Machine learning in the healthcare domain is booming because of its ability to provide accurate and stable techniques. Machine learning algorithms provide strategies to deal with a variety of structured, unstructured, and semi-structured data. This book is packed with new approaches and methodologies to create powerful solutions for healthcare analytics.
This book will implement key machine learning algorithms and their use cases using a range of libraries from the Python ecosystem. We will build five end-to-end projects within the organization to evaluate the efficiency of artificial intelligence applications when carrying out simple and complex healthcare analytics tasks. Each project will help you to delve deep into newer and better ways to manage insights and handle healthcare data efficiently. We will use machine learning to detect cancer in a set of patients using the SVM and KNN models. Apart from that, we will create a deep neural network in Keras to predict the onset of diabetes on a huge dataset of patients. We will also learn how to predict heart diseases using neural networks.
By the end of this book, you will learn how to address long-standing challenges, provide specialized solutions to deal with them, and carry out a range of cognitive tasks in the healthcare domain.
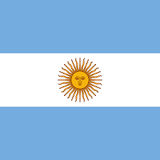
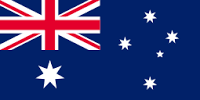
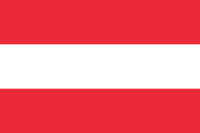
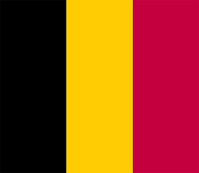
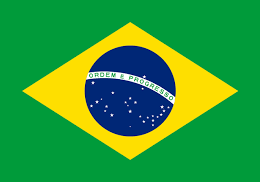
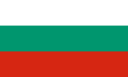
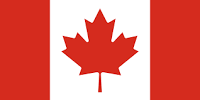
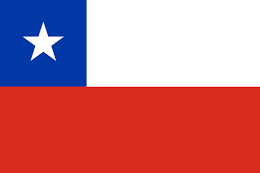
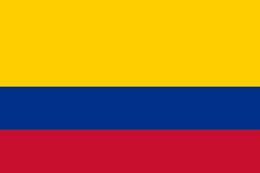
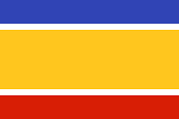
