be used to alleviate that, allowing you to load models for production deployment with full provenance tracking.
- Model validation and A/B testing: During online validation and experimentation, model performance needs to be validated and user behavior metrics need to be collected. This is so that we can easily roll back or redeploy a particular version of the models. A model registry is critical for at-scale online model production validation and experimentation.
- Monitoring and feedback loops: Model drifting and degradation over time is a real challenge. The visibility of model performance in production needs to be continuously monitored. Feedback data can be used to decide whether a model needs to be retrained.
In summary, DL model challenges in the full life cycle are unique. It is also worth pointing out a common framework that can assist the model development and online production back-and-forth is of great importance, as we don't want to use different tools just because the execution environment is different. MLflow provides this unified framework to bridge such gaps.
Understanding DL code challenges
In this section, we will discuss DL code challenges. Let's look at how these code challenges are manifested in each of the stages described in Figure 1.3. In this section, and within the context of DL development, code refers to the source code that's written in certain programming languages such as Python for data processing and implementation, while a model refers to the model logic and architecture in its serialized format (for example, pickle, TorchScript, or ONNX):
- Data collection/cleaning/annotation: While data is the central piece in this stage, the code that does the query, extraction/transformation/loading (ETL), and data cleaning and augmentation is of critical importance. We cannot decouple the development of the model from the data pipelines that provide the data feeds to the model. Therefore, data pipelines that implement ETL need to be treated as one of the integrated steps in both offline experimentation and online production. A common mistake is that we use different data ETL and cleaning pipelines in offline experimentation, and then implement different data ETL/cleaning pipelines in online production, which could cause different model behaviors. We need to version and serialize the data pipeline as part of the entire model pipeline. MLflow provides several ways to allow us to implement such multistep pipelines.
- Model development: During offline experiments, in addition to different versions of data pipeline code, we might also have different versions of notebooks or use different versions of DL library code. The usage of notebooks is particularly unique in ML/DL life cycles. Tracking which model results are produced by which notebook/model pipeline/data pipeline needs to be done for each run. MLflow does that with automatic code version tracking and dependencies. In addition, code reproducibility in different running environments is unique to DL models, as DL models usually require hardware accelerators such as GPUs or TPUs. The flexibility of running either locally, or remotely, on a CPU or GPU environment is of great importance. MLflow provides a lightweight approach in which to organize the ML projects so that code can be written once and run everywhere.
- Model deployment and serving in production: While the model is serving production traffic, any bugs will need to be traced back to both the model and code. Thus, tracking code provenance is critical. It is also critical to track all the dependency code library versions for a particular version of the model.
- Model validation and A/B testing: Online experiments could use multiple versions of models using different data feeds. Debugging any experimentation will require not only knowing which model is used but also which code is used to produce that model.
- Monitoring and feedback loops: This stage is similar to the previous stage in terms of code challenges, where we need to know whether model degradation is due to code bugs or model and data drifting. The monitoring pipeline needs to collect all the metrics for both data and model performance.
In summary, DL code challenges are especially unique because DL frameworks are still evolving (for example, TensorFlow, PyTorch, Keras, Hugging Face, and SparkNLP). MLflow provides a lightweight framework to overcome many common challenges and can interface with many DL frameworks seamlessly.
Understanding DL explainability challenges
In this section, we will discuss DL explainability challenges at each of the stages described in Figure 1.3. It is increasingly important to view explainability as an integral and necessary mechanism to define, test, debug, validate, and monitor models across the entire model life cycle. Embedding explainability early will make subsequent model validation and operations easier. Also, to maintain ongoing trust in ML/DL models, it is critical to be able to explain and debug ML/DL models after they go live in production:
- Data collection/cleaning/annotation: As we have gathered, explainability is critical for model prediction. The root cause of any model's trustworthiness or bias can be traced back to the data used to train the model. Explainability for the data is still an emerging area but is critical. So, what could go wrong and become a challenge during the data collection/cleaning/annotation stage? For example, let's suppose we have an ML/DL model, and its prediction outcome is about whether a loan applicant will pay back a loan or not. If the data collected has certain correlations between age and the loan payback outcome, this will cause the model to use age as a predictor. However, a loan decision based on a person's age is against the law and not allowed even if the model works well. So, during data collection, it could be that the sampling strategy is not sufficient to represent certain subpopulations such as different loan applicants in different age groups.
A subpopulation could have lots of missing fields and then be dropped during data cleaning. This could result in underrepresentation following the data cleaning process. Human annotations could favor the privileged group and other possible unconscious biases. A metric called Disparate Impact could reveal the hidden biases in the data, which compares the proportion of individuals that receive a positive outcome for two groups: an unprivileged group and a privileged group. If the unprivileged group (for example, persons with age > 60) receives a positive outcome (for example, loan approval) less than 80% of the proportion of the privileged group (persons with age < 60), this is a disparate impact violation based on the current common industry standard (a four-fifths rule). Tools such as Dataiku could help to automate the disparate impact and subpopulation analysis to find groups of people who may be treated unfairly or differently because of the data used for model training.
