Constraint-based causal discovery
In this section, we’ll introduce the first of the four families of causal discovery methods – constraint-based methods. We will learn the core principles behind constraint-based causal discovery and implement the PC algorithm (Sprites et al., 2000).
By the end of this chapter, you will have a solid understanding of how constraint-based methods work and you’ll know how to implement the PC algorithm in practice using gCastle.
Constraints and independence
Constraint-based methods (also known as independence-based methods) aim at decoding causal structure from the data by leveraging the independence structure between three basic graphical structures: chains, forks, and colliders.
Let’s start with a brief refresher on chains, forks, and colliders. Figure 13.4 presents the three structures:
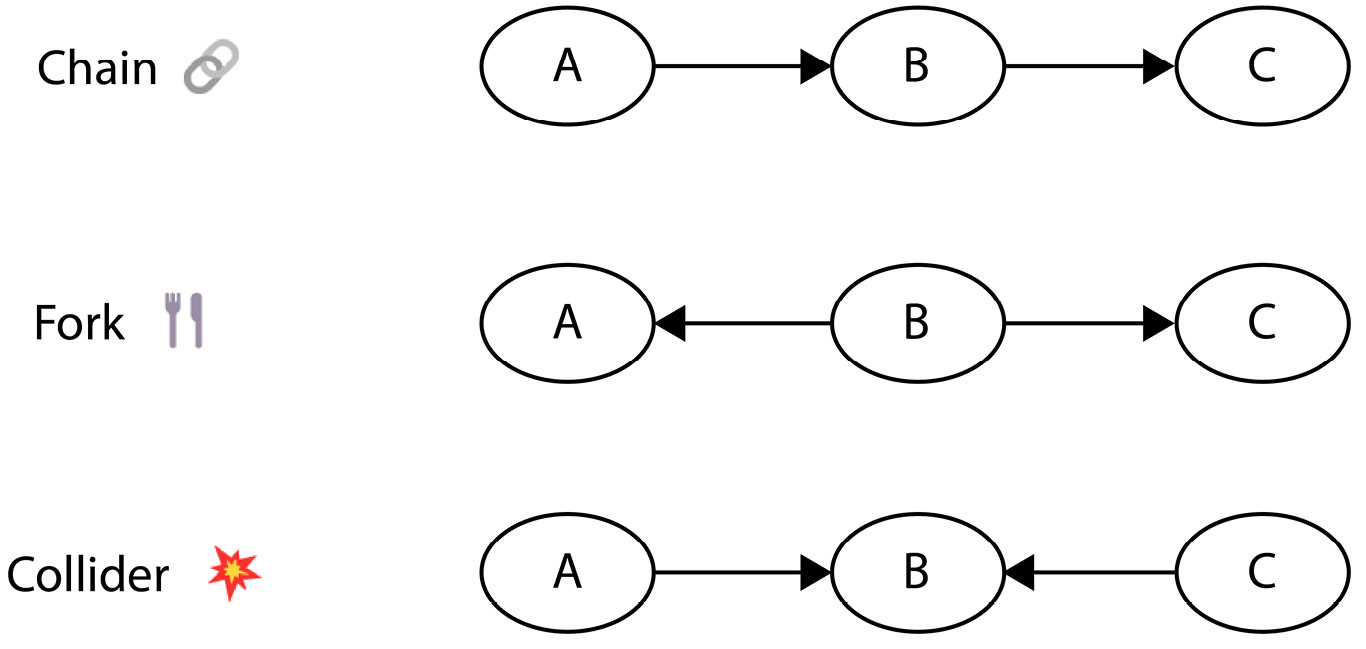
Figure 13.4 – The three basic graphical structures
In Chapter 5, we demonstrated that the...