Process
Chapter 2, Good Data Science, mentioned the requirement for governance in data science to ensure the outcomes of projects are sound. The process of creating value from data follows an iterative workflow that works from raw data to a finished project. (Wickham, H., & Grolemund, G. (2016). R for data science: Import, Tidy, Transform, Visualize, and Model Data Sebastopol, CA: O'Reilly. Available at—https://r4ds.had.co.nz/). The workflow starts with defining a problem that needs solving as shown in Figure 4.2. The next step involves loading and transforming the data into a format that is suitable for the required analysis. The data science workflow contains a loop that consists of exploration, modelling, and reflection, which is repeated until the problem is solved or is shown to be unsolvable.
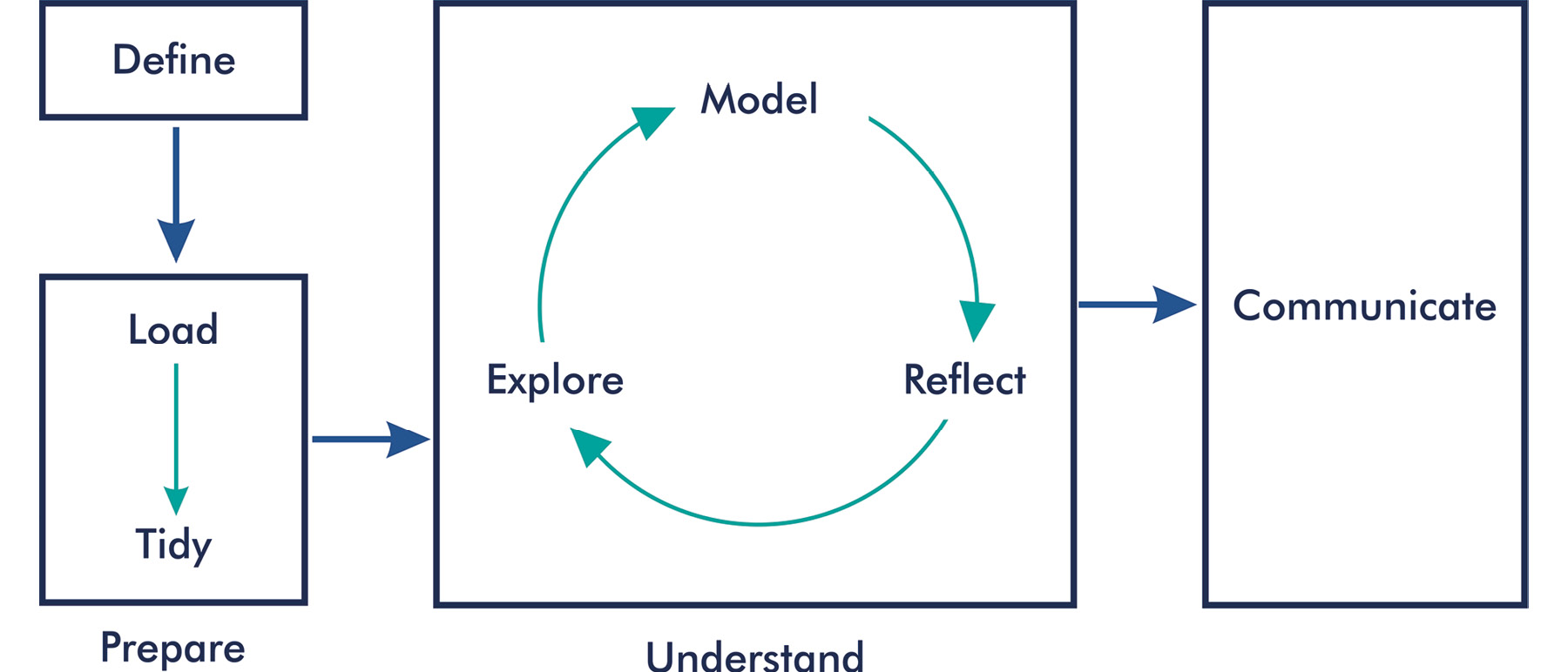
Figure 4.2: Data science workflow
The workflow for a data project is independent of the aspect of the data science continuum under consideration. The same principles...