In this chapter, you will learn the basics of ML pipelines and how they can be used in a variety of contexts. The pipeline is made up of several components. ML pipelines leverage the Spark platform and machine learning to provide key features for making the construction of large-scale learning pipelines simple.
You're reading from Machine Learning with Spark. - Second Edition
Introduction to pipelines
The pipeline API was introduced in Spark 1.2 and is inspired by scikit-learn. The concept of pipelines is to facilitate the creation, tuning, and inspection of ML workflows.
ML pipelines provide a set of high-level APIs built on top of DataFrames that help users create and tune practical machine learning pipelines. Multiple algorithms from Spark machine learning can be combined into a single pipeline.
An ML pipeline normally involves a sequence of data pre-processing, feature extraction, model fitting, and validation stages.
Let's take an example of text classification, where documents go through preprocessing stages, such as tokenization, segmentation and cleaning, extraction of feature vectors, and training a classification model with cross-validation. Many steps involving pre-processing and algorithms can be tied together using the pipeline. The pipeline typically sits above the...
How pipelines work
We run a sequence of algorithms to process and learn from a given dataset. For example, in text classification, we split each document into words and convert the words into a numerical feature vector. Finally, we learn a predictive model using this feature vector and labels.
Spark ML represents such a workflow as a pipeline, which consists of a sequence of PipelineStages (transformers and estimators) to be run in a particular order.
Each stage in PipelineStages is one of the components, either a transformer or an estimator. The stages are run in a particular order while the input DataFrame flows through the stages.
