Cyber Security and Digital Forensics are two topics of increasing importance. Digital forensics especially, is getting more and more important, not only during law enforcement investigations, but also in the field of incident response. During all of the previously mentioned investigations, it's fundamental to get to know the root cause of a security breach, malfunction of a system, or a crime. Digital forensics plays a major role in overcoming these challenges.
In this book, we will teach you how to build your own lab and perform profound digital forensic investigations, which originate from a large range of platforms and systems, with the help of Python. We will start with common Windows and Linux desktop machines, then move forward to cloud and virtualization platforms, and end up with mobile phones. We will not only show you how to examine the data at rest or in transit, but also take a deeper look at the volatile memory.
Python provides an excellent development platform to build your own investigative tools because of its decreased complexity, increased efficiency, large number of third-party libraries, and it's also easy to read and write. During the journey of reading this book, you will not only learn how to use the most common Python libraries and extensions to analyze the evidence, but also how to write your own scripts and helper tools to work faster on the cases or incidents with a huge amount of evidence that has to be analyzed.
Let's begin our journey of mastering Python forensics by setting up our lab environment, followed by a brief introduction of the Python ctypes.
If you have already worked with Python ctypes and have a working lab environment, feel free to skip the first chapter and start directly with one of the other chapters. After the first chapter, the other chapters are fairly independent of each other and can be read in any order.
As a base for our scripts and investigations, we need a comprehensive and powerful lab environment that is able to handle a large number of different file types and structures as well as connections to mobile devices. To achieve this goal, we will use the latest Ubuntu LTS version 14.04.2 and install it in a virtual machine (VM). Within the following sections, we will explain the setup of the VM and introduce Python virtualenv, which we will use to establish our working environment.
To work in a similar lab environment, we suggest you to download a copy of the latest Ubuntu LTS Desktop Distribution from http://www.ubuntu.com/download/desktop/, preferably the 32-bit version. The distribution provides a simple-to-use UI and already has the Python 2.7.6 environment installed and preconfigured. Throughout the book, we will use Python 2.7.x and not the newer 3.x versions. Several examples and case studies in this book will rely on the tools or libraries that are already a part of the Ubuntu distribution. When a chapter or section of the book requires a third-party package or library, we will provide the additional information on how to install it in the virtualenv (the setup of this environment will be explained in the next section) or on Ubuntu in general.
For better performance of the system, we recommend that the virtual machine that is used for the lab has at least 4 GB of volatile memory and about 40 GB of storage.
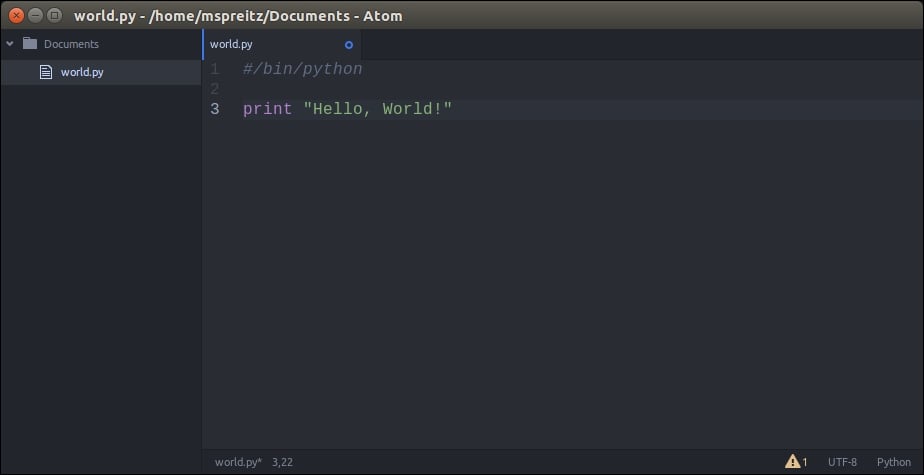
Figure 1: The Atom editor
To write your first Python script, you can use a simple editor such as vi or a powerful but cluttered IDE such as eclipse. As a really powerful alternative, we would suggest you to use atom, a very clean but highly customizable editor that can be freely downloaded from https://atom.io/.
According to the official Python documentation, Virtual Environment is a tool to keep the dependencies required by different projects in separate places by creating virtual Python environments for them. It solves the "Project X depends on version 1.x, but Project Y needs 4.x" dilemma and keeps your global site-packages directory clean and manageable.
