Welcome to Instant R Starter. This book has been created especially to provide you with all the information that you need to set up with R. You will learn the basics of R, get started with manipulation of R objects, write your own functions, and discover some tips and tricks for using R.
This document contains the following sections:
So, what is R? shows you what R actually is, what you can do with it, and why it’s so great.
Installation teaches you how to download and install R with a few easy steps and then set it up so that you can use it as soon as possible.
Quick start – R language will make you familiar with the R language. You will learn how to create different objects (vectors, matrices, data frames) and you will see some practical examples of their manipulation and use.
Top 5 features you need to know about will teach you how to perform five tasks with the most important features of R. By the end of this section you will be able to perform data input and output in the system, use flow control and loops, write your own functions and debug them, and create basic plots.
People and places you should get to know provides you with many useful links to the project’s page and forums, as well as a number of helpful guides, tutorials, blogs, and the Twitter feeds about R, as every open-source project is centered around a community of users.
R is a high-level language and environment for data analysis and visualization. It provides an environment in which you can perform statistical analysis and produce high-quality graphics. It is actually a complete programming language derived from the statistical programming language S. It has been developed and is maintained by a core of statistical programmers, with the support of a large community of users. It is most widely used for statistical computing and graphics, but is a fully functional programming language well suited for scientific programming, data management, and data visualization in general.
The interaction with the R system is mainly command-driven, with the user typing in text and asking R to execute the specific command. As soon as you start R, a session will be opened on which the commands may be introduced and the expression executed. Complex procedures can be implemented in the scripts, which are executed as soon as they are loaded into the system or, more efficiently, as functions, which can be loaded in the system and used when needed.
R can do a lot more than what a typical beginner can be expected to need. R also comes with extensive online help in text form as well as in HTML files. The help pages can be accessed via the Help section in the cascade menu or via the help.start()
command on the console. You will notice that all the help pages present the same structure, and if they don't appear to be clear at a first glance, they contain all the main information you will need. Normally, all the functions as well as the datasets contained in the packages have their own specific help file. The easiest way to access such pages is to call the help()
function with an argument as the name of the R object for which you desire help; for example, help for the function mean()
can be found with the command help(mean)
. As an alternative, the prefix form ?mean
is also available. Within R, there is also an option available that searches for all the functions containing a specific word; for example, with the command apropos("mean")
, it is possible to search for all the functions containing the word mean
. Remember that all the help pages are available only if the package containing the function is loaded in the workspace.
Additionally, a series of manuals are also available in the Help section of the cascade menu. Of particular interest for a beginner is the manual An introduction to R, which contains an introduction to the R language and environment. For a more advanced usage, the Writing R Extensions manual is extremely useful, which is focused on the creation of R packages.
An R package is a collection of source code and other additional files that, when installed in R, allow the user to load them in the workspace via a call to the library()
function. An example of code to load the package lattice
may be found as follows:
> library(lattice)
An R installation contains one or more libraries of packages. Some of these packages are part of the basic installation and are loaded automatically as soon as the session is started. Others can be installed from the CRAN, the official R repository, or downloaded and installed manually. Package files are compiled as a .zip
file on Windows, as .pkg
for Mac OS, or as a source code package for Linux X. In Windows, the manual installation can be performed by simply downloading the .zip
file and loading it on R by following the path Packages | Install Package(s) from local ZIP files in the cascade menu. Anyhow, in general, the installation via the CRAN repository should be preferred, since in this way it will be downloaded automatically. Also the dependency of the package you are installing will be downloaded automatically, which otherwise should be installed manually with the manual installation.
In this section, we will see how you can obtain and install R on your computer. There are two main ways to install R; by downloading the binary distribution or by compiling the software from a source. Both methods will be described in the following sections.
R can be obtained by downloading it from the CRAN website (http://www.r-project.org/). The Comprehensive R Archive Network (CRAN) is a network of FTP and web servers around the world that store identical, up-to-date versions of code and documentation for R. The CRAN is directly accessible from the R website, and on such a website it is also possible to find information about R, some technical manuals, the R journal, and details about the packages developed for R and stored on the CRAN repositories. You can find detailed information of such websites in the section People and places you should get to know.
Binary distribution will very likely be the one for you. It is a compiled version of R that can be downloaded and installed directly on your system.
For a Windows system, this version comes as a unique .exe
(downloadable from the CRAN website) file, which can be easily installed by double-clicking on it and following the few steps of the installation. Once the process is completed, you can start R via the icon on your desktop or via its location in the list of programs available on the system.
For a Mac OS X system, R is also available as a unique installation file, .pkg
, which can be downloaded and installed on the system.
For a Linux system, there are several versions of the installation file. In the download section, it is necessary to select the appropriate version of R depending on the Linux distribution. Installation files are available in two main formats, .rpm
for Fedora, Suse, or Mandriva and .deb
for Ubuntu, Debian, and Linux Mint.
Installation of R from source code is possible on all supported platforms, although it is not very easy on Windows since the installation tools are not part of the system. Detailed information on the process and the tools required are available on the CRAN website. On Unix-like systems, the process is much simpler; the installation must be performed following the usual steps:
./configure make make install
Such procedures work assuming that the relevant compilers and support libraries are available and correctly installed. A more detailed description of the installation procedure can be found on the CRAN website (http://www.r-project.org/).
As you will see, there are constantly new versions of R released thanks to the work of the development community. It would be best if you download and install the most recent release; at the time of writing this book, the latest version is the 2.15.2 one. In case you already have R installed on your computer, you can check its version by navigating to Help | About in the cascade menu or by typing R.Version()
on the R console. This function can be particularly useful in case you would need to write some code to extract the version number automatically from the system; for example, to verify that the user is not using a version of R older than the one you used to write your code.
Once you have R installed on your system, it may be interesting for you to check the differences of the current version from the previous one. If this may be negligible at the beginning, this may become quite important as soon as you become familiar with R and you have defined your way of interacting with the system. Also, this may become important if you would like to check that some code you wrote in the past is still working properly. Usually the changes of each version from the previous one are referred to as "news". You can access the news of the current R version by simply calling the function news()
without any argument.
One of the main reasons why R is a very powerful and advanced environment is the numerous extensions that can be added to the basic environment via extension packages. There are two main methods to install such packages in R. Most of them are available on the CRAN repositories; so in this case the desired package can simply be chosen from the list of available packages by navigating to Packages | Install package(s). Installation of a package from the CRAN may also be launched from the R console. In both cases, the Internet connection needs to be active and for Linux systems you should have root rights. For example, to install the package lattice
we can use the following command:
> install.packages("lattice")
If the package is not available on the CRAN, or if you would need to install the package directly from the package file, it can be done on the R GUI in Windows by navigating to Packages | Install Package(s) from local zip files. The extension of the package file may be different depending on the operating system; please check the section Binary Distribution for more details.
As soon as you become more experienced with R, you may notice that the GUI development environment provided together with R may become quite difficult to use, especially if you have to deal with several scripts at the same time. For this reason, it may become useful for you to start using Integrated Development Environment (IDE). The number of specific IDEs that get integrated with R is still limited, but some of them are quite efficient, well designed, and open source. The three most important IDEs for R are RStudio, Eclipse, and Emacs.
RStudio is probably the only development environment developed specifically for R. It is available for all the major platforms (Windows, Linux, and Mac OS X) and it can be run on a local machine such as your computer or even over the Web using RStudio Server. With RStudio Server you can provide a browser-based interface (the RStudio IDE) to a version of R running on a remote Linux server.