In the following figure, the dpText document pipeline demonstrates the document workflow where Tokenizer, Hashing, and Logistic Regression are the components of the pipeline. The Pipeline...
Machine learning pipeline with an example
As discussed in the previous sections, one of the biggest features in the new ML library is the introduction of the pipeline. Pipelines provide a high-level abstraction of the machine learning flow and greatly simplify the complete workflow.
We will demonstrate the process of creating a pipeline in Spark using the StumbleUpon dataset.
Download the training data (train.tsv)--you will need to accept the terms and conditions before downloading the dataset. You can find more information about the competition at http://www.kaggle.com/c/stumbleupon.
Here is a glimpse of the StumbleUpon dataset stored as a temporary table using Spark SQLContext:
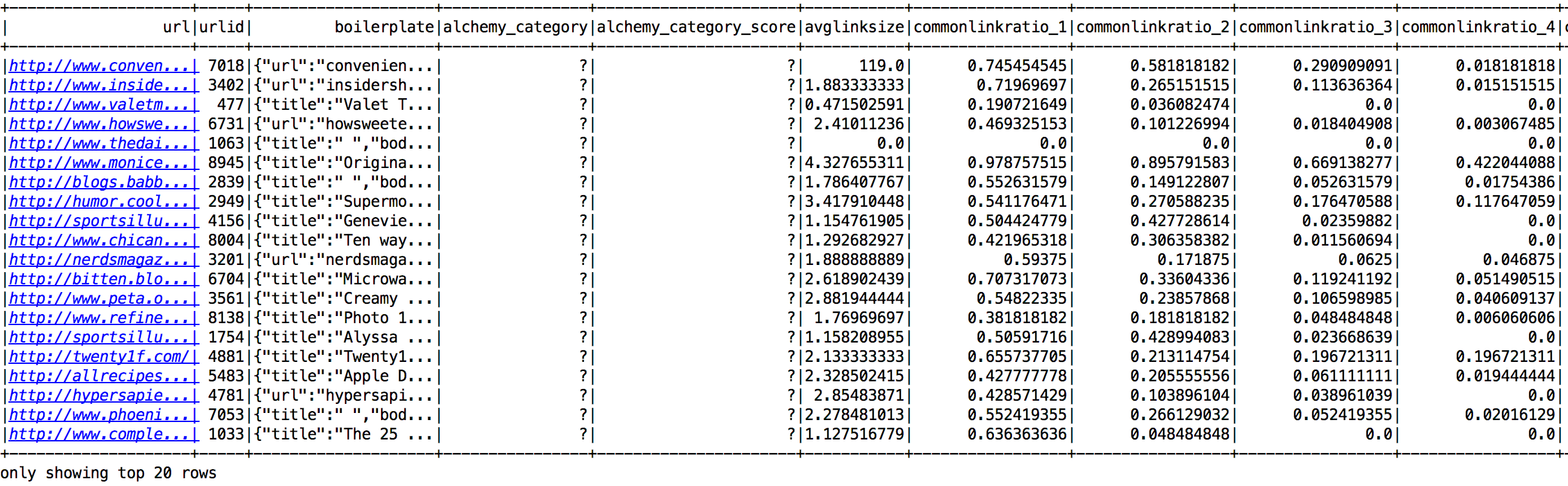
Here is a visualization of the StumbleUpon dataset:
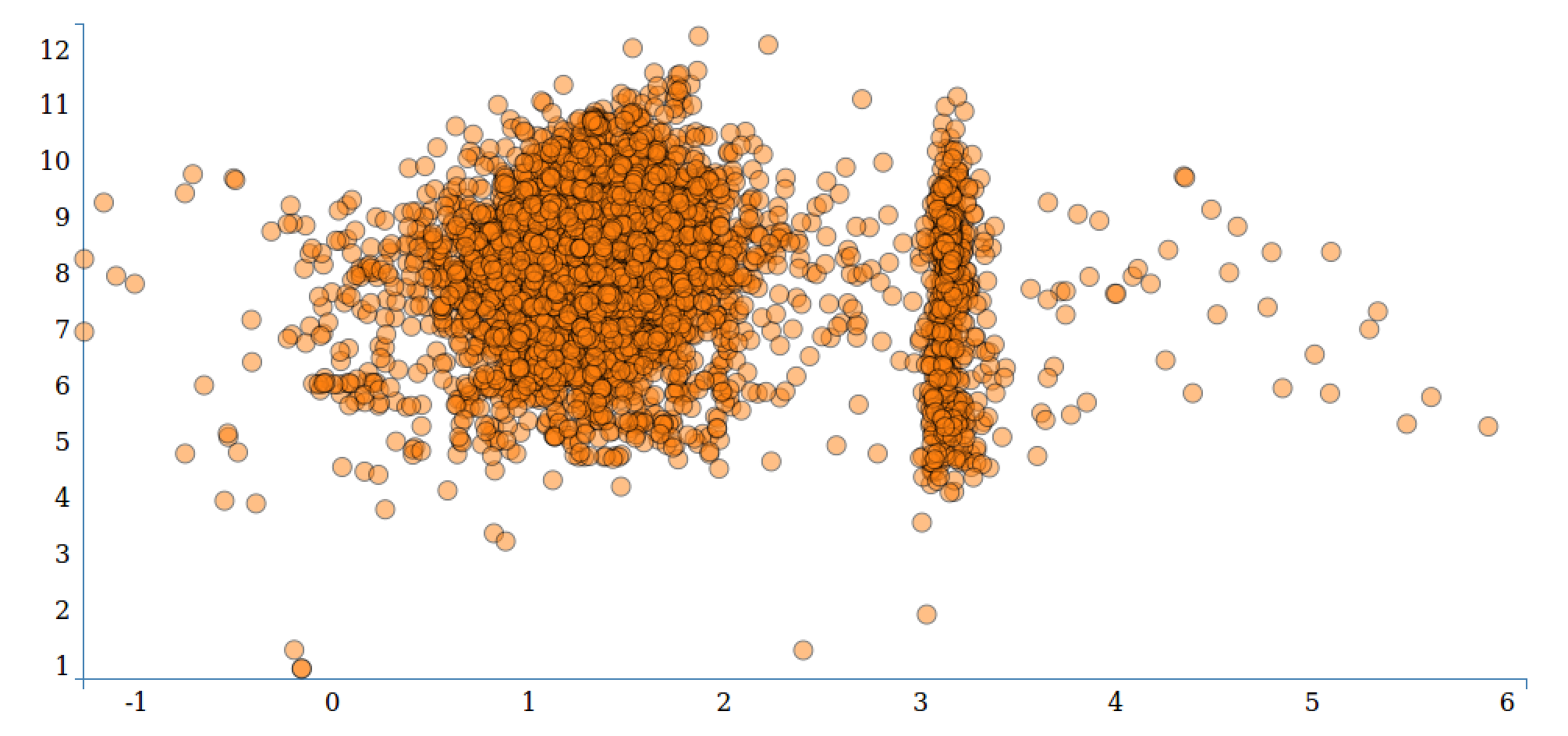
Summary
In this chapter, we covered the basics of Spark ML Pipeline and its components. We saw how to train models on input DataFrame and how to evaluate their performance using standard metrics and measures while running them through spark ML pipeline APIs. We explored how to apply some of the techniques like transformers and estimators. Finally, we investigated the pipeline API by applying different algorithms on the StumbleUpon dataset from Kaggle.
Machine Learning is the rising star in the industry. It has certainly addressed many business problems and use cases. We hope that our readers will find new and innovative ways to make these approaches more powerful and extend the journey to understand the principles that hold learning and intelligence. For further practice and reading on Machine Learning and Spark refer https://www.kaggle.com and https://databricks.com/spark/ respectively.