This is also what we will use in the following chapters to keep a common environment for all the readers of the book and not run into any compatibility issues. First of all, we have to install the virtualenv package. This is done by the following command:
user@lab:~$ pip install virtualenv
We will now create a folder in the users' home directory for our virtual Python environment. This directory will contain the executable Python files and a copy of the pip library, which can be used to install other packages in the environment. The name of the virtual environment (in our case, it is called labenv) can be of your choice. Our virtual lab environment can be created by executing the following command:
user@lab:~$ virtualenv labenv New python executable in labenv/bin/python Installing setuptools, pip...done.
To start working with the new lab environment, it first needs to be activated. This can be done through:
user@lab:~$ source labenv/bin/activate (labenv)user@lab:~$
Now, you can see that the command prompt starts with the name of the virtual environment that we activated. From now on, any package that you install using pip will be placed in the labenv folder, isolated from the global Python installation in the underlying Ubuntu.
Throughout the book, we will use this virtual python environment and install new packages and libraries in it from time to time. So, every time you try to recap a shown example remember or challenge to change into the labenv environment before running your scripts.
If you are done working in the virtual environment for the moment and you want to return to your "normal" Python environment, you can deactivate the virtual environment by executing the following command:
(labenv)user@lab:~$ deactivate user@lab:~$
This puts you back in the system's default Python interpreter with all its installed libraries and dependencies.
If you are using more than one virtual or physical machine for the investigations, the virtual environments can help you to keep your libraries and packages synced with all these workplaces. In order to ensure that your environments are consistent, it's a good idea to "freeze" the current state of environment packages. To do this, just run:
(labenv)user@lab:~$ pip freeze > requirenments.txt
This will create a requirements.txt
file, which contains a simple list of all the packages in the current environment and their respective versions. If you want to now install the same packages using the same version on a different machine, just copy the requirements.txt
file to the desired machine, create the labenv environment as described earlier and execute the following command:
(labenv)user@lab:~$ pip install -r requirements.txt
Now, you will have consistent Python environments on all the machines and don't need to worry about different library versions or other dependencies.
After we have created the Ubuntu virtual machine with our dedicated lab environment, we are nearly ready to start our first forensic analysis. But before that, we need more knowledge of the helpful Python libraries and backgrounds. Therefore, we will start with an introduction to the Python ctypes in the following section.
According to the official Python documentation, ctypes is a foreign function library that provides C compatible data types and allows calling functions in DLLs or shared libraries. A foreign function library means that the Python code can call C functions using only Python, without requiring special or custom-made extensions.
This module is one of the most powerful libraries available to the Python developer. The ctypes library enables you to not only call functions in dynamically linked libraries (as described earlier), but can also be used for low-level memory manipulation. It is important that you understand the basics of how to use the ctypes library as it will be used for many examples and real-world cases throughout the book.
In the following sections, we will introduce some basic features of Python ctypes and how to use them.
Python ctypes export the cdll
and on Windows windll
or respectively oledll
objects, to load the requested dynamic link libraries. A dynamically linked library is a compiled binary that is linked at runtime to the executable main process. On Windows platforms, these binaries are called Dynamic Link Libraries (DLL) and on Linux, they are called shared objects (SO). You can load these linked libraries by accessing them as the attributes of the cdll
, windll
or oledll
objects. Now, we will demonstrate a very brief example for Windows and Linux to get the current time directly out of the time
function in libc
(this library defines the system calls and other basic facilities such as open
, printf
, or exit
).
Note that in the case of Windows, msvcrt
is the MS standard C library containing most of the standard C functions and uses the cdecl
calling convention (on Linux systems, the similar library would be libc.so.6
):
C:\Users\Admin>python >>> from ctypes import * >>> libc = cdll.msvcrt >>> print libc.time(None) 1428180920
Windows appends the usual .dll
file suffix automatically. On Linux, it is required to specify the filename, including the extension, to load the chosen library. Either the LoadLibrary()
method of the DLL loaders should be used or you should load the library by creating an instance of CDLL
by calling the constructor, as shown in the following code:
(labenv)user@lab:~$ python >>> from ctypes import * >>> libc = CDLL("libc.so.6") >>> print libc.time(None) 1428180920
As shown in these two examples, it is very easy to be able to call to a dynamic library and use a function that is exported. You will be using this technique many times throughout the book, so it is important that you understand how it works.