For information about where to download this program, please refer to the section People and places you should get to know. It allows you to integrate several useful functionalities that are extremely useful, especially if you use R for some more complex projects. The environment is composed of four different areas:
Scripting area: In this area you can open, create, and write your scripts.
Console area: This area is the actual R console on which the commands are executed.
Workspace/History area: In this area you can find a practical summary of all the objects created in the workspace in which you are working.
Visualization area: Here you can easily load packages and open R help files, but even more importantly, you can visualize plots.
Thanks to such an area separation, RStudio allows you to efficiently manage the several components you will have to deal with, such as scripts, commands, plots, and so on.
For more details on how to install and use RStudio, you can refer to the official website of the project, on which you can find useful video tutorials and documentation. Let us take a look at the following screenshot that shows the RStudio on Windows 8:
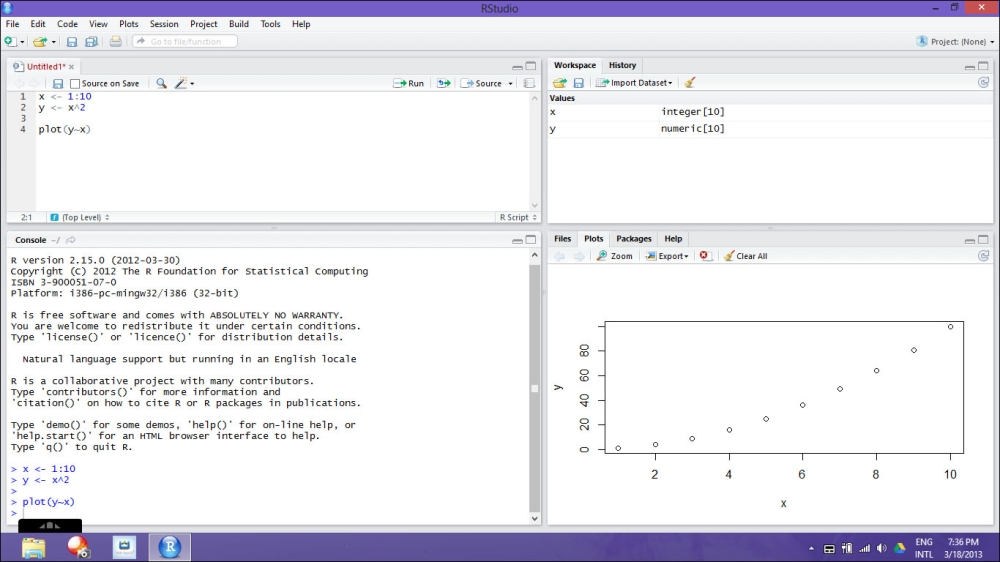
Eclipse is an open source IDE that was originally developed for Java, but was later extended to other applications and programming languages such as C++ and Python as well. Eclipse is structured in such a way that it is particularly useful for the management of complex programming projects, for example, when you have your projects divided in several folders, since Eclipse allows you to keep a grip on all the files simultaneously. One inconvenience of such a development environment is probably its huge size (around 200 MB) and a slightly slow start-up time for the environment.
Eclipse does not support natively the interaction with R; so in order to be able to write your code and execute it directly in the R shell, you need to add StatET to your basic Eclipse installation. StatET is a plugin for the Eclipse IDE and it offers a set of tools for R coding and package building. More detailed information on how to install Eclipse and StatET and how to configure the connections between R and Eclipse can be found on the websites of the relative projects listed in the section People and places you should get to know.
Emacs is a customizable text editor that is very popular particularly in the Linux environment. Although this text editor appears with a very simple GUI, it is an extremely powerful environment, particularly thanks to the numerous key shortcuts that allow interacting with the environment in a very efficient manner. Also, if a normal desktop computer IDE such as RStudio is more complete for a better graphical visualization of R results, Emacs may be useful in case you will need to work with R on systems with a poor graphical interface, such as servers. Along with Eclipse, Emacs, by default, does not support the interface with R, so you will need to install on your Emacs an add-on package such as Emacs Speaks Statistics (ESS), which will allow for doing that. ESS is designed to support the editing of scripts and interaction with various statistical analysis programs including R.
The purpose of this section is to learn the basic syntax of the R language and how to interact with the console. If you are a beginner, you would not need any additional IDE; on the contrary it would be better for you to use the basic R interface so that you will get a chance to get familiar with the basic software.
In this section, you will begin your first interaction with the R console and you will find a description of the basics of this programming language.
As soon as you start R, you will see that a workspace is open; you can see a screenshot of the R Console window in the following image. The workspace is the environment in which you are working; where you will load your data and create your variables. As soon as you close R, the program will ask you if you want to save the workspace on the hard drive of the computer. Loading a previously saved workspace will allow you to find again all the objects that you have created in the previous session.
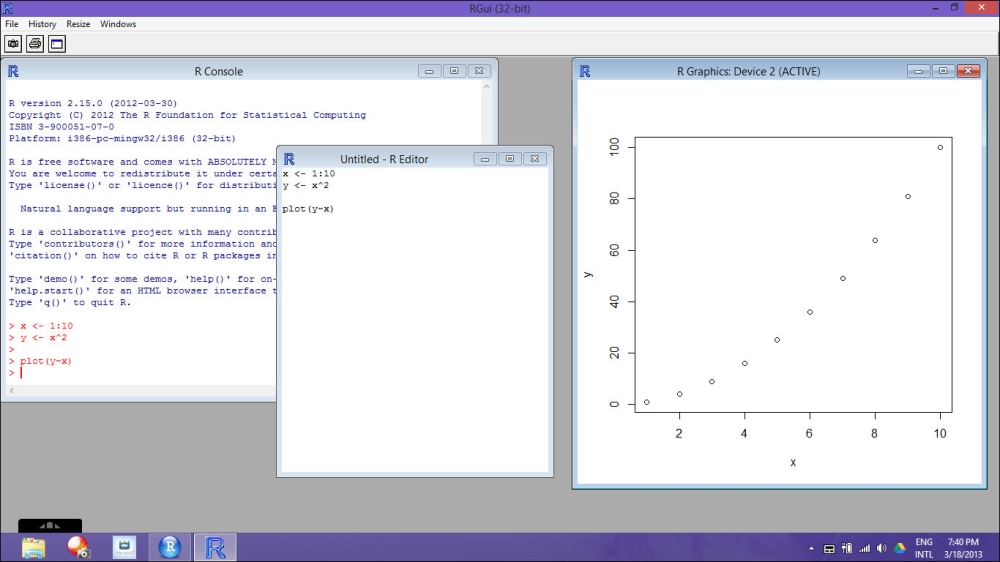
You can save the workspace in the cascade menu by navigating to File | Save Workspace…. Alternatively, you can also save the workspace with the command save.image()
. This will create a file called .RData
in your working directory. The working directory is basically the location on your computer where R is operating. This means that in such a directory, R is able to read and write files. You can identify which is your working directory with the help of the command getwd()
. You may change your working directory with the command setwd("dir")
, where "dir"
is the directory address. While this last option may be very useful within script files, in a more common situation it will be easier to change the working directory via the GUI by navigating to File | Change dir….
