Defining Gradient Descent
The purpose of training a neural networkneural network model is to find the right values for weights. We start training a neuralneural network with random or default values for the weights. Then, we iteratively use an optimizer algorithm, such as gradient descent, to change the weights in such a way that our predictions improve.The starting point of a gradient descent algorithm is the random values of weights that need to be optimized as we iterate through the algorithm. In each of the subsequent iterations, the algorithm proceeds by changing the values of the weights in such a way that the cost is minimized.The following diagram explains the logic of the gradient descent algorithm:
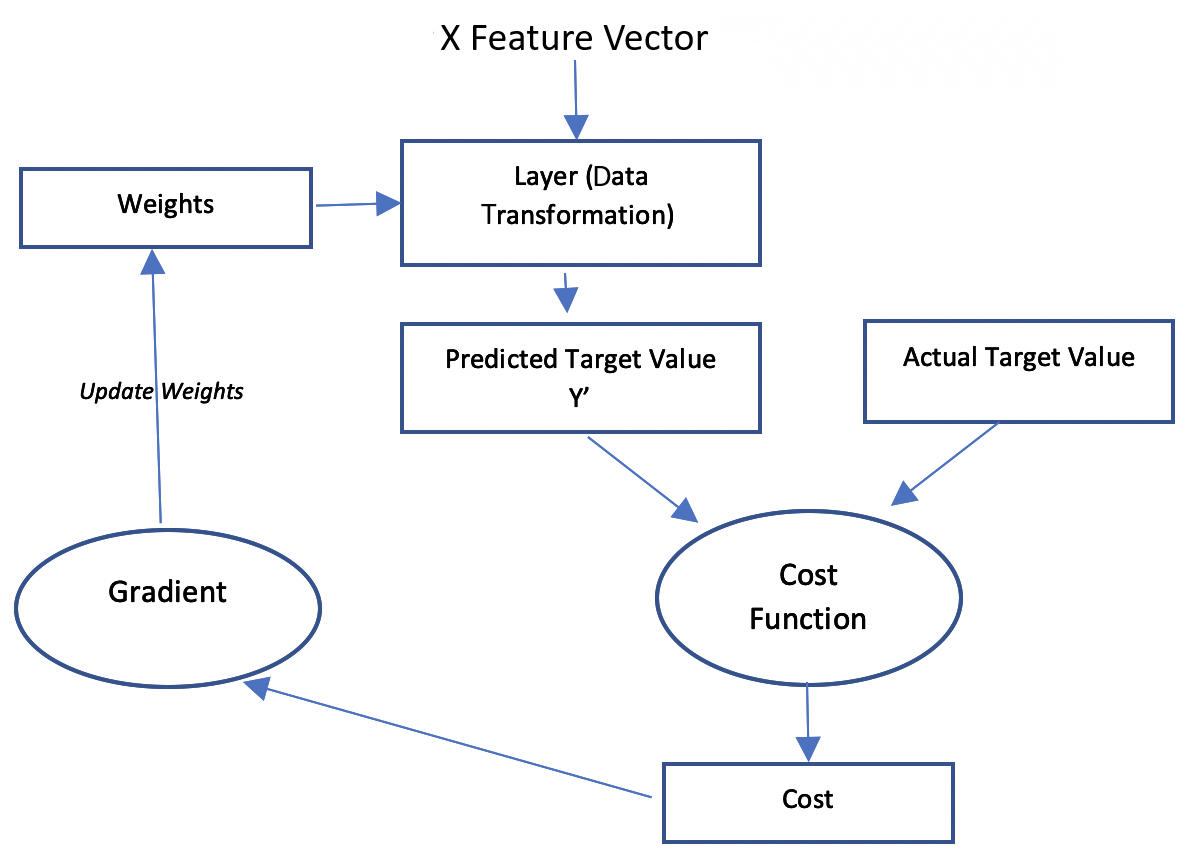
In the preceding diagram, the input is the feature vector X. The actual value of the target variable is Y and the predicted value of the target variable is Y’. We determine the deviation of the actual value from the...