Configuring Power BI Desktop to work with Python
Since you have everything you need installed, you can now configure Power BI Desktop to interact with Python engines and IDEs. This is really a very simple task:
- In Power BI Desktop, go to the File menu, click on the Options and settings tab, and then click on Options.
- In the Options window, click on the Python scripting link on the left. The contents of the panel on the right will update, giving you the option to select the Python environment to reference and the Python IDE to use for Python visuals. In order to select a specific environment, you need to choose Other and then click Browse and supply a reference to your environment folder:
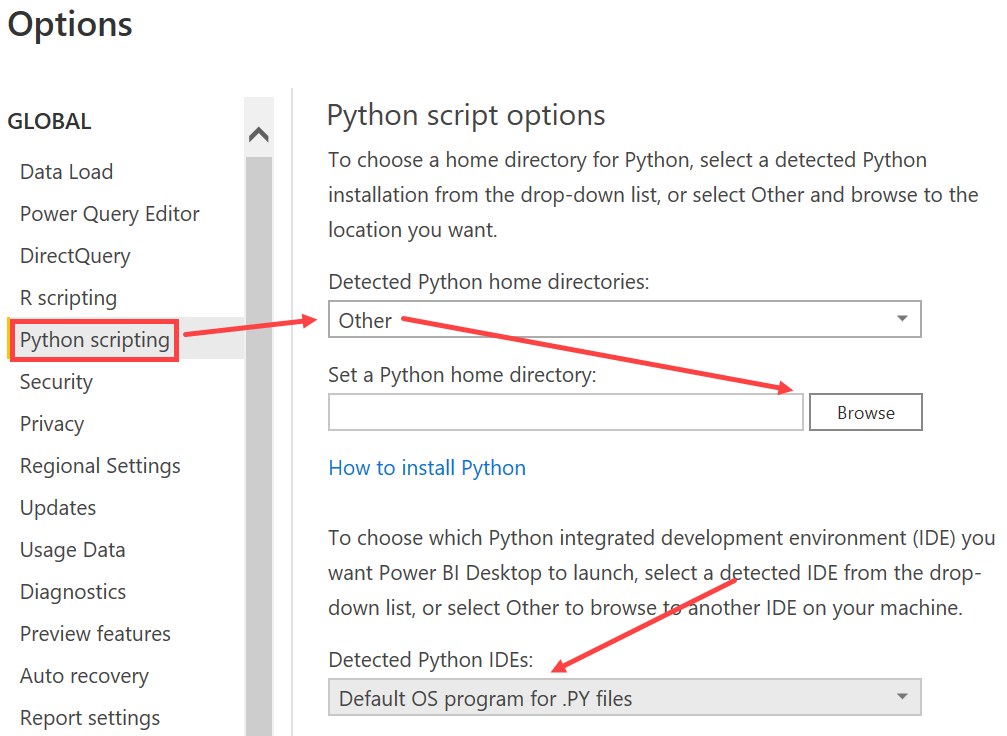
Usually, you can find the default environments folder in C:\ProgramData\Miniconda3\envs\
(all user installation) or C:\Users\<username>\miniconda3\envs\
(just your user installation). If you cannot see the hidden ProgramData...