Now that you have already typed some commands in the R shell, you will probably have already noticed a couple of things. R is case sensitive; this means that if you type Setwd()
instead of setwd()
, you will get an error. You will also have probably noticed that most of the commands you saw end with a couple of parentheses. The parentheses indicate that what you are using is not an object, but a function. Within the parentheses, you may specify the arguments of the function (an example is the function library()
that you have already encountered previously), while if the function doesn't need any argument or if you intend to use only default values, you don't need any argument within the parentheses. We will go more in detail on this matter in the section Top features you'll want to know about. Finally you also noticed that typing your command directly in the R shell is not really optimal. One efficient way of interacting with the shell is via scripts. In this method, you can write your code in a separate window and then you can execute the code in the shell, so that if you would need to save your code or execute it several times you will not have to type it again. You can do that also in the basic R interface by navigating to File | New script. Once you have some commands in the script window, you will need to execute them in the console. Of course you can do copy-paste as usual, but it is better to select with your mouse the code you intend to execute and press the keys Ctrl + R. Alternatively, you can also right-click in the window and select Run line or selection.
These are some additional functions that you can use to keep an eye on your workspace. Let's create a variable, x
, with a numeric value using the following code:
> x <- 10
If you would like to know which variables you have created in the current session, you can use the command objects()
or ls()
, while if you would like to see which libraries and data frames are attached in the workspace, you can use the command search()
. R keeps a record of all the commands you type. For example, to save the history in a file named myHistory
, use the savehistory(file = "myHistory")
command, and to load the history file named myHistory, use loadhistory(file = "myHistory")
. If you save your workspace image when quitting, then your current history will be saved in .Rhistory
in the current working directory.
The screen prompt, >
, is the R prompt that waits for commands. In this book, all the R code that is typed in this prompt will be preceded by the symbol >
. The [1]
that prefixes the output indicates that this is item one in a vector of output, and will be reported together with the output results to make them easier to be identified. All the functions will be followed by double brackets in the text.
On the start-up screen, you can either type any function, command, or you can use R to perform basic calculations. R uses the usual symbols for addition (+
), subtraction (-
), multiplication (*
), division (/
), and exponentiation (^
). Parentheses ( )
can be used to specify the order of operations. R also provides %%
for taking the modulus and %/%
for integer division. Comments in R are defined by the character #
; so everything after such characters up to the end of the line will be ignored by R.
R has a number of built-in functions, such as sin(x)
, cos(x)
, tan(x)
, (all in radians), exp(x)
, log(x)
, and sqrt(x)
. Some special constants such as pi
are also predefined. You can see an example of the use of such a function in the following code:
> exp(2.5) [1] 12.18249
You can write a command on each line, or you can divide longer lines of commands in more than one line if the line is incomplete according to the R language (for example, with a comma, an operator, or with more left parentheses than right parentheses, implying that more right parentheses will follow). When the submitted line is not complete, the R prompt changes from >
to +
. For example, you can divide a sum on two lines by pressing Enter
after each number:
> 5+ + 5 [1] 10
If you have made a mistake and you want to get rid of the +
prompt and return to the >
prompt, either press the Esc key or the Stop button in the GUI.
As you already have probably noticed, in R, a certain value is assigned to an object by assignment. This is achieved by the arrow operator <-
, which is a composite symbol made up of the less than sign and minus sign with no space between them. Thus, to create a scalar constant x
with a value 5
, we type:
> x<-5
The command x=5
also works in R, but the arrow option is usually preferred. The direction of the arrow represents the direction of the assignment; so x<-5
and 5->x
have the same result. Be aware that there is a potential ambiguity if you get the spacing wrong. In fact, comparing the assignment x<-5
, "x gets 5", with x < -5
becomes a logical question, which is asking if x is less than minus 5, and will produce an answer TRUE
or FALSE
.
At this point, you have obtained an idea of the way R works, and you have already learned how you can specify the arguments within a function. Many things in R are done via function calls. The format of such calls is the name of the function followed by a parenthesis containing the arguments separated by a comma. The arguments passed to the function may be assigned directly within the body of the function or they can be defined in the workspace and then recalled within the function.
One example of a function with multiple arguments is the function rnorm
. Such a function requires the arguments rnorm(n, mean, sd)
and can be used to simulate n
values from a distribution with mean and standard deviation provided within the function. You could provide such arguments in two different ways:
> rnorm(n=10, mean=0, sd=1)
or
> rnorm(10, 0, 1)
In the second way, since the values are not associated with a specific argument, R will assume that they will be provided in an order, first n
, then mean
, and finally sd
. This is known as positional matching. Also, keep in mind that if the function does not require any argument, you will still need to include the parenthesis after the function name. By executing only the name of the function (without brackets), you will have access to the actual code, which is defined in the function itself. This may be helpful in some cases if you would like to know what exactly a function does.
Many packages, external or included in the basic R distribution, come with built-in datasets. In some cases the data is already available in the R workspace directly, while in some other situations it requires a specific call to the data
function with the name of the dataset to load as an argument. Particularly at the beginning of your learning experience (but also once you become more experienced), it may be very handy to have access to the same data, particularly if you would like to do some tests with the function that you are just learning. You can access the list of the available datasets with a short description of the data by just using the command data()
.
In some cases, you may find out that the calculation you perform in R may lead to a special value: infinity. Such a value is represented in R by Inf
or –Inf
. You can easily see an example of that by dividing a number by zero:
> 17/0 [1] Inf
The value infinity, as you have seen, may be returned by R during a calculation, but it may also be provided as an argument within calculations:
> exp(-Inf) [1] 0
Some other calculations may lead to results that are not numbers. Such quantities are defined in R as "Not a Number", and are represented by NaN
. Some typical examples of such calculations are:
> 0/0 [1] NaN > Inf/Inf [1] NaN
A completely different situation is the one for which a certain value is not available, for example, in data collection. Such a missing value is represented by NA
. You need to clearly understand the difference between NA
and NaN
, since they refer to different classes of values and they are treated differently in R. Missing values may be represented with the characters NA
in the data, and in such a case, normally R will import them as NA
values. In some cases, the values that are not identified, such as dots or blank spaces in the data sheet, may be read as NA
values in R. Such values will be particularly important in statistical tests and data manipulation.
In R, there are a series of functions with the objective of testing objects for all these different classes of values. These are some examples of such functions:
> x <- Inf > y <- NaN > z <- NA > is.infinite(x) [1] TRUE > is.nan(y) [1] TRUE > is.na(z) [1] TRUE
A logical expression is formed using the comparison operators <
, >
, <=
, >=
, ==
(equal to), and !=
(not equal to), and the logical operators &
(and), |
(or), and !
(not). The order of operations can be controlled using parentheses ()
.
The value of a logical expression is either TRUE
or FALSE
. The integers 1
and 0
can also be used to represent TRUE
and FALSE
respectively (which is an example of what is called coercion). This is an easy example of a logical expression:
> c(1,2,3,4)==3 [1] FALSE FALSE TRUE FALSE
The previous example also shows how logical expressions can be applied to vectors, generating logical vectors of TRUE
/FALSE
values.
In every computer language, variables provide ways and means of accessing the data stored in memory. R does not provide direct access to the computer's memory, but rather provides a number of specialized data structures that we will refer to as objects. These objects are referred to through symbols or variables.
The basic object in R is the vector; even scalars are vectors of length 1. Vectors can be thought of as a series of data of the same class. There are six basic vector types (called atomic vectors): logical, integer, real, complex, string (or character), and raw. Integer and real represent numeric objects; logicals are Boolean data types with a possible value of TRUE
or FALSE
. Among such atomic vectors, the more common ones are logical, string, and numeric (integer and real).
