In this chapter, you learned about two deep learning algorithms that don't require pre-training: deep neural networks with dropout and CNN. The key to high precision rates is how we make the network sparse, and dropout is one technique to achieve this. Another technique is the rectifier, the activation function that can solve the problem of saturation that occurred in the sigmoid function and the hyperbolic tangent. CNN is the most popular algorithm for image recognition and has two features: convolution and max-pooling. Both of these attribute the model to acquire translation invariance. If you are interested in how dropout, rectifier, and other activation functions contribute to the performance of neural networks, the following could be good references: Deep Sparse Rectifier Neural Networks (Glorot, et. al. 2011, http://www.jmlr.org/proceedings/papers/v15/glorot11a/glorot11a.pdf), ImageNet Classification with Deep Convolutional Neural Networks (Krizhevsky et. al. 2012, https:/...
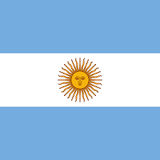
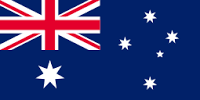
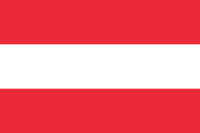
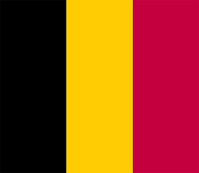
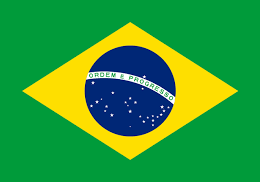
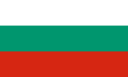
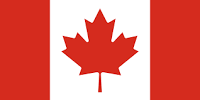
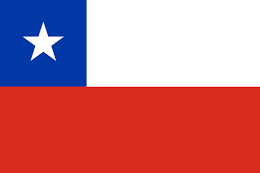
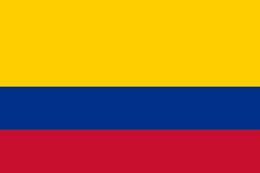
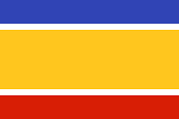
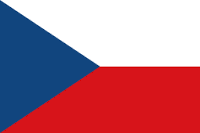
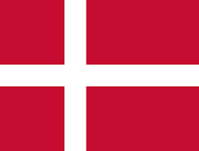
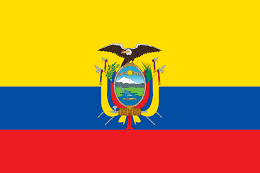
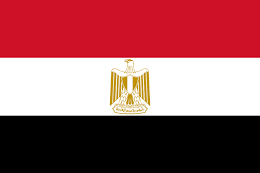
