The single most important factor in machine learning and IoT is data collection design. If the data collected is garbage data, then no machine learning can be done on top of it. Suppose you are looking at vibrations of a pump (shown in the following graph) to determine whether the pump is having issues with its mechanics or ball bearings so that preventive maintenance can be performed before serious damage is done to the machine:
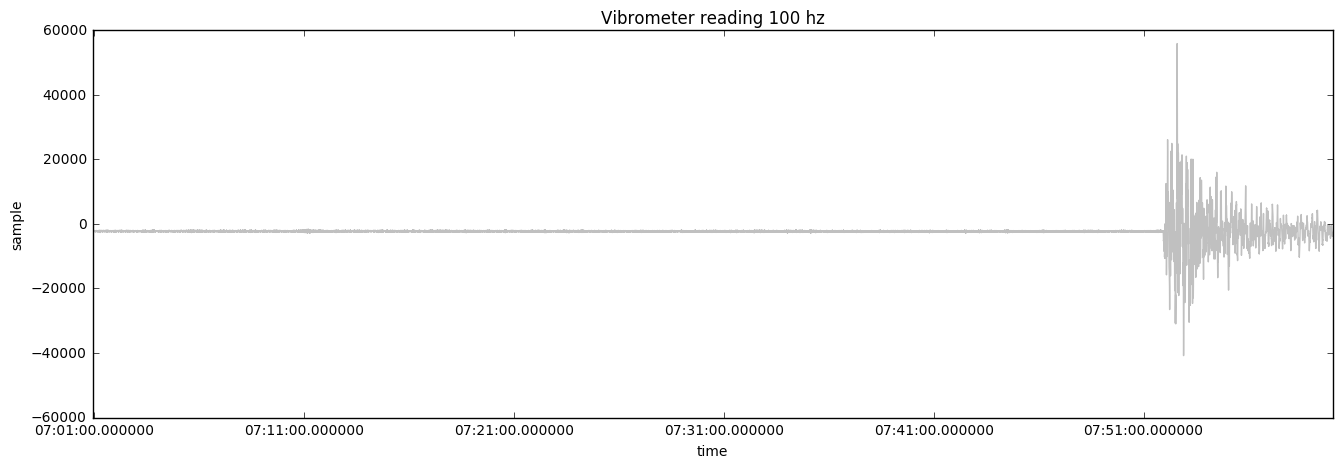
Importantly, real-time data at 100 Hz is prohibitively expensive to store in the cloud. To keep costs down, engineers often send data at frequencies of 1 minute. Low-frequency sensor data often cannot accurately represent the issue that is being looked at. The next chart shows how the data looks when only sampled once per minute:
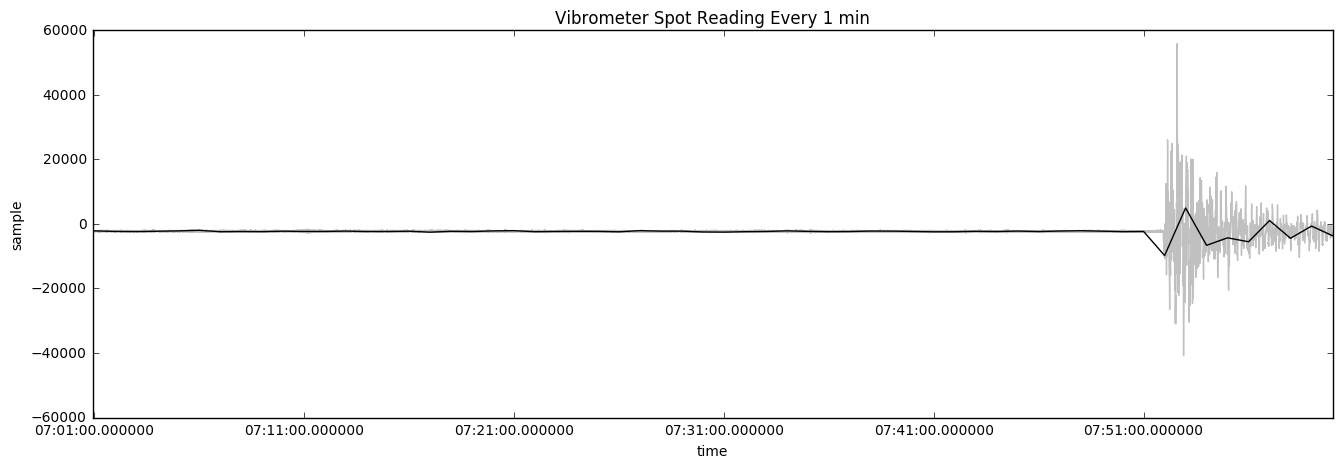