There are several ways to create vectors.
The operator :
(colon) is a sequence-generating operator; it creates sequences by incrementing or decrementing by one. The following are some examples:
> 1:10 [1] 1 2 3 4 5 6 7 8 9 10 > 5:-6 [1] 5 4 3 2 1 0 -1 -2 -3 -4 -5 -6
If the interval between the numbers is not 1, you can use the seq()
function. Its arguments are the initial value of the series, the final one, and the increment. You can also generate a downward sequence if the increment is negative. If the increment does not match exactly the final value that you provided, the sequence will stop at the last matching number before the final value. Following are some examples:
> seq(from=2, to=2.5, by=0.1) [1] 2.0 2.1 2.2 2.3 2.4 2.5 > seq(from=0, to=-2, by=-0.5) [1] 0.0 -0.5 -1.0 -1.5 -2.0 > seq(from=1, to=2.7, by=0.5) [1] 1.0 1.5 2.0 2.5
If the elements of your vector do not follow a specific series, or if they are not numeric, as alternative you may use the concatenation function c()
. In such a function, you simply list the values of your vector separated by a comma. Here are some examples of numeric, logical, and character vectors. Remember that in R, character strings are defined by double-quotation marks.
> c(10,8,3,5,2) > c(TRUE,FALSE,TRUE,TRUE) > c("R","Programming")
One additional alternative in the creation of vectors is via the rep()
function. Such a function will repeat a value (or a vector) n times. The vector to repeat and the number of repetitions are arguments to the function, which are provided to the function in the following order:
> rep(x=2, times=3) [1] 2 2 2 > vec <-c(3,10,4) > rep(x=vec, times=2) [1] 3 10 4 3 10 4 > rep(x=vec, times=2, each=3) [1] 3 3 3 10 10 10 4 4 4 3 3 3 10 10 10 4 4 4
One of the more important features of R is the possibility to use an entire vector as arguments of functions, thus avoiding the use of cyclic loops. For example, the use of some of these functions is reported as follows, but remember that most of the functions in R also allow the use of vectors as arguments. In case you have any doubt about which kind of data is allowed for a specific argument in a function, remember to check the help page of that function.
> x <- c(12,10,4,6,9) > max(x) [1] 12 > min(x) [1] 4 > mean(x) [1] 8.2
Although we can apply functions directly to vectors, there are some cases in which you will need to access specific elements of the vector. This is possible in R via subscripts or indices. Indices allow identifying a specific element of an R object, and the same concept applies to a vector and also to a more complex data structure. In a vector, which is a one-dimensional object, they represent the position of the element in the vector, while if the object has two dimensions (for example, in a matrix), you will need to specify two positions (row number and column number). Indices must be specified within the []
operator, and in R, the element index starts from 1
and not from 0
.
Remember, if a subscript appears as empty, it means that all the elements will be used; so when printing, the original vector remains unchanged while the negative index will exclude the element in that position. A couple of simple examples will make it clear how to use subscripts in vectors. Two-dimensional subscripts will be considered in the next sections.
> x <- c( 1,4,6,10) > x[2] [1] 4 > x[1:3] [1] 1 4 6 > x[-2] [1] 1 6 10
In R, the matrix notation is extended to elements of any kind, so it is possible to have a matrix of character strings. Matrices and arrays are basically vectors with a dimension attribute. You can see a very simple example of such an idea in the following code. The function dim()
in the example returns a vector indicating the dimension of an object:
> x <- c("a","b","c","d") > matrix(x,nrow=2) [,1] [,2] [1,] "a" "c" [2,] "b" "d" > dim(x) NULL > y<- matrix(x,nrow=2) > dim(y) [1] 2 2
As you have seen in the example, the function matrix()
may be used to create matrices. By default, such a function creates the matrix by column; as an alternative, it is possible to specify to the function to build the matrix by row:
> matrix(1:9,nrow=3,byrow=TRUE) [,1] [,2] [,3] [1,] 1 2 3 [2,] 4 5 6 [3,] 7 8 9
Some useful functions when working with matrices are the functions to rename rows or columns—rownames()
and colnames()
—and the transposition function t()
, which turns the rows into columns and columns into rows:
> x <- matrix(1:12,nrow=3) > x [,1] [,2] [,3] [,4] [1,] 1 4 7 10 [2,] 2 5 8 11 [3,] 3 6 9 12 > rownames(x) <- c("A","B","C") > colnames(x) <- c("a","b","c","d") > x a b c d A 1 4 7 10 B 2 5 8 11 C 3 6 9 12 > t(x) A B C a 1 2 3 b 4 5 6 c 7 8 9 d 10 11 12
Remember that in a matrix, as well as in vectors, all elements must be of the same class; so it is not possible to have characters and numeric data together. In the following example, the first line of code produces a numeric matrix, while in the second one, since a character is provided in the matrix, R forces the other values to change to characters (that is why they appear between quotes).
> matrix(c(1,2,3,4),nrow=2) [,1] [,2] [1,] 1 3 [2,] 2 4 > matrix(c(1,2,"c",4),nrow=2) [,1] [,2] [1,] "1" "c" [2,] "2" "4"
Considering two matrices, x
and y
, other useful operations are matrix multiplication, x%*%y
, transpose of a matrix, t(x)
, extracting the diagonal of a matrix, diag(x)
, inverse, solve(x)
, and determinant, det(x)
.
A list in R is a collection of different objects. One of the main advantages of lists is that the objects contained within a list may be of different types; for example, numeric and character values. In order to define a list, you simply will need to provide the object that you want to include as an argument of the function list()
:
> element1 <- c(1:9) > element2 <- c("a","b","c") > element3 <- matrix(1:9,nrow=3) > list(element1, element2, element3) [[1]] [1] 1 2 3 4 5 6 7 8 9 [[2]] [1] "a" "b" "c" [[3]] [,1] [,2] [,3] [1,] 1 4 7 [2,] 2 5 8 [3,] 3 6 9
As you can see, different elements may be combined together in one list object. Each of the listed objects is indicated with a double set of square brackets [[]]
. You can use such an index to access an object, or as an alternative, you can rename the elements of the list. In the following example, you will notice how you can access a specific element using its name and the operator $
:
> myList <- list(element1, element2, element3) > myList[[3]] [,1] [,2] [,3] [1,] 1 4 7 [2,] 2 5 8 [3,] 3 6 9 > names(myList) <- c("Vector1","Vector2","Matrix") > myList$Matrix [,1] [,2] [,3] [1,] 1 4 7 [2,] 2 5 8 [3,] 3 6 9
As an alternative, you may also provide the names of the objects within the list directly in the list()
function. Notice how the quotes are not necessary in this case:
> myList <- list(Vector1=element1, Vector2=element2, Matrix=element3)
A data frame corresponds to a data set; it is basically a special list in which the elements have the same length. Elements may be of different types in different columns, but within the same column, all the elements are of the same type. You can easily create data frames using the function data.frame()
, and you can recall a specific column using the operator $
.
