In this chapter, we will continue with 2D convolution and understand how convolution can be done faster in the frequency domain (with basic concepts of the convolution theorem). We will see the basic differences between correlation and convolution with an example on an image. We will also describe an example from SciPy that will show how to find the location of specific patterns in an image with a template image using cross-correlation. Finally, we will describe a few filtering techniques (that can be implemented with convolution using kernels, such as box-kernel or Gaussian kernel) in the frequency domain, such as high-pass, low-pass, band-pass, and band-stop filters, and how to implement them with Python libraries by using examples. We shall demonstrate with examples how some filters can be used for image denoising (for example, the band-reject
or notch
filters to remove periodic noise from an image, or the inverse or Wiener filters...
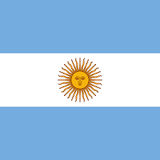
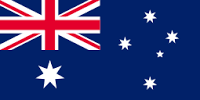
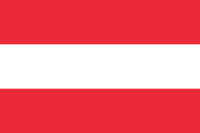
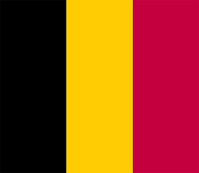
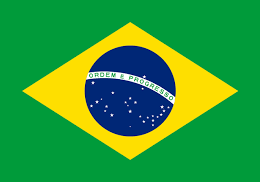
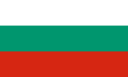
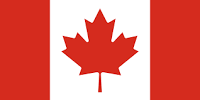
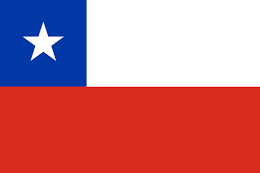
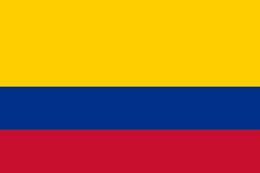
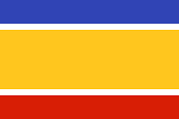
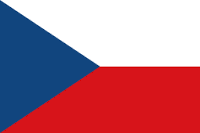
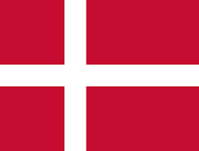
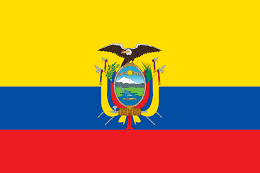
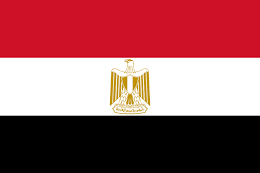
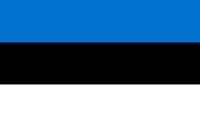
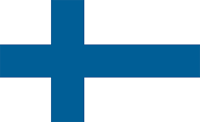
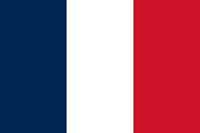
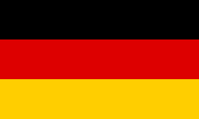
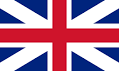
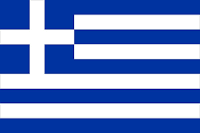
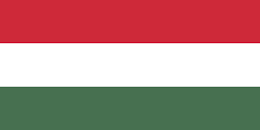
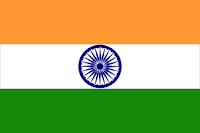
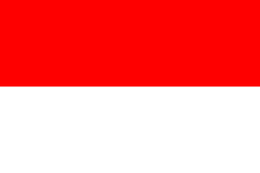
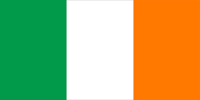
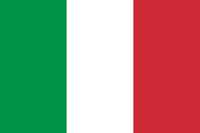
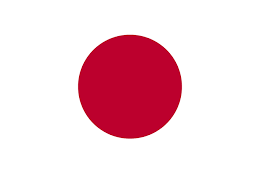
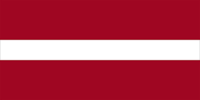
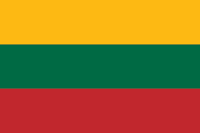
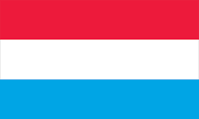
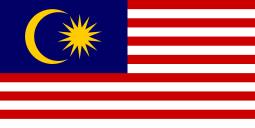
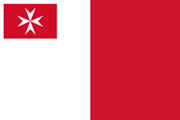
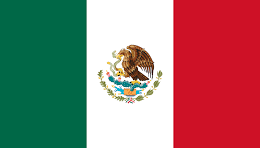
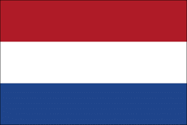
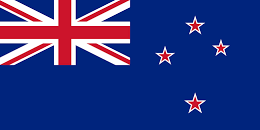
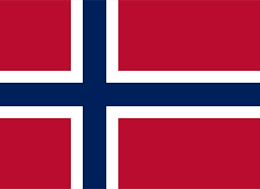
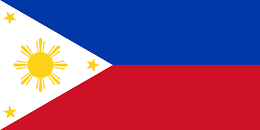
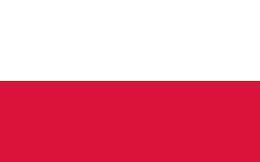
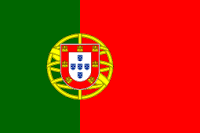
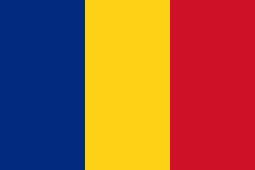
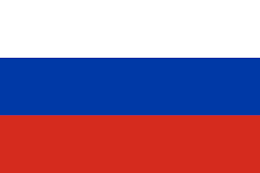

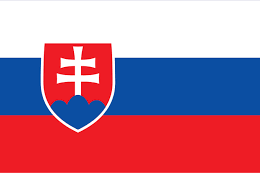
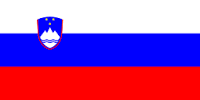
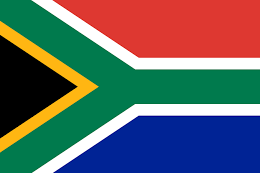
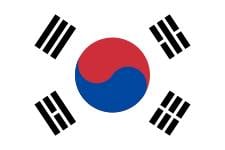
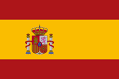
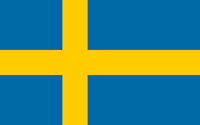
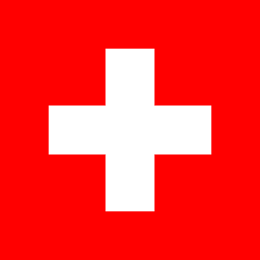
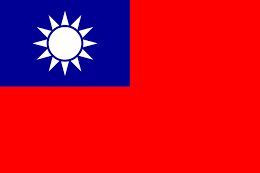
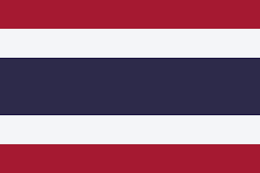
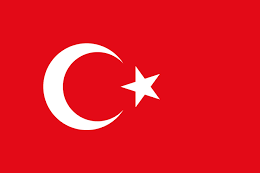
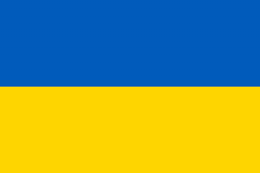
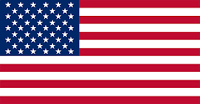