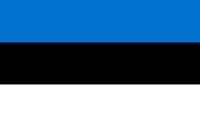
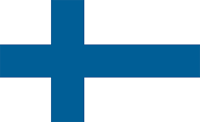
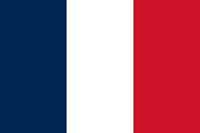
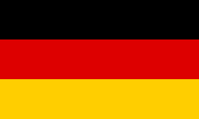
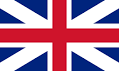
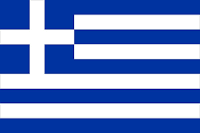
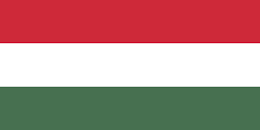
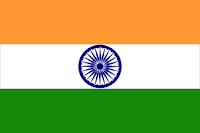
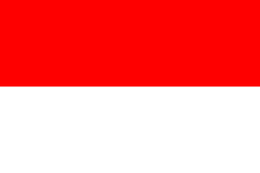
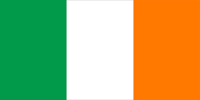
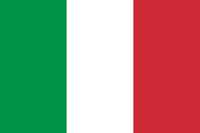
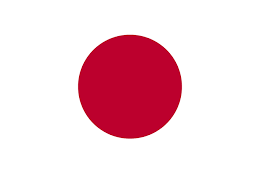
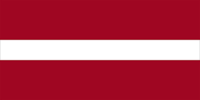
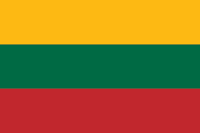
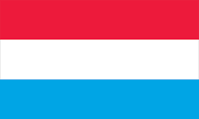
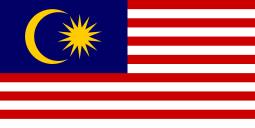
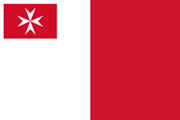
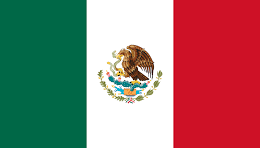
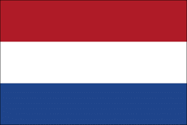
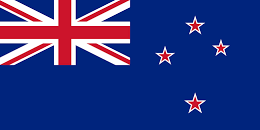
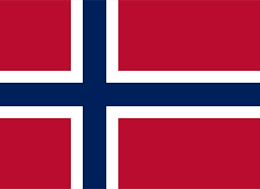
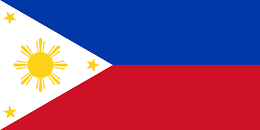
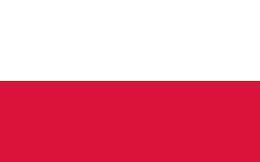
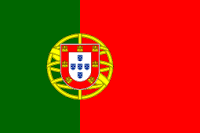
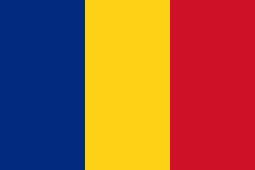
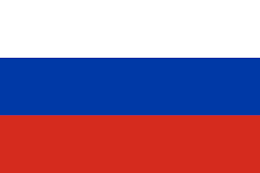

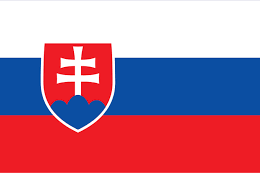
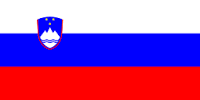
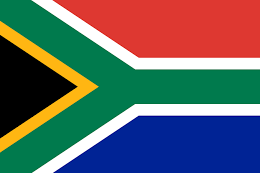
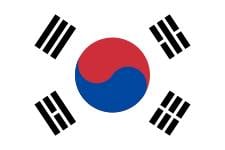
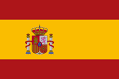
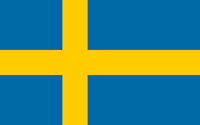
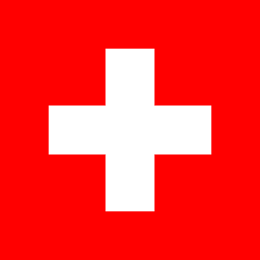
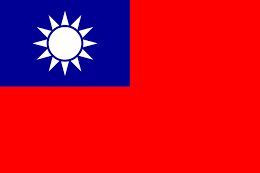
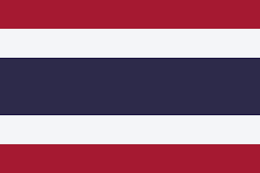
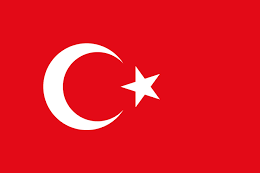
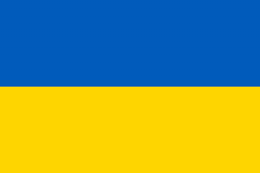
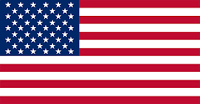