An overview of machine learning
In this section, we will present a brief overview of ML principles and techniques. The traditional computing paradigm defines an algorithm as having three elements: the input, an output, and a process that specifies how to derive the output from the input. For example, in a credit card detection system, a module to flag suspicious transactions may have transaction metadata (location, amount, type) as input and the flag (suspicious or not) as output. The process will define the rule to set the flag based on the input, as shown in Figure 1.2:
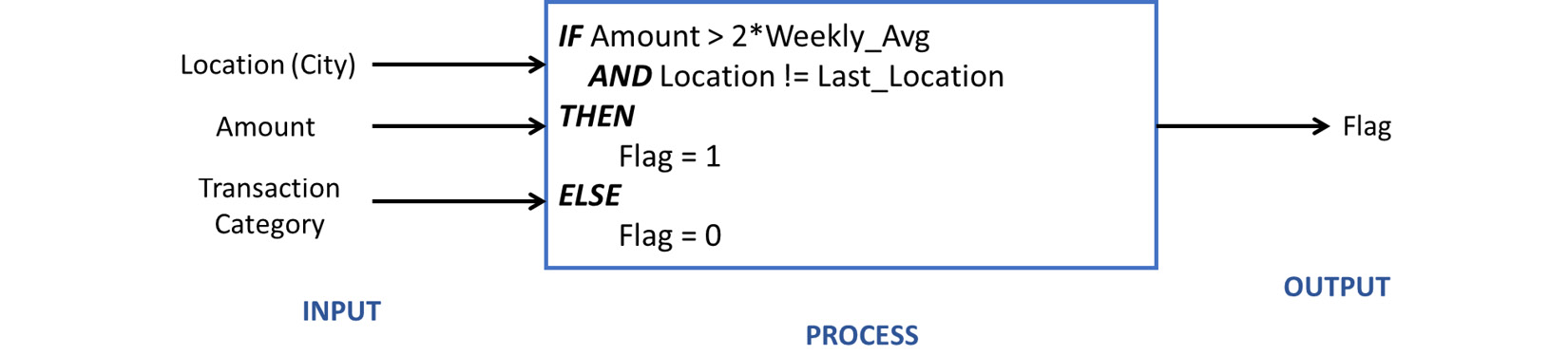
Figure 1.2 – Traditional input-process-output model for fraud detection
ML is a drastic change to the input-process-output philosophy. The traditional approach defined computing as deriving the output by applying the process to the input. In ML, we are given the input and output, and the task is to derive the process that connects the two.
Continuing our analogy of the credit...