> col1 <- c("A","B","C","D") > col2 <- 1:4 > col3 <- 10:13 > myData <- data.frame(col1,col2,col3) > myData col1 col2 col3 1 A 1 10 2 B 2 11 3 C 3 12 4 D 4 13 > myData$col2 [1] 1 2 3 4
Often, the data frame that you will use will be quite huge to be opened in the R console. Useful functions in such cases are the head()
and tail()
functions, which will visualize only a certain number of rows in the data; at the beginning (the head()
function) and at the end (the tail()
function) of the data frame. Both such functions have an argument n
, which defines the number of rows that should be visualized. As an alternative, the function fix()
will open a new window representing a spreadsheet with the data. Another alternative is the edit()
function, which invokes a text editor on an R object. A useful way to display a concise summary of a data frame (or a generic object) is to use the function str()
and the function summary()
. In the following code, you can find examples of such functions, which will be applied to one of the datasets available in the R environment, that is, the orange
dataset, which contains the data of growth of orange trees:
> head(Orange,n=6) Tree age circumference 1 1 118 30 2 1 484 58 3 1 664 87 4 1 1004 115 5 1 1231 120 6 1 1372 142 > tail(Orange, n=3) Tree age circumference 33 5 1231 142 34 5 1372 174 35 5 1582 177 > fix(Orange) > str(Orange) 'data.frame': 35 obs. of 3 variables: $ Tree : Ord.factor w/ 5 levels "3"<"1"<"5"<"2"<..: 2 2 2 2 $ age : num 118 484 664 1004 1231 ... $ circumference: num 30 58 87 115 120 142 145 33 69 111 ... > summary(Orange) Tree age circumference 3:7 Min. : 118.0 Min. : 30.0 1:7 1st Qu.: 484.0 1st Qu.: 65.5 5:7 Median :1004.0 Median :115.0 2:7 Mean : 922.1 Mean :115.9 4:7 3rd Qu.:1372.0 3rd Qu.:161.5 Max. :1582.0 Max. :214.0
As soon as you become more familiar with R, you will realize that there are a wide variety of things that you can do with it. This section will teach you all about the most commonly performed tasks and the most commonly used features in R. The code shown in these sections will be slightly longer and more complex compared to the one shown previously, as sometimes comments will also be included in the code. Remember that comments in R come after the symbol #
.
As you have already noticed, most of the potential of R is in data analysis and data manipulation. When working with data, you will sometimes need to save data to a file and/or read it from files. In this section, you will find an introduction on writing and reading data files. Additional information on this subject can also be found in the R Data Import/Export manual included in the software.
Most often, you will have to write datasets to a file, and the important functions that allow you to do that are write.table()
and write.csv()
. The first one is a more general function that can be used to create files in different formats, such as .txt
. The second one is basically a call to the first one with some specific arguments for the .csv
files. Such a format is particularly useful since it can be easily read and created with software such as Excel. Remember that the files that you create will be located in the current working directory. In order to check in which folder the working directory is, or to change the folder of a working directory, you can check the section Quick Start. Let's create some examples using such functions and the dataset Orange
, which we have already used in the previous example.
The basic call to the function write.table()
would be:
> write.table(Orange,"orange.txt")
You can check the presence of the new file, orange.txt
, with the command dir()
, which will list all the files in the working directory.
The file that will be created is a .txt
file; remember that the file extension must be included in the filename as well. If you open the file you just created (you can use any text editor), you will see that all the elements are delimited by quotes, and that a new column of consecutive numbers appears in the dataset. This column represents the row number. These two properties of the created file—quotes and row numbers—are defined respectively by the arguments row.names
and quote
, which by default are TRUE
. You can change these options by setting them to FALSE
:
> write.table(Orange, file="orange.txt", row.names=FALSE, quote=FALSE)
Another important option in the function write.table()
is the argument sep
. This argument allows you to choose the character to be used for separating elements within each row. For example, the following code will use the character --
as a separator; in each row, a comment explaining the meaning of each argument is also included:
> write.table(Orange, # Dataset to save + file="orange.txt", # Name of the file with extension + row.names=FALSE, # Numbering of each row + quote=FALSE, # Each element between quotes + sep="--") # Separating character
Other useful characters that are used as separators are \t
for a tabular separator, \n
for a new line, ,
(comma), and ;
(semicolon). Remember that the separator character must be included within quotes, as in the previous example. In order to create a data file with Comma Separated Values (CSV), you may also use the function write.csv()
; this function corresponds basically to a call to the function write.table()
using different default arguments, such as the separating character, in this case the comma. For example, to create a .csv
file for the dataset Orange
, you can use the following code:
> write.csv(Orange, file="orange.csv", row.names=FALSE, quote=FALSE)
Depending on the language selected in the regional settings of your computer, you may have a different separating character for decimal values. For instance, in the English or American language, you will have the dot character as a separator for decimals, and the .csv
files will use the comma as a separating value. In this case, you may use the function write.csv()
. In some other International page's options, such as German, Dutch or Italian, the decimal separator used by the system is a comma, so the .csv
files will use a semicolon to separate values. In these cases, you can change the default separating character in the write.csv()
function or you can use the function write.csv2()
, which by default will use the semicolon as a separating character. Remember that .csv
files can be easily opened using Excel as well, but if you would like to verify which character is actually used in the separation of the elements between columns, you will have to open the file with a normal text editor such as Notepad.
In some cases, you may need to write to the file's text data. In this case, a useful function is writeLines()
, which allows you to write text lines to a connection, for example, a file.
The most convenient way of reading data in R is using the function read.table()
. This function requires the data to be in the ASCII format, which will be created by any plain text editor. The way of using this function is very similar to write.table()
, as explained previously. In R, the result of the function read.table()
is a data frame, in which R expects to find the same type of data in each column (for example, character or numeric). Each element in each row is expected to be separated by some form of separator or a blank space. The first line of the file may contain a header giving the names of the variables (highly recommended). Let's assume that you created a datafile .txt
of the dataset Orange
using the default separator (you can use the code reported in the previous section Writing data to file.)
You would be able to import this dataset as a data frame within R using the following code:
> read.table(file="orange.txt", header=TRUE)
The previous code will print the data frame on the console, and if your intention is to save it as an object (as it is normally the case), you simply use the assignment operator:
> myData <- read.table(file="orange.txt", header=TRUE)
As you have seen in the previous code snippets, within the arguments of the function we used the option header=TRUE
in order to communicate to R that the first line of the data contains the name of the column. This option is particularly important because the header present in the original data will be used as names in the columns of the data frame created in R, meaning that using these names will allow you to access the data in R. For example, if you consider the data frame just created, that is, myData
, using the command head(myData)
, you will be able to check that actually the headers were recognized by R, and using the code myData$age
will allow you to access the second column of the data frame.
Along with the functions for writing data, in this case you have the functions read.csv()
and read.csv2()
, which can be used to easily read .csv
files with commas as separators (the first one) or semicolon (the second one). These functions will have the right separator character and will have the option header
as TRUE
by default.
Some additional functions that may be useful to read data are the readLines()
function, which may be used to read text lines from a connection, and the scan()
function, which reads data from the console or a file within a vector or a list.
In R, you can also write a vector in the Windows clipboard using the function writeClipboard(x)
, where x
is a character vector you want to paste. With this approach, you would be able to build up a spreadsheet in Excel one column at a time.
The vector provided to the function must be a character vector. You can see an example of the code in the following snippet, in which it is shown how to specify that. In the code, character.variable
and numeric.variable
represent a generic character and a numeric vector respectively:
> writeClipboard(as.character(character.variable)) > # Then paste in Excel using Ctrl+V > writeClipboard(as.character(numeric.variable)) > # Then paste in Excel using Ctrl+V
You can also create or modify data frames directly in R. This can be done by opening the data with the function fix()
. This function will open a GUI window with the data frame in it. If you want to create a new data frame and type in data, you can do it with the following code, where we create an empty data frame d
and then modify it:
> d <- data.frame() > fix(d)
After you type the values in Windows, you need to simply close the GUI and the data will be stored in the variable d
. You can also change the column name by clicking on it.