- Model development: Model explainability during offline experimentation is very important to not only help understand why a model behaves a certain way but also help with model selection to decide which model to use if we need to put it into production. Accuracy might not be the only criteria to select a winning model. There are a few DL explainability tools, such as SHAP (please refer to Figure 1.5). MLflow integration with SHAP provides a way to implement DL explainability:
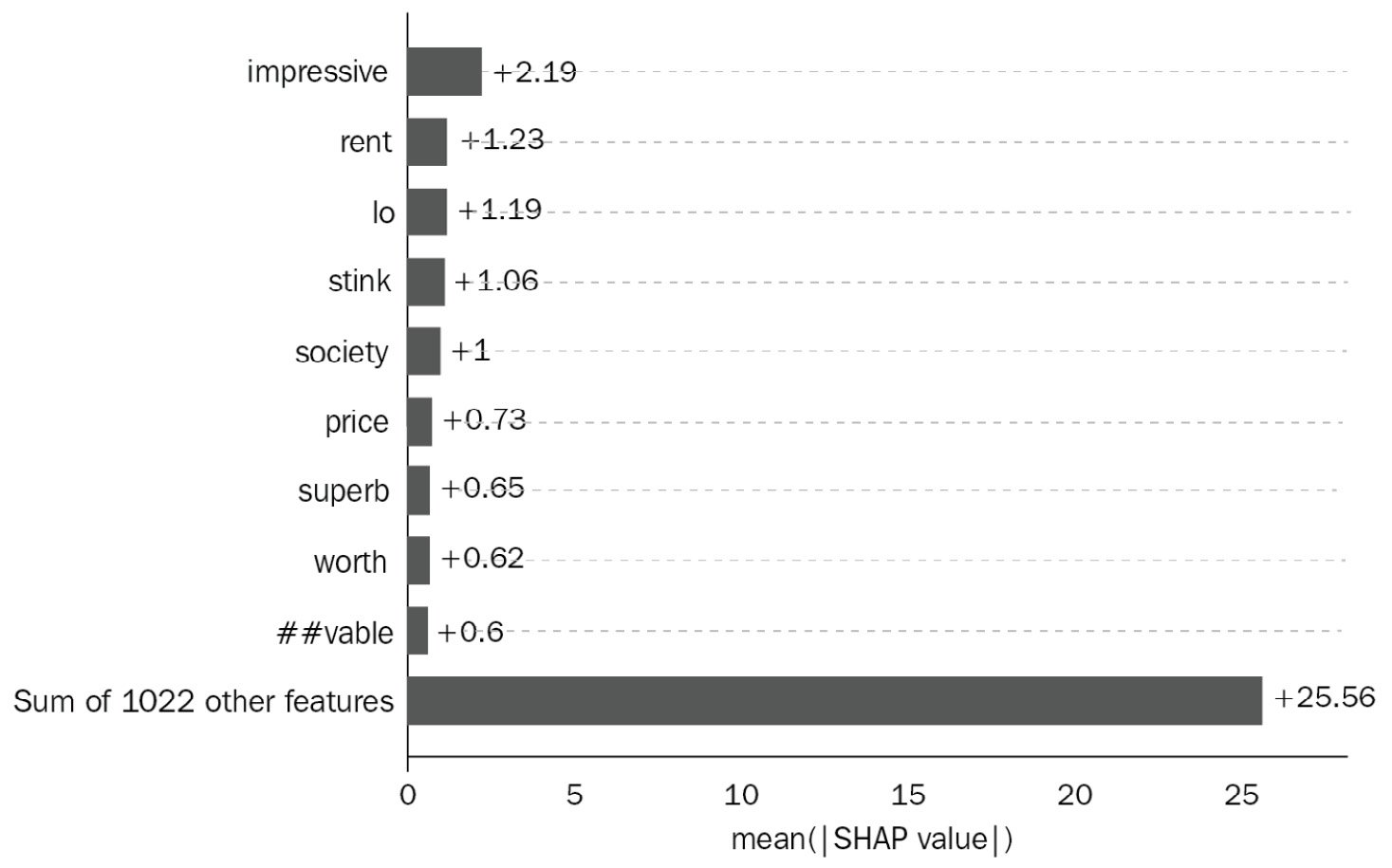
Figure 1.5 – NLP text SHAP Variable Importance Plot when using a DL model
Figure 1.5 shows that this NLP model's prediction results' number one feature is the word impressive
, followed by rent
. Essentially, this breaks the black box of the DL model, giving much confidence to the usage of DL models in production.