When looking at the two examples from the earlier section in detail, you can see that we use None
as one of the parameters for a dynamically linked C library. This is possible because None
, integers
, longs
, byte strings
, and unicode strings
are the native Python objects that can be directly used as the parameters in these function calls. None
is passed as a C, NULL pointer
, byte strings
, and unicode strings
are passed as pointers to the memory block that contains their data (char *
or wchar_t *
). Python integers
and Python longs
are passed as the platform's default C int type
, their value is masked to fit into the C type. A complete overview of the Python types and their corresponding ctype types can be seen in Table 1:
Table 1: Fundamental Data Types
This table is very helpful because all the Python types except integers
, strings
, and unicode strings
have to be wrapped in their corresponding ctypes type so that they can be converted to the required C data type in the linked library and not throw the TypeError
exceptions, as shown in the following code:
(labenv)user@lab:~$ python >>> from ctypes import * >>> libc = CDLL("libc.so.6") >>> printf = libc.printf >>> printf("An int %d, a double %f\n", 4711, 47.11) Traceback (most recent call last): File "<stdin>", line 1, in <module> ctypes.ArgumentError: argument 3: <type 'exceptions.TypeError'>: Don't know how to convert parameter 3 >>> printf("An int %d, a double %f\n", 4711, c_double(47.11)) An int 4711, a double 47.110000
Unions and Structures are important data types because they are frequently used throughout the libc
on Linux and also in the Microsoft Win32 API.
Unions are simply a group of variables, which can be of the same or different data types, where all of its members share the same memory location. By storing variables in this way, unions allow you to specify the same value in different types. For the upcoming example, we will change from the interactive Python shell to the atom editor on our Ubuntu lab environment. You just need to open atom editor, type in the following code, and save it under the name new_evidence.py
:
from ctypes import * class case(Union): _fields_ = [ ("evidence_int", c_int), ("evidence_long", c_long), ("evidence_char", c_char * 4) ] value = raw_input("Enter new evidence number:") new_evidence = case(int(value)) print "Evidence number as a int: %i" % new_evidence.evidence_int print "Evidence number as a long: %ld" % new_evidence.evidence_long print "Evidence number as a char: %s" % new_evidence.evidence_char
If you assign the evidence
union's member variable evidence_int
a value of 42
, you can then use the evidence_char
member to display the character representation of that number, as shown in the following example:
(labenv)user@lab:~$ python new_evidence.py Enter new evidence number:42 Evidence number as a long: 42 Evidence number as a int: 42 Evidence number as a char: *
As you can see in the preceding example, by assigning the union a single value, you get three different representations of that value. For int
and long
, the displayed output is obvious but for the evidence_char
variable, it could be a bit confusing. In this case, '*'
is the ASCII character with the value of the equivalent of decimal 42
. The evidence_char
member variable is a good example of how to define an array
in ctypes. In ctypes, an array is defined by multiplying a type by the number of elements that you want to allocate in the array. In this example, a four-element character array was defined for the member variable evidence_char
.
A structure is very similar to unions, but the members do not share the same memory location. You can access any of the member variables in the structure using dot notation, such as case.name
. This would access the name
variable contained in the case
structure. The following is a very brief example of how to create a structure
(or struct, as they are often called) with three members: name
, number
, and investigator_name
so that all can be accessed by the dot notation:
from ctypes import * class case(Structure): _fields_ = [ ("name", c_char * 16), ("number", c_int), ("investigator_name", c_char * 8) ]
Tip
Downloading the example code
You can download the example code files from your account at http://www.packtpub.com for all the Packt Publishing books you have purchased. If you purchased this book elsewhere, you can visit http://www.packtpub.com/support and register to have the files e-mailed directly to you.
In the first chapter, we created our lab environment: a virtual machine running Ubuntu 14.04.2 LTS. This step is really important as you can now create snapshots before working on real evidence and are able to roll back to a clean machine state after finishing the investigation. This can be helpful, especially, when working with compromised system backups, where you want to be sure that your system is clean when working on a different case afterwards.
In the second part of this chapter, we demonstrated how to work with Python's virtual environments (virtualenv) that will be used and extended throughout the book.
In the last section of this chapter, we introduced the Python ctypes to you, which is a very powerful library available to the Python developer. With those ctypes, you are not only able to call functions in the dynamically linked libraries (available Microsoft Win32 APIs or common Linux shared objects), but they can also be used for low-level memory manipulation.
After completing this chapter, you will have a basic environment created to be used for the rest of the book, and you will also understand the fundamentals of Python ctypes that will be helpful in some of the following chapters.