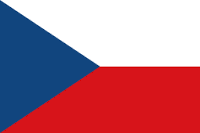
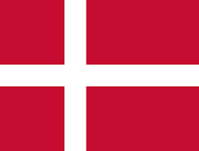
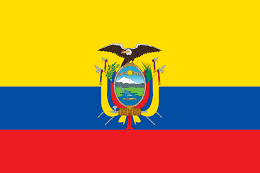
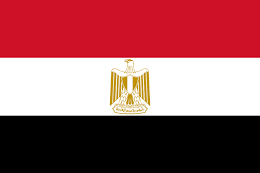
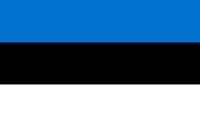
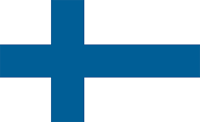
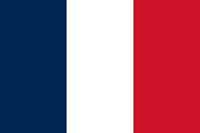
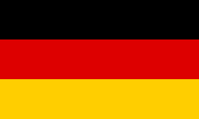
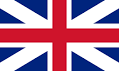
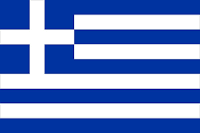
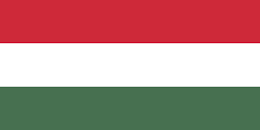
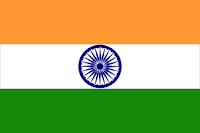
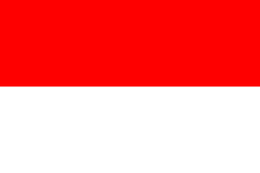
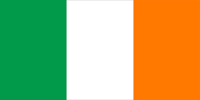
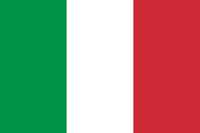
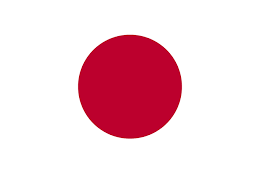
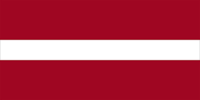
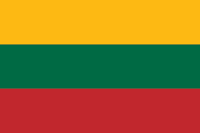
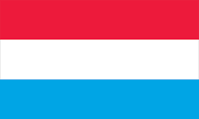
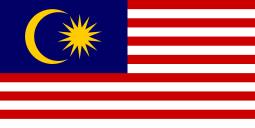
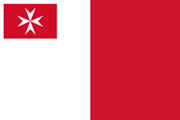
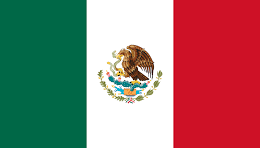
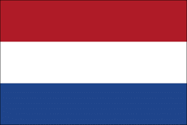
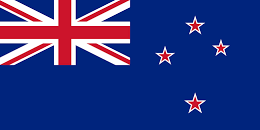
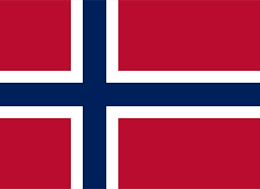
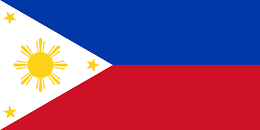
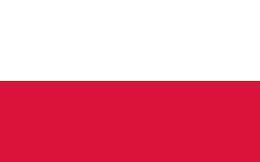
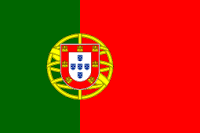
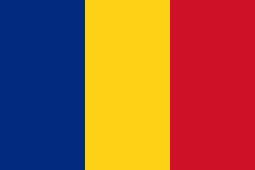
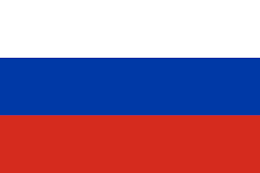

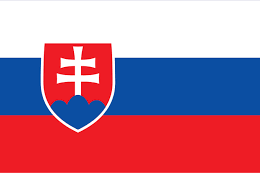
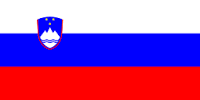
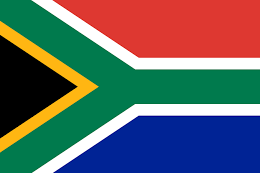
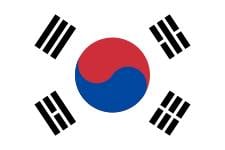
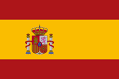
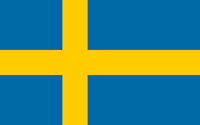
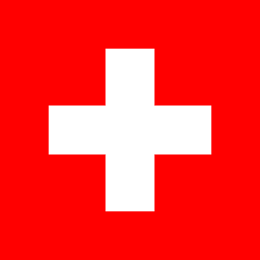
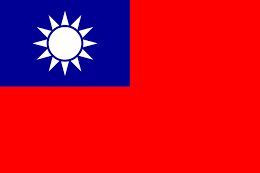
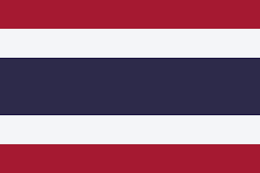
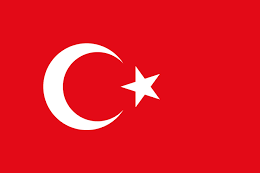
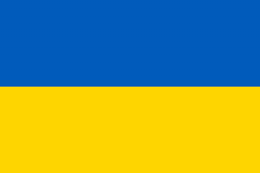
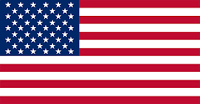