Here, we see vibrometer data overlaid with the data that is being collected in 1-minute intervals. The data has some use but it is not accurate as it does not show the true magnitude of what is going on with the data. Using the mean is worse. The following chart shows the average reading of the vibrometer's mean over 1 minute:
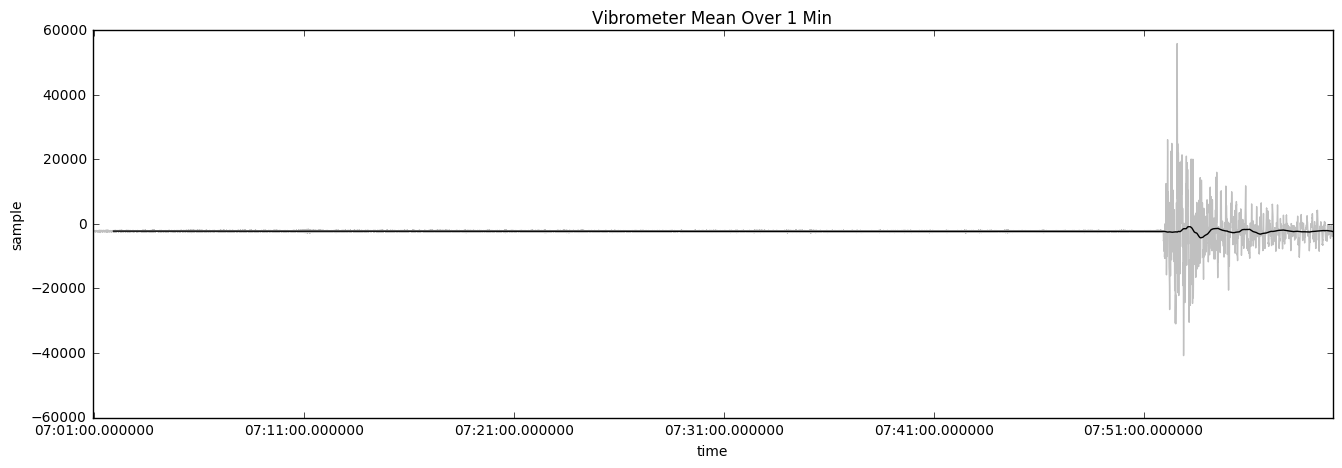
Taking the average reading windowed over 1 minute is an even worse solution because the average value is not changing when there is a problem with the pump. The following chart shows the vibrometer's standard reading over 1 minute:
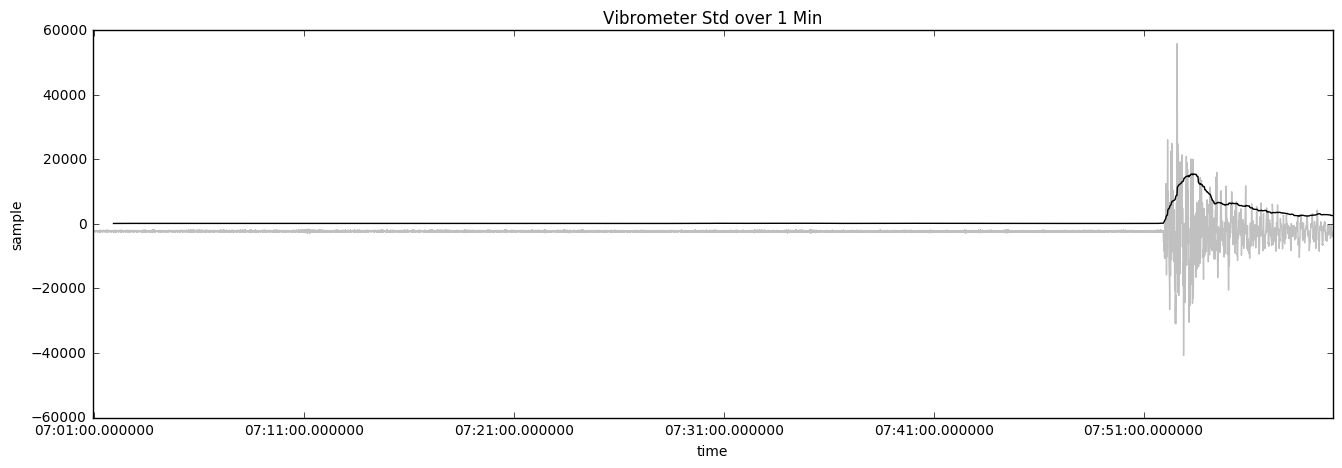
Using a standard deviation technique shows variance compared to the mean to determine whether there is an issue with the pump. This is a more accurate solution over the average technique.