Flow control expressions are programming constructions that allow the conditional execution of a portion of code. In this section, you will find a description of the main flow-control expressions in R.
The R language is a true programming language that allows conditional execution and programming loops as well. It is, for instance, often useful to force the execution of some piece of code to depend on a certain condition. This can be done using the if..else
expression, which follows the following structure:
if (logical.expression) { expression.1 ... } else { expression.2 ... }
expression.1
will be executed if logical.expression
is TRUE
and expression.2
is FALSE
. In this construction, the else
statement may also be omitted; in this case, if logical.expression
is FALSE
, nothing will be executed.
Braces { }
are used to group together one or more expressions. If there is only one expression, the braces are optional. Several if
statements may also be nested, creating complex conditional code. Since the else
statement is optional, you will get an error if the else
statement is not on the same line of the brace defining the end of the if
statement, since R will assume that the code was complete with the first statement.
Consider the following simple example:
> x <- 1 > if(x %% 2 == 0) print("x is even") else print("x is odd") [1] "x is odd" > x <- 2 > if(x %% 2 == 0) print("x is even") else print("x is odd") [1] "x is even"
In this simple example, we assigned a value to a variable and then we checked if the value is even or odd. This is done using the modulus operator %%
. So if the modulus is 0
, the code will print on the console the message "x is even"
, otherwise it will print "x is odd"
. You will also notice the use of the operator ==
(equal to), and how the braces can be omitted with statements containing only one command.
In R, there is also an alternative (more concise) option available for the if…else
statement, the ifelse()
function. This function has the general form ifelse(test, yes, no)
, where test
is the logical expression that is evaluated as yes
and is executed if test
is TRUE
, and as no
if otherwise. The previous example would look like the following if coded using this alternative function:
> x<-1 > ifelse(x%%2==0, print("x is even"), print("x is odd"))
The for
loop is one of the methods that can be used to repeat a certain portion of code. The underlying idea is that you request that an index ,i
, takes on a sequence of values, and that one or more lines of commands are executed many times as there are different values of i
. An important aspect of such looping is that the variable i
will take a different value at each loop, and that value is usually used in the code. The general syntax of the for
loop is the following, where i
is a simple variable and myVector
is a vector:
for (i in myVector) { expression.1 ... }
When executed, the for
command executes the group of expressions within the braces { }
, once for each element of the vector. The variable i
will take the value of each element of the vector myVector
. You can find a very simple example of a for
loop in the following code, in which the for
construct is used to print the square root of each element of a vector:
> for (i in c(3,4,9,5)) print(sqrt(i)) [1] 1.732051 [1] 2 [1] 3 [1] 2.236068
As a simple application, consider an improvement of one of the previous examples; a code that will print an even-odd message but will test each element of a vector instead of an individual variable. You can do that by combining a for
loop and an if..else
statement as shown in the following code:
> x <- c(2,5,1,6) > for (i in x){ + if(i %% 2 == 0){ + print("x is even") + } else{ print("x is odd") + } + } [1] "x is even" [1] "x is odd" [1] "x is odd" [1] "x is even"
In this example, the variable i
takes the value of one of the elements of the vector at each run of the loop, which is then tested for an even-odd message using the if..else
construct.
In some cases, you may need to generate a loop accessing the position of each element in a vector, instead of the element itself. You may see the previous example in the following code snippet, but this time the i
variable in the for
loop will not take the value of the elements of the vector x
, but the position of each element within the vector:
> x <- c(2,5,1,6) > for (i in 1:length(x)){ + vecElelement <- x[i] + if(vecElelement %% 2 == 0){ + print("x is even") + } else{ print("x is odd") + } + } [1] "x is even" [1] "x is odd" [1] "x is odd" [1] "x is even"
In the previous example, the for
loop defines a vector with numeric values going from 1
up to the length of the vector x
. In this way, the loop can locate each element of the vector and assign it to a variable, vecElement
, which then will be simply tested for odd-even messages using the if...else
construct.
In some situations, we do not know beforehand how many times we will need to go around a loop, so each time we go around the loop, we will have to check some condition to see if we are done yet. In these situations, we use a while
loop, which has the following general syntax:
while (logical.expression) { expression.1 ... }
When while
is executed, the value of the value of logical.expression
is evaluated first. If it is TRUE
then the group of expressions in braces { }
is executed. After that, the execution comes back to the beginning of the loop; if logical.expression
is still TRUE
, the grouped expressions are executed again, and so on. Clearly, for the loop to stop, the value of logical.expression
must eventually become FALSE
. Achieving logical.expression
usually depends on a variable that is altered within the grouped expressions. Remember that the key point is if you want to use while
for avoiding infinite loops, it is advised to set up an indicator variable and change its value within each iteration. The while
loop is more fundamental than the for
loop, as we can always rewrite a for
loop as a while
loop. Following is a simple example of a while
loop that will print on the console the numbers from 1
to 10
. You can notice how the variable x
is increased at each run of the loop.
> x<-1 > while(x<10){ + print(x) + x <- x+1 # Counter which will increase at each run of the loop + } [1] 1 [1] 2 [1] 3 [1] 4 [1] 5 [1] 6 [1] 7 [1] 8 [1] 9
A slightly more complex example of the while
loop is the generation of the Fibonacci series. In this series, each number is equal to the sum of the two preceding numbers, so you will have 1,1,2,3,5,8, and so on. In the following example, we will define a variable, n
, representing the number of elements of the Fibonacci series that we intend to obtain, and two variables, a
and b
, which will be used to start the generation of the series:
> a<-1 > b<-0 > n<-10 > while(n>0){ + c <- a + a <- a+b + b <- c + n<-n-1 + print(b) + } [1] 1 [1] 1 [1] 2 [1] 3 [1] 5 [1] 8 [1] 13 [1] 21 [1] 34 [1] 55
Within the loop, we decrease the value of n
by 1
at each run, bringing the loop to an end as soon as we have n
elements of the series. In the loop we also need to define a new variable, c
, the only function of which is to avoid losing the value of the variable a
. In fact, when we replace a
by a+b
on line six, we lose the original value of a
. If we had not stored this value in c
, we could not have set the new value of b
to the old value of a
on line seven.
At this point you have already noticed that many things in R are done using function calls. Functions in R are objects that carry out operations on arguments that are supplied to them and return one or more values. The general syntax for writing a function is:
function(argument list) body
The first component of the function declaration is the keyword function()
, which indicates to R that you want to create a function. An argument list is a comma-separated list of formal arguments. A formal argument can be a symbol (that is, a variable name such as x
or y
), an assignment statement of the form symbol=expression
(for example, mean=2
), or the special formal argument ...
(triple dot). The body can be any valid R expression or a set of R expressions. Generally, the body is a group of expressions contained in curly brackets { }
, with each expression on a separate line. As soon as you have defined the function in your workspace, you can use it by writing a call to it. As already mentioned before, R assumes the positional matching for the arguments, so if the argument is not clearly defined in the function call, R will assume that the arguments provided will have the same order as the default arguments in the function declaration. All these details will become clearer after a couple of examples.
