Detecting and classifying objects in images is a challenging problem. So far, we have treated the issue of image classification on a simple level; in a real-life scenario, we are unlikely to have pictures containing just one object. In industrial environments, it is possible to set up cameras and mechanical supports to capture images of single objects. However, even in constrained environments, such as an industrial one, it is not always possible to have such a strict setup. Smartphone applications, automated guided vehicles, and, more generally, any real-life application that uses images captured in a non-controlled environment require the simultaneous localization and classification of several objects in the input images. Object detection is the process of localizing an object into an image by predicting the coordinates of a bounding box that...
You're reading from Hands-On Neural Networks with TensorFlow 2.0
Getting the data
Object detection is a supervised learning problem that requires a considerable amount of data to reach good performance. The process of carefully annotating images by drawing bounding boxes around the objects and assigning them the correct labels is a time-consuming process that requires several hours of repetitive work.
Fortunately, there are already several datasets for object detection that are ready to use. The most famous is the ImageNet dataset, immediately followed by the PASCAL VOC 2007 dataset. To be able to use ImageNet, dedicated hardware is required since its size and number of labeled objects per image makes the object detection task hard to tackle.
PASCAL VOC 2007, instead, consists of only 9,963 images in total, each of them with a different number of labeled objects belonging to the 20 selected object classes. The twenty object classes are as follows...
Object localization
Convolutional neural networks (CNNs) are extremely flexible objects—so far, we have used them to solve classification problems, making them learn to extract features specific to the task. As shown in Chapter 6, Image Classification Using TensorFlow Hub, the standard architecture of CNNs designed to classify images is made of two parts—the feature extractor, which produces a feature vector, and a set of fully connected layers that classifies the feature vector in the (hopefully) correct class:
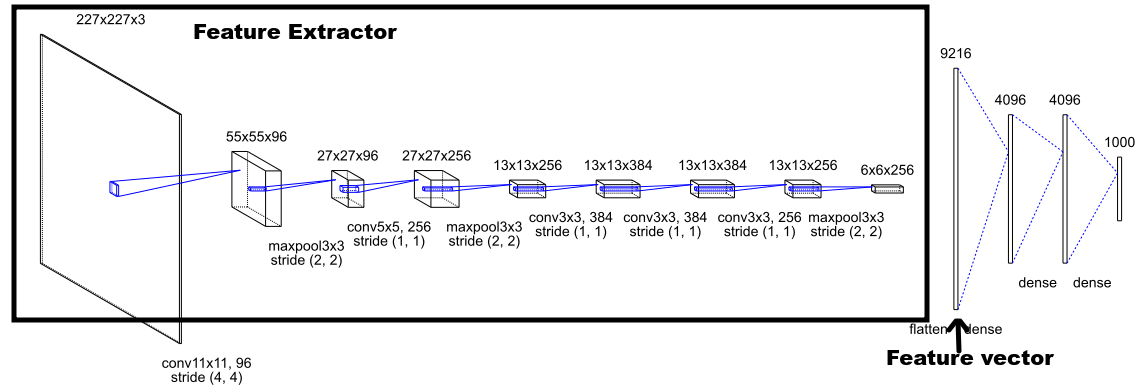
The fact that, so far, CNNs have only been used to solve classification problems should not mislead us. These types of networks are extremely powerful, and, especially in their multilayer setting, they can be used to solve many different kinds of problems, extracting...
Classification and localization
An architecture like the one defined so far that has no information about the class of the object it's localizing is called a region proposal.
It is possible to perform object detection and localization using a single neural network. In fact, there is nothing stopping us adding a second head on top of the feature extractor and training it to classify the image and at the same time training the regression head to regress the bounding box coordinates.
Solving multiple tasks at the same time is the goal of multitask learning.
Multitask learning
Rich Caruna defines multi-task learning in his paper Multi-task learning (1997):
Summary
In this chapter, the problem of object detection was introduced and some basic solutions were proposed. We first focused on the data required and used TensorFlow datasets to get the PASCAL VOC 2007 dataset ready to use in a few lines of code. Then, the problem of using a neural network to regress the coordinate of a bounding box was looked at, showing how a convolutional neural network can be easily used to produce the four coordinates of a bounding box, starting from the image representation. In this way, we build a region proposal, that is, a network able to suggest where in the input image a single object can be detected, without producing other information about the detected object.
After that, the concept of multi-task learning was introduced and how to add a classification head next to the regression head was shown by using the Keras functional API. Then, we covered...
Exercises
You can answer all the theoretical questions and, perhaps more importantly, struggle to solve all the code challenges that each exercise contains:
- In the Getting the data section, a filtering function was applied to the PASCAL VOC 2007 dataset to select only the images with a single object inside. The filtering process, however, doesn't take into account the class balancement.
Create a function that, given the three filtered datasets, merges them first and then creates three balanced splits (with a tolerable class imbalance, if it is not possible to have them perfectly balanced). - Use the splits created in the previous point to retrain the network for localization and classification defined in the chapter. How and why do the performances change?
- What measures the Intersection over Union metric?
- What does an IoU value of 0.4 represent? A good or a bad match?
- What...