Using minimum and maximum windowed over a 1-minute window can present the best representation of the magnitude of the situation. The following chart shows what the reading will look like:
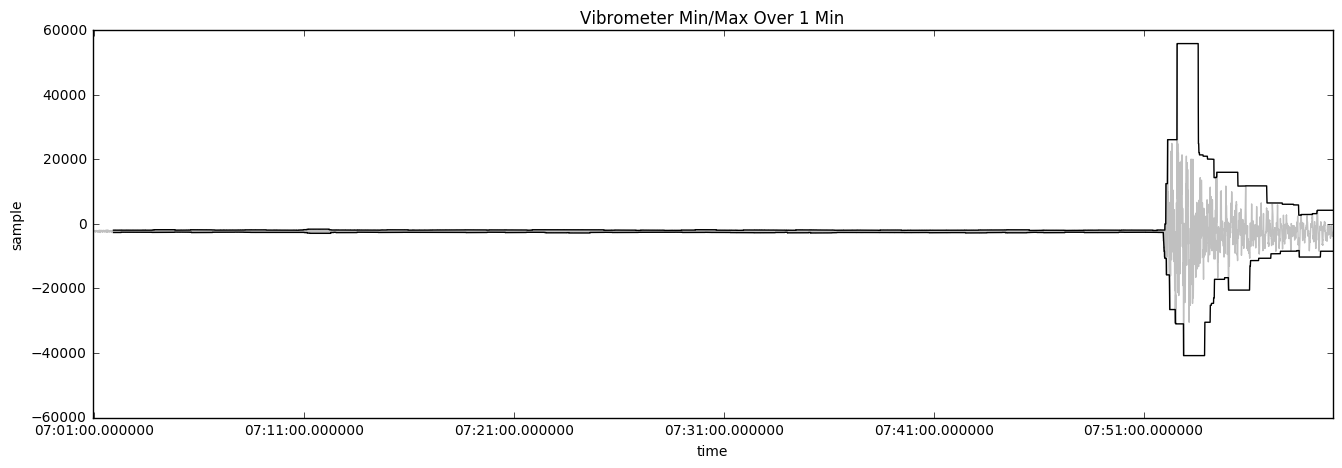
Because IoT machines can work correctly for years before having issues and forwarding high-frequency data in the cloud is cost-prohibitive, other measurements are used to determine whether the device needs maintenance. Techniques such as min/max, standard deviation, or spikes can be used to trigger a cloud-to-device message telling the device to send data at a much higher frequency. High-frequency diagnostic data can use blob storage to store large files.
One of the challenges of IoT is finding meaningful data in a sea of data. In this recipe, we shall demonstrate techniques to mine for valuable data.