First, let's assume that we want to define a function that takes a vector as an argument and returns the greater element of the vector. Clearly such a function is already available in R, that is, max()
, but for the purpose of this exercise, let's code our own function!
Following is a possible coding:
> max.value1 <- function(myVec){ + myVec <- sort(myVec, decreasing = TRUE) + myVec[1] + } > > max.value1(c(1,2,3,4)) [1] 4
We have defined a function called max.value1()
, which takes only one argument, myVec
. This vector is then sorted in the descending order using a call to the function sort()
, and the first element, which will be the greatest element, will be provided by the function.
Now let's assume an improvement of such a function. Consider that we would like the function to not only provide the maximum value within a vector, but also check if the value appears multiple times and, if this is the case, print a message telling the number of times for which this value appeared in the vector, otherwise print only the maximum value. Clearly we will need to include an if…else
statement within the function.
Following is an example of the possible code:
> max.value2 <- function(myVec){ + myVec <- sort(myVec, decreasing = TRUE) + myMax <- myVec[1] + n <- length(myVec[myVec==myMax]) + if(n>1) print(paste("The max value + is",myMax,"and it appears in the + vector",n,"times")) + else print(paste("The max value is",myMax)) + } > max.value2(c(1,2,3,4,5)) [1] "The max value is 5" > max.value2(c(1,2,3,4,5,5)) [1] "The max value is 5 and it appears in the vector 2 times"
In the previous example, the function simply counts the number of times the maximum value appears in the vector and how it defines the variable n
(line 4). Then it checks if this value is greater than 1
and prints on the screen the relative message. Since we need to include actual text together with the values of other variables such as myMax
and n
in the message to print, we can use the function paste()
, which allows us to combine strings delimited by quotes and values of variables. In this case the use of the paste()
function is not necessary, but in some other situation this function may turn out to be really useful.
Some applications are much more straightforward if the number of arguments is not required to be specified in advance. There is a special formal name ...
(triple dot), which is used in the argument list to specify that an arbitrary number of arguments will be passed to the function. As a simple example, consider the function max.value1()
. In this function we assume that the user should provide a vector as an argument. Let's assume that we would like to build a function that would get any number of values and would provide the maximum number. In the following case, you will not know many of the arguments that will be provided by the user, so you can use ...
as an argument:
> max.value3 <- function(...){ + myVec <- c(...) + myVec <- sort(myVec, decreasing = TRUE) + myVec[1] + } > max.value3(2,3,4,5,6) [1] 6
As you can see, in the previous case, we do not define the vector in the call to the function, but the vector is defined within the body of the function itself. This flexible call is particularly useful when you use other functions within the one you are creating.
You can see an example of a function returning a vector in the following code snippet. This function was built on the Fibonacci example shown previously, but in this case the code is included within a function. This function takes a number, n
, as an argument, and returns a vector containing the first n
elements of the Fibonacci series:
> fibonacci <- function(n=10){ + a<-1 + b<-0 + fib<-NULL + while(n>0){ + c <- a + a <- a+b + b <- c + n<-n-1 + fib <- c(fib,b) + } + return(fib) + } > fibonacci(n=12) [1] 1 1 2 3 5 8 13 21 34 55 89 144 > fibonacci() [1] 1 1 2 3 5 8 13 21 34 55
You can see how in this case, we also specified a default value for the function fibonacci()
. This means that a call to the function without any specified argument (the last two lines of code) will generate a vector containing the first 10 elements of the series. In this case, we create during the execution of the function a vector, fib
, in which n
elements of the Fibonacci series are added one after the other. Then, simply using the command return()
, the function will return the vector.
When you have a function providing any kind of result, you can also assign this result to a variable, for instance; in this way you will be able to use the results of the function on additional calculations. You can see an example of using the output of the function fibonacci()
as an input to the function max.value3()
in the following snippet:
> x <- fibonacci(12) # creation of a Fibonacci vector > > x [1] 1 1 2 3 5 8 13 21 34 55 89 144 > > max.value3(x) # finding the max value of the vector [1] 144
Like any other programming language, R will occasionally produce error messages that are not easily understandable. For this reason, several tools are available in order to perform debugging of new functions.
You will spend a lot of time correcting errors in your programs. In order to find an error or a bug, you need to be able to see how your variables change as you move through the branches and loops of your code so that you can safely monitor what your code does. An effective and simple way of doing this is to include statements such as cat("var =", var, "\n")
throughout the program, to display the values of variables such as var
while the program executes. Once you have the program working, you can delete these statements or just make them a comment so that they are not executed. For example, taking the example of the fibonacci()
function reported previously, you could require the function to print at each while
loop the value of the vector fib
and check that the function is working properly:
> fibonacci <- function(n=10){ + a<-1 + b<-0 + fib<-NULL + while(n>0){ + c <- a + a <- a+b + b <- c + n<-n-1 + fib <- c(fib,b) + cat("n=",n,"\t","fib=",fib,"\n") + } + return(fib) + } > > fibonacci(12) n= 11 fib= 1 n= 10 fib= 1 1 n= 9 fib= 1 1 2 n= 8 fib= 1 1 2 3 n= 7 fib= 1 1 2 3 5 n= 6 fib= 1 1 2 3 5 8 n= 5 fib= 1 1 2 3 5 8 13 n= 4 fib= 1 1 2 3 5 8 13 21 n= 3 fib= 1 1 2 3 5 8 13 21 34 n= 2 fib= 1 1 2 3 5 8 13 21 34 55 n= 1 fib= 1 1 2 3 5 8 13 21 34 55 89 n= 0 fib= 1 1 2 3 5 8 13 21 34 55 89 144 [1] 1 1 2 3 5 8 13 21 34 55 89 144
As you have seen, using the cat()
command, the function was able to print on the screen the value of the vector fib
and the number for which the while
loop was executed. Let's analyze this line of code in more detail. You can see the same line divided in portions, with some explanation for each component, in the following code snippet:
cat("n=", # Print the character "n=" on screen n, # Print the value of n "\t", # Print a tabular space on the same line "fib=", # Print the character "fib=" fib, # Print the value of the variable fib "\n") # Move to a new line
When debugging a function or a code in general, always try to use values for which you know the results, so that you can easily test if the code is working fine. Always start by writing code with a lower complexity, apply some tests, and only when you are quite sure there are no bugs, increase the complexity. Remember that the more complex the code, the more difficult it will be for finding bugs. Try to indent your code as well, so that it will be easier for you (and everybody else who will look at your code) to understand where each portion of code starts and ends; this is particularly important for nested loops.
There are several additional functions available in R for a more detailed analysis of your code and to perform debugging; following is the list of the main functions:
traceback()
: This function will print the sequence of calls that lead to an error. You need to call this function without arguments after you have obtained the error from the function you want to debug. The following example includes the functionlm()
, which calculates a linear regression between two arguments,x
andy
. The error is generated because we do not definex
andy
. The function traces back from the beginning of the function to the point where the error was produced:> lm(x~y) Error in eval(expr, envir, enclos) : object 'x' not found > traceback() 7: eval(expr, envir, enclos) 6: eval(predvars, data, env) 5: model.frame.default(formula = x ~ y, drop.unused.levels = TRUE) 4: model.frame(formula = x ~ y, drop.unused.levels = TRUE) 3: eval(expr, envir, enclos) 2: eval(mf, parent.frame()) 1: lm(x ~ y)
debug()
: This function allows you to interact with R on a step-by-step basis. This function accepts the name of the function to debug as an argument, and this function is then flagged for debugging. To unflag the function, you can pass the name of the function toundebug()
. When you pass a call to thedebug()
function, the body of the function will be printed on the screen and then each statement in the function gets executed one at a time. You can control when each statement gets executed by pressing Enter.An example of the use of this function is the following code. The output of the function is not reported because of its size:
debug(lm) lm(x~y) undebug(lm)
browser()
: This function suspends the execution of a function wherever it is called and puts the function in the debug mode. If you place a call tobrowser
() inside your function, the execution will pause allowing you to go line-by-line from there.