- Model deployment and serving in production: During the production stage, if the explainability of the model prediction can be readily provided to users, then not only will the usability (user-friendliness) of the model be improved, but also, we can collect better feedback data as users are more incentivized to give more meaningful feedback. A good explainability solution should provide point-level decisions for any prediction outcome. This means that we should be able to answer why a particular person's loan is rejected and how this rejection compares to other people in a similar or different age group. So, the challenge is to have explainability as one of the gated deployment criteria for releasing a new version of the model. However, unlike accuracy metrics, it is very difficult to measure explainability as scores or thresholds, although certain case-based reasoning could be applied and automated. For example, if we have certain hold-out test cases where we expect the same or similar explanations regardless of the versions of the model, then we could use that as a gated release criterion.
- Model validation and A/B testing: During online experimentation and ongoing production model validation, we would need explainability to understand whether the model has been applied to the right data or whether the prediction is trustworthy. Usually, ML/DL models encode complex and non-linear relationships. During this stage, it is often desirable to understand how the model influences the metrics of user behavior (for example, a higher conversion rate on a shopping website). Influence sensitivity analysis could provide insights regarding whether a certain user feature such as a user's income has a positive or negative impact on the outcome. If during this stage, we found, for some reason, that higher incomes cause a negative loan approval rate or a lower conversion rate, then this should be automatically flagged. However, automated sensitivity analysis during model validation and A/B testing is still not widely available and remains a challenging problem. A few vendors such as TruEra provide potential solutions to this space.
- Monitoring and feedback loops: While model performance metrics and data characteristics are of importance here, explainability can provide an incentive for users to provide valuable feedback and user behavior metrics to identify drivers and causes of model degradation if there are any. As we know, ML/DL models are prone to overfitting and cannot generalize well beyond their training data. One important explainability solution during model production monitoring is to measure how feature importance shifts across different data splits (for example, pre-COVID versus post-COVID). This can help data scientists to identify where degradation in model performance is due to changing data (such as a statistical distribution shift) or changing relationships between variables (such as a concept shift). A recent example provided by TruEra (https://truera.com/machine-learning-explainability-is-just-the-beginning/) illustrates that a loan model changes its prediction behavior due to changes in people's annual income and loan purposes before and after the COVID periods. This explainability of Feature Importance Shift greatly helps to identify the root causes of changes in model behavior during the model production monitoring stage.
In summary, DL explainability is a major challenge where ongoing research is still needed. However, MLflow's integration with SHAP now provides a ready-to-use tool for practical DL applications, which we will cover in our advanced chapter later in this book.
Summary
In this opening chapter, we implemented our first DL model by following the pretrain plus fine-tuning core DL development paradigm using PyTorch lightning-flash
for a text sentiment classification model. We learned about the five stages of the full life cycle of DL. We defined the concept of MLOps along with the three foundation layers and four ML/DL pillars, where MLflow plays critical roles in all four pillars (data, model, code, and explainability). Finally, we described the challenges in DL data, model, code, and explainability.
With the knowledge and first DL model experience gained in this chapter, we are now ready to learn about and implement MLflow in our DL model in the following chapters. In the next chapter, we will start with the implementation of a DL model with MLflow autologging enabled.
Further reading
To further your knowledge, please consult the following resources and documentation:
- On the Opportunities and Risks of Foundation Models (Stanford University): https://arxiv.org/abs/2108.07258
- MLOps: not as Boring as it Sounds: https://itnext.io/mlops-not-as-boring-as-it-sounds-eaebe73e3533
- AI is Driving Software 2.0… with Minimal Human Intervention: https://www.datasciencecentral.com/profiles/blogs/ai-is-driving-software-2-0-with-minimal-human-intervention
- MLOps: Continuous delivery and automation pipelines in machine learning (Google): https://cloud.google.com/architecture/mlops-continuous-delivery-and-automation-pipelines-in-machine-learning
- Deep Learning Development Cycle (Salesforce): https://metamind.readme.io/docs/deep-learning-dev-cycle
- MLOps – The Missing Piece In The Enterprise AI Puzzle: https://www.forbes.com/sites/janakirammsv/2021/01/05/mlopsthe-missing-piece-in-the-enterprise-ai-puzzle/?sh=3d5c89dd24ad
- MLOps: What It Is, Why It Matters, and How to Implement It: https://neptune.ai/blog/mlops
- Explainable Deep Learning: A Field Guide for the Uninitiated: https://arxiv.org/abs/2004.14545
- Machine learning explainability is just the beginning: https://truera.com/machine-learning-explainability-is-just-the-beginning/
- AI Fairness — Explanation of Disparate Impact Remover: https://towardsdatascience.com/ai-fairness-explanation-of-disparate-impact-remover-ce0da59451f1
- Datasheets for Datasets: https://arxiv.org/pdf/1803.09010.pdf