Exception handling features help you deal with any unexpected or exceptional situations that can occur when a program is running. Such expressions are usually introduced within the body of the function.
After you have defined an initial version of your function, during the debugging and testing phases, you will probably discover that a special input to the function can lead to a malfunction, so you can use a special expression to inform the user that something exceptional happened or to stop the function from working. The main functions that will allow you to do that are warning()
, stop()
, and try()
(or its general version tryCatch()
). You can find a brief description of these functions in the following list, but for more detailed information on their use, you can have a look at their help
file in R:
warning()
: This function will generate a warning message on the console containing its argument. The appearance of a warning does not stop the execution of the function. In some cases, it may be helpful to suppress the warnings produced by a function; for instance, if the function is working fine for you, usesuppressWarnings()
as an argument in the expression that produces the warning.stop()
: This function will stop the execution of the current expression and print an error message on the console. The printed message is defined in the call to thestop()
function.try()
: This is a wrapper function that "tries" the execution of an expression. It contains a logical argument,silent
, which you can use to choose if the error messages should be suppressed. The functiontry()
is a simplified version of the more general functiontryCatch()
.
One of the most important aspects in the presentation of data is the production of quality plots. R has several options that allow you to produce plots in a standard format and also gives a deep control of the plot appearance. There are three different main packages available for data representation:
graphics
: This is the basic package already available in the R environment and is already loaded by default.lattice
: This package is already available with the basic installation of R, but you will have to load it in the workspace using thelibrary()
function.ggplot2
: This is probably the more recent package for data plotting. It is not included in the R basic installation, so you will need to install it using the CRAN mirrors, for instance, using the commandinstall.packages("ggplot2")
.
The difference between these packages is not only related to the aesthetic aspect of the plots generated, but also on the underlying philosophy behind the plot definition. Because of such substantial difference, the code used in one package is different from the others. In this case, we will consider some simple examples with the basic package graphics
, but as soon as you become familiar with R, you should definitely start using the others as well if you want to get the maximum in data visualization.
If you want to investigate the relationship between two variables and produce a typical x versus y plot, you can do that with the plot()
function. As an example, we can use the dataset Orange
and produce a plot of the age
versus circumference
values for the orange trees:
> plot(Orange$age,Orange$circumference)
Other basic plots available are, for instance, histograms, with the function histogram()
, the bar plot, with the function barplot()
, and pie charts, with the function piegraph()
.
The previous code will lead to the output as shown in the following image. Within the plot()
function, you can specify other optional arguments in order to modify the basic plot and change its look and/or add information to the figure. The following is a list of the main functions:
main
: This function will specify the title of the plot.xlab
andylab
: These functions will specify the x and y axes' labels.pch
: This function will give a numeric argument defining the plotting symbol.lwd
: This function will give the thickness of lines.type
: This function will give the plotting type (dots, lines, mix, and so on).col
: This function will select the color of the plot. You can have a list of the colors available using the codecolors()
.
On top of the main plot, which is defined by a high-level function (plot()
in this case), you may add additional components using low-level functions, such as points
for points or lines
for lines. The following screenshot shows a simple x-y plot with the dataset Orange
:
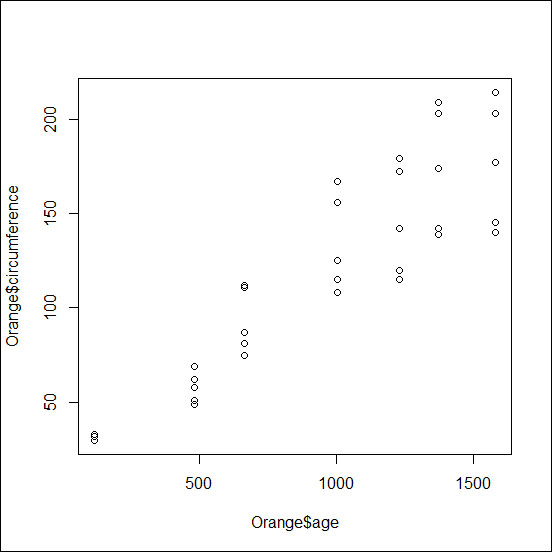
The following is an example of how to specify these options in plot
:.
plot(Orange$age,Orange$circumference, main="Plot of Orange dataset", xlab="Age", ylab="Circumference", type="p", pch=2, col="red") lines(loess.smooth(Orange$age,Orange$circumference), col="blue", lwd=2)
The output of this plot is reported in the following screenshot:
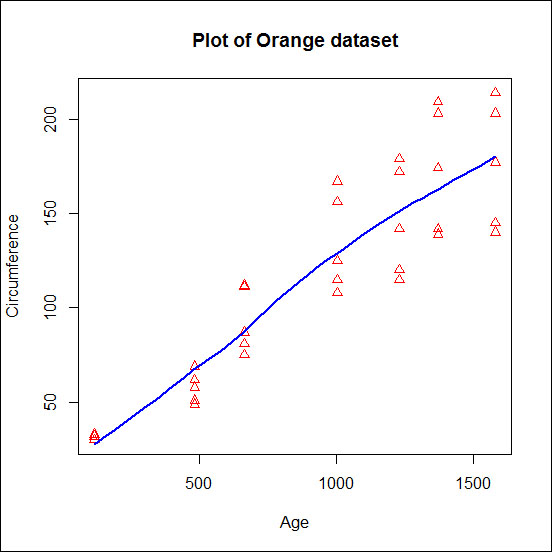
In the previous example, we used the plot()
function to generate the first plot containing the individual observation, and then we used the lines()
function to add a line representing the tendency of the data. The function loess.smooth()
computes the point of a smooth curve, a curve describing the tendency of the data, and these points are then passed to the function lines
that draws them in the plot. You can have an idea of the output produced by the loess.smooth()
function by running the following code:
> loess.smooth(Orange$age,Orange$circumference)
If you need help with R, the following section gives you information about some people and places that will prove invaluable.
The following are the official sites:
Website of the R project and CRAN: http://www.r-project.org/
RStudio IDE: http://www.rstudio.com/ide/
Eclipse IDE: http://www.eclipse.org/
Emacs Speaks Statistics: http://ess.r-project.org/
Manual and documentation: http://www.r-project.org/
The following are some useful websites with articles and tutorials on the most important topics:
Graph Gallery: http://gallery.r-enthusiasts.com/
R Journal: http://journal.r-project.org/
Cookbook for R: http://www.cookbook-r.com/
The following are some community sites:
Official mailing list: http://www.r-project.org/mail.html
LinkedIn R group: http://www.linkedin.com/groups/R-Project-Statistical-Computing-77616?trk=myg_ugrp_ovr
Unofficial forums: http://stackoverflow.com/
For more Open Source information, follow Packt at http://twitter.com/